BoxNet: Fine-grained Action Classification in Boxing
2023 35TH CHINESE CONTROL AND DECISION CONFERENCE, CCDC(2023)
摘要
The skeleton data is widely used in general action recognition since it is robustly distinguish the action operator and complex background. When it comes to more fine-grained scenario like those in sports competitions, such characteristic of skeleton data could further enlarge differences of actions among varied classes and reduce negative effects from background to achieve better recognition result. However, the problem of coarse video record which severely deteriorate the validity of neural network is still to be solved. In this work, we present an improved graph convolutional network(GCN) methods of fine-grained technical action classification based on previous works, where early fusion and parameterized adjacency matrix are also adopted. An interpolation method is firstly applied in action classification to elevate the performance of networks with coarse input. Moreover, a new dataset about boxing established for fine-grained action classification task. Our model is verified on NTU-RGB+D and tested on established boxing dataset, and exceeds common GCN methods.
更多查看译文
关键词
Graph convolution,fine-grained action classification,skeleton dataset
AI 理解论文
溯源树
样例
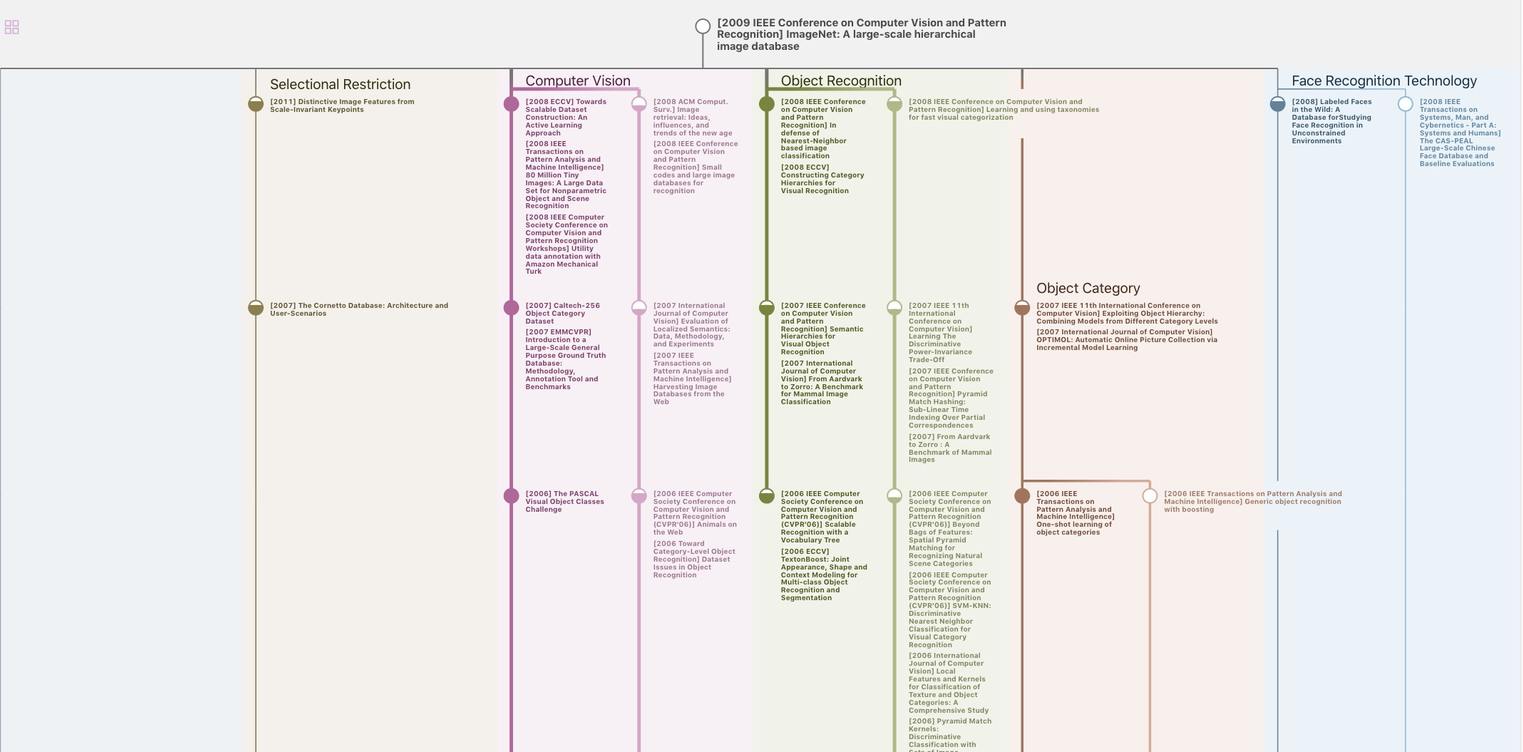
生成溯源树,研究论文发展脉络
Chat Paper
正在生成论文摘要