Load Balancing and Energy Saving Algorithm Based on Deep Q-Learning in Mobile Edge Computing
2023 35TH CHINESE CONTROL AND DECISION CONFERENCE, CCDC(2023)
摘要
Mobile Edge Computing (MEC) offloads computationally intensive tasks to edge servers, enabling resource-limited mobile devices to supply higher-quality computing services. However, the access to large-scale mobile devices puts forward higher requirements on the allocation of MEC computing and communication resources. In order to rationally allocate system resources, this paper conducts an in-depth study on the joint optimization problem of task scheduling, offloading decisions and resource allocation under the MEC server system consisting of multiple mobile devices (MDs) and multiple edge servers. The task deployment and resource allocation, etc. are accomplished under the conditions of satisfying the constraints of CPU frequency, transmission power, computing resources and latency to achieve the weighted sum minimization of energy consumption and server workload. This paper presents a Deep Q-Learning (DQN) enabled task deployment and resource allocation (DQLB) algorithm for mobile edge computing task offloading. Firstly, the action space for joint task offloading and task deployment is constructed. Secondly, pruning optimization is performed on the action space to remove the redundant action space and reduce the magnitude of the action space. Finally, the optimized action space is trained with a model using neural networks to obtain suboptimal task deployment, task offloading and resource allocation strategies. The simulation results show that the algorithm can achieve convergence in a small number of iterations, and it has achieved remarkable results in reducing balancing energy consumption, balancing server workload, and shortening task execution time.
更多查看译文
关键词
Edge Computing,Computation Offloading,Load Balancing,Task Deployment,Deep Q-Learning
AI 理解论文
溯源树
样例
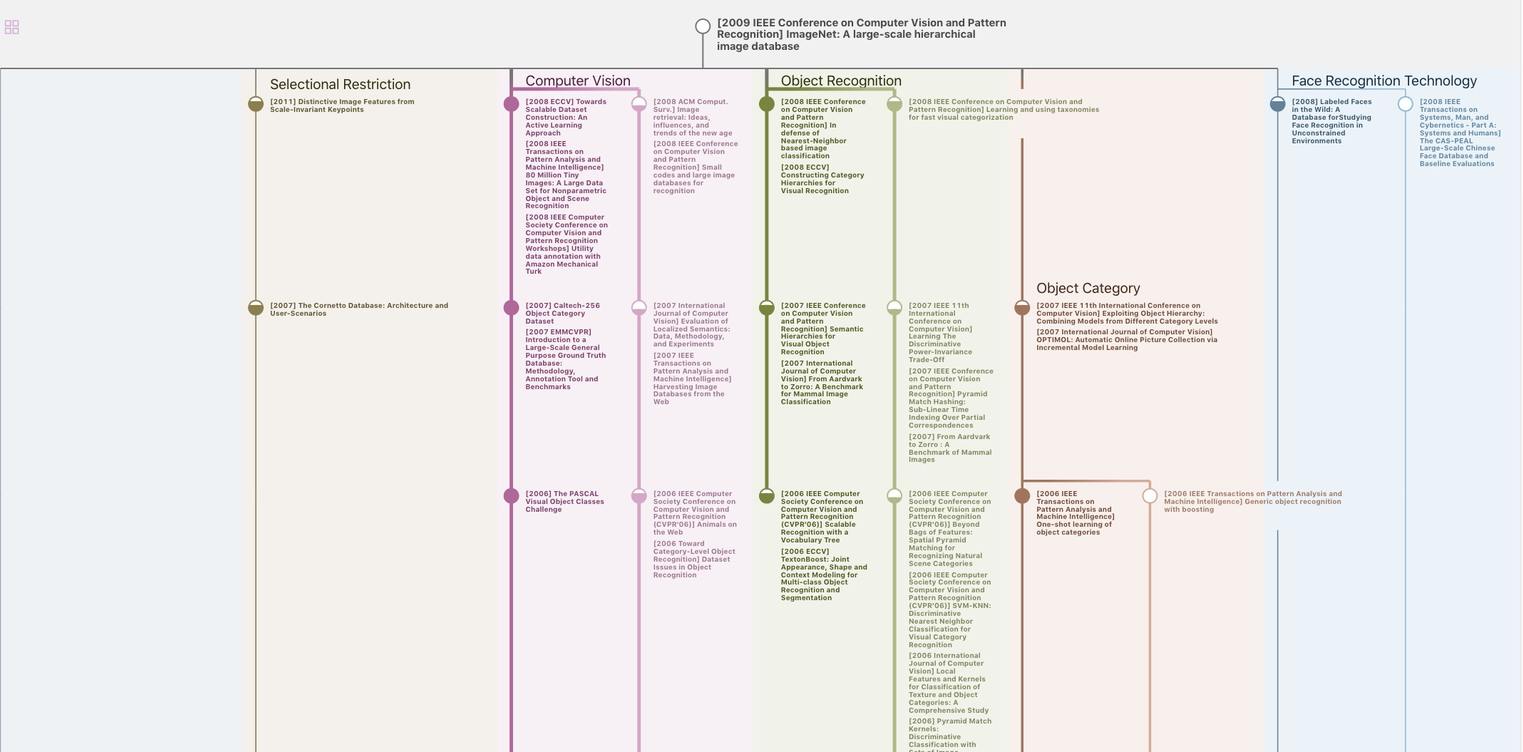
生成溯源树,研究论文发展脉络
Chat Paper
正在生成论文摘要