Deep-learning based triple-stage framework for MRI-CT cross-modality gross tumor volume (GTV) segmentation for rectal cancer neoadjuvant radiotherapy
BIOMEDICAL SIGNAL PROCESSING AND CONTROL(2024)
摘要
Background & objective: Delineation of the gross tumor volume (GTV) for rectal cancer is pivotal for successful radiotherapy (RT) treatment, but the procedure is cross-modality, therefore labor-intensive and error-prone. This was the first study to address this challenge with a novel deep-learning (DL) based triple-stage framework for fast and automated GTV segmentation. Methods & materials: The proposed DL-based triple-stage framework integrated intemediate GTV-MRI (Stage 1) and GTV-CT segmentation (Stage 2) respectively, and specialized post-processing with MRI-CT mapping and a pretrained rectal semgnetaion model to make necessary revisions (Stage 3). We employed a dual-path-network Unet (DpnUnet) architecture as the kernel backbone and devised a hybrid loss function that combined binary cross-entropy and Dice losses. We retrospectively enrolled 88 Stage II/III rectal cancer patients recieving neoadjuvant RT, of which 70 were for training and 18 for external testing. The model performance was evaluated using the Dice similarity coefficient (DSC), 95th percentile of the Hausdorff distance (95HD), sensitivity, and specificity over the 18 external cases. The Wilcoxon paired signed-rank test was used to statistically compare the proposed method with ablated methods as alternatives. Results: The proposed framework significantly outperformed alternatives (p < 0.05) with 0.876 +/- 0.076 in DSC, 5.790 +/- 3.001 mm in 95HD, 0.871 +/- 0.121 in sensitivity, and 0.953 +/- 0.035 in specificity. Compared with methods and results in the literature, our approach exhibited highly superior accuracy. The overall predication time per testing case was less than 40 s. Conclusion: The proposed DL-based triple-stage framework represents a leap forwards automated GTV segmentation in rectal cancer neoadjuvant RT with promising accuracy and efficiency.
更多查看译文
关键词
Radiotherapy,Rectal cancer,Gross tumor volume (GTV),Deep learning,Cross modality,Computed tomography (CT),Magnetic resonance imaging (MRI)
AI 理解论文
溯源树
样例
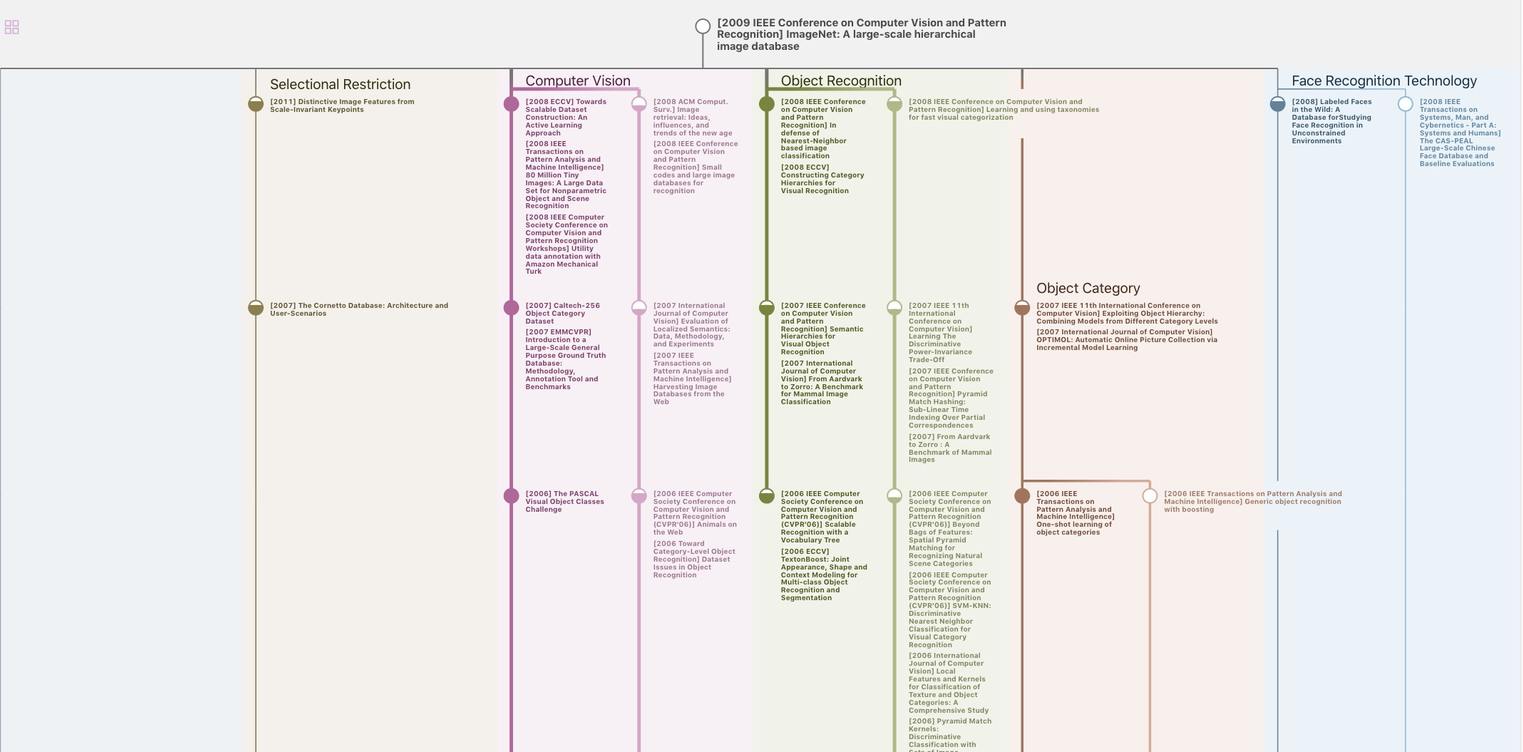
生成溯源树,研究论文发展脉络
Chat Paper
正在生成论文摘要