Predicting Adolescent Female Stress with Wearable Device Data Using Machine and Deep Learning
2023 IEEE 19th International Conference on Body Sensor Networks (BSN)(2023)
摘要
The prevalence of mental health issues in adolescent females has become a significant concern in recent years. To investigate the potential of wearable biosensors in predicting stress responses in this understudied demographic, we collected wearables data from eight teenage girls over 1-4 months and explored stress prediction using several machine learning (ML) and deep learning (DL) models. Various person-dependent and person-independent prediction schemes, feature extraction methods, and classifier types were systematically investigated to provide recommendations for effective stress prediction. Feature importance for the physiological signals was also analyzed to provide insights into adolescent stress responses. The study provides actionable recommendations for classifiers, feature extraction, and personalization schemes to enhance stress prediction accuracy, enhancing the understanding and early detection of mental health issues in adolescent females.
更多查看译文
关键词
wearable devices,machine learning,deep learning,adolescent mental health,stress prediction
AI 理解论文
溯源树
样例
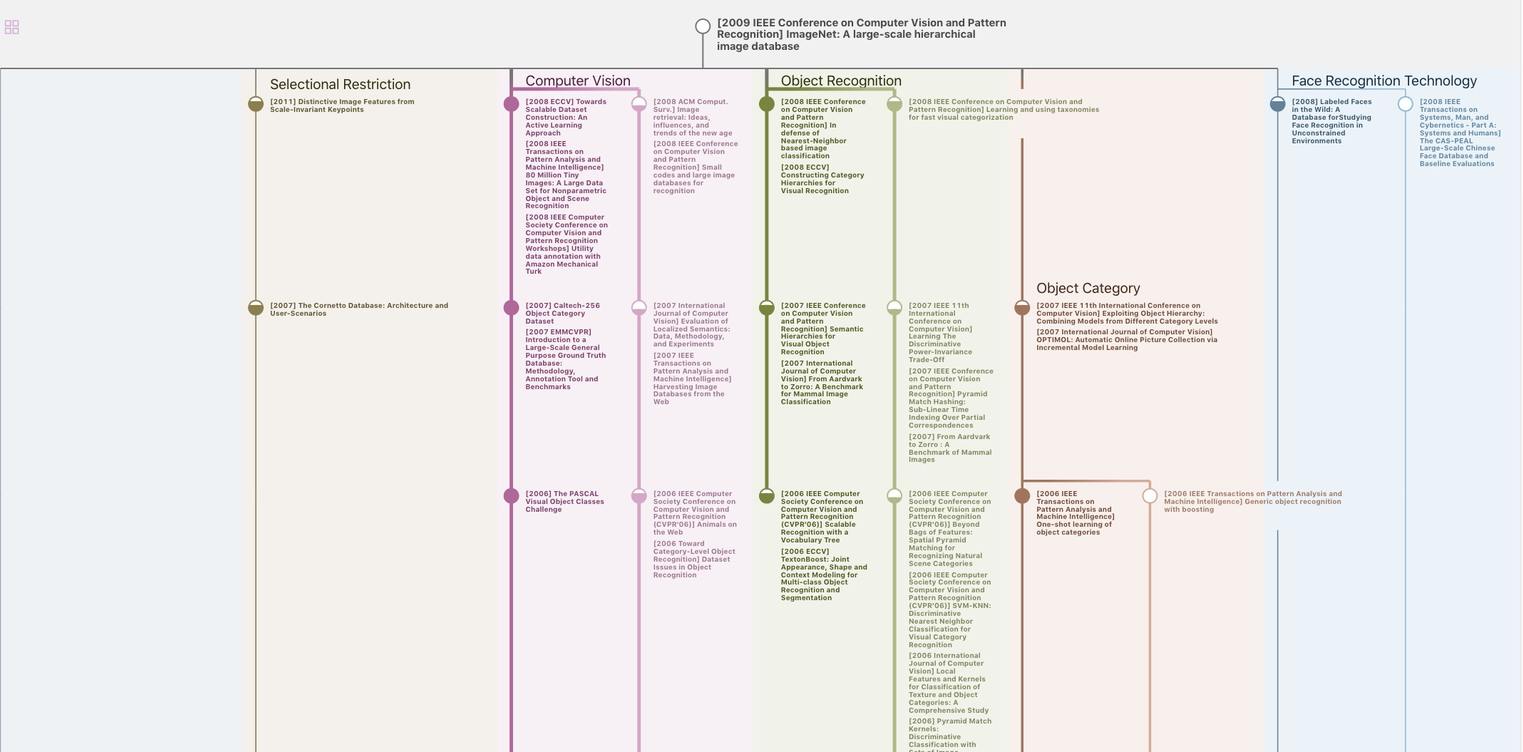
生成溯源树,研究论文发展脉络
Chat Paper
正在生成论文摘要