Mapping surface water dynamics (1985-2021) in the Hudson Bay Lowlands, Canada using sub-pixel Landsat analysis
REMOTE SENSING OF ENVIRONMENT(2024)
摘要
The fate of the carbon stored in frozen peatlands in Canada's Hudson Bay Lowlands (HBL) depends strongly on water, with wetter conditions favoring long-term storage. Dynamic surface water products generated from historical Landsat data exist to inform surface water trends in the HBL based on binary classifications of land vs water at 30 m spatial resolution. However, the HBL contains many water features smaller than 30 m, including streams and patterned fens that require a sub-pixel mapping approach to fully capture their dynamics. In this paper, we leverage an existing binary dynamic surface water product to generate a spatially and temporally comprehensive Landsat sub-pixel surface water time-series over the HBL. We first generate Landsat Red, NIR and SWIR composites from 1985 to 2021 and evaluate data-driven machine learning and physical models to derive sub-30 m water fractions across the entire mapping domain. Data-driven models were calibrated on 30 m water fractions obtained from 16 of 17 WorldView scene water fraction maps and assessed on the 17th held-out scene in a jackknife validation. Data-driven models were also calibrated by relating scaled Landsat reflectance to water fractions obtained from binary water masks at corresponding scales from 90 to 270 m. Physical models involved simple linear unmixing using a new approach that samples land endmembers in the vicinity of each pixel to represent land reflectance, and a global water endmember sampled beneath permanent water in each image. The assumption of linear scaling was verified at sub-30 m to 270 m resolution for NIR, SWIR and NDVI, which were all highly correlated with water fraction and had no significant differences among water fraction - spectral feature relationships across the full range of scales. Each of 11 different methods and input spectral features were evaluated against WorldView water fractions and ranked based on nine criteria. We found that machine learning using all spectral features including local NIR land endmembers performed best among data-driven approaches, and linear unmixing of the NIR band using local land endmembers performed best among physical. Both best performing methods were applied to the entire time-series and surface water area trends were calculated and compared with trends obtained from binary water masks. All three methods agree on the direction of surface water change towards wetting, with sub-pixel inter-annual variation agreeing with stream discharge noted in other studies.
更多查看译文
关键词
Unmixing,Surface water,Peatland,Climate change,Landsat,Time-series
AI 理解论文
溯源树
样例
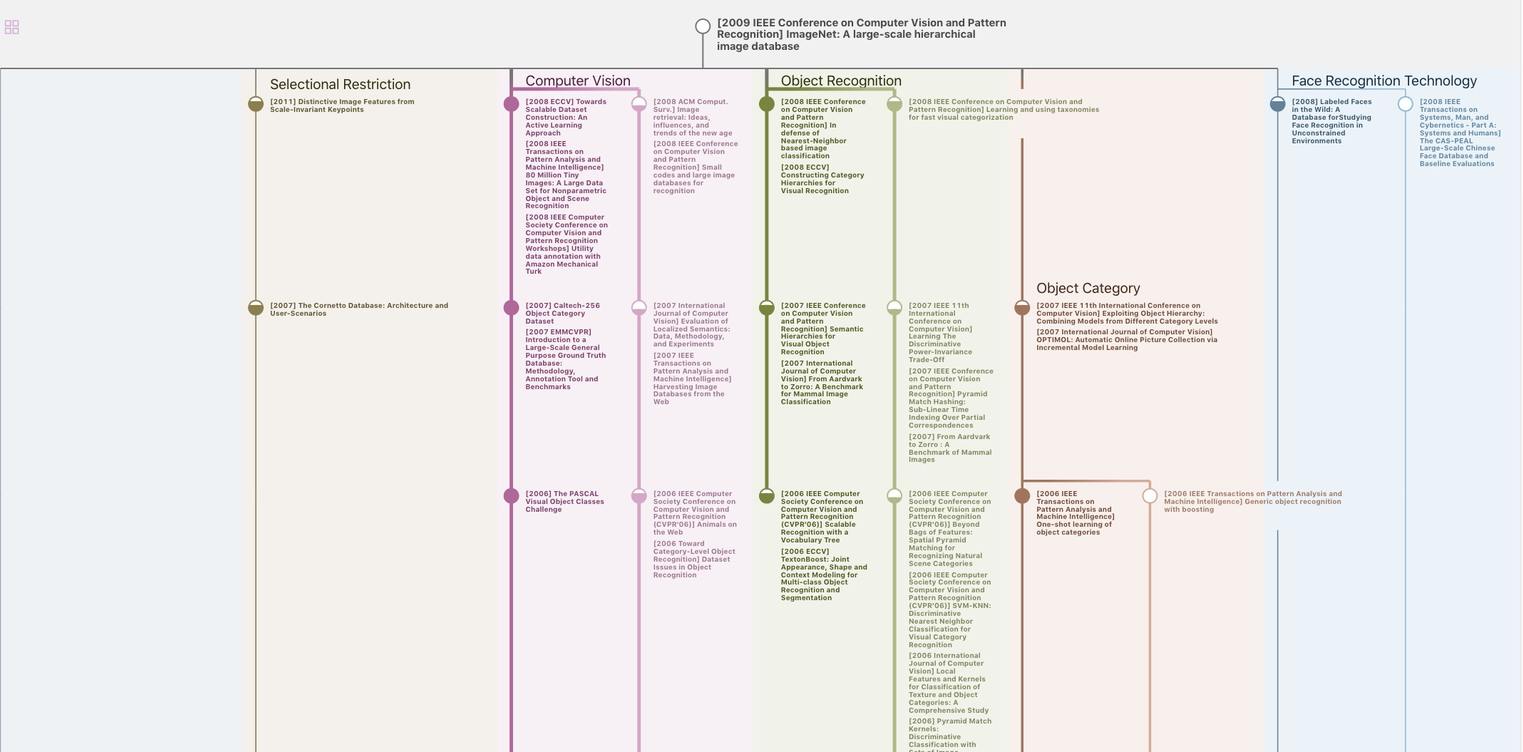
生成溯源树,研究论文发展脉络
Chat Paper
正在生成论文摘要