A novel dual-stage progressive enhancement network for single image deraining
ENGINEERING APPLICATIONS OF ARTIFICIAL INTELLIGENCE(2024)
摘要
The dense rain accumulation in heavy rain can significantly wash out images and thus destroy the background details of images. Although existing deep rain removal models lead to improved performance for heavy rain removal, we find that most of them ignore the detail reconstruction accuracy of rain-free images. In this paper, we propose a dual-stage progressive enhancement network (DPENet-v2) to achieve effective deraining with structure-accurate rain-free images. Three main modules are included in our framework, namely a rain streaks removal network (R2Net), a details reconstruction network (DRNet) and a cross-stage feature interaction module (CFIM). The former aims to achieve accurate rain removal, and the latter is designed to recover the details of rain-free images. We introduce two main strategies within our networks to achieve trade-off between the effectiveness of deraining and the detail restoration of rain-free images. Firstly, a dilated dense residual block (DDRB) within the rain streaks removal network is presented to aggregate high/low level features of heavy rain. Secondly, an enhanced residual pixel-wise attention block (ERPAB) within the details reconstruction network is designed for context information aggregation. Meanwhile, CFIM learns the long-range dependencies and achieves cross-stage information communication. We also propose a comprehensive loss function to highlight the marginal and regional accuracy of rain-free images. Extensive experiments on benchmark public datasets show both efficiency and effectiveness of the proposed method in achieving structure-preserving rain-free images for heavy rain removal.
更多查看译文
关键词
Image deraining,Detail restoration,Efficient network,Dual-stage leaning,Context aggregation
AI 理解论文
溯源树
样例
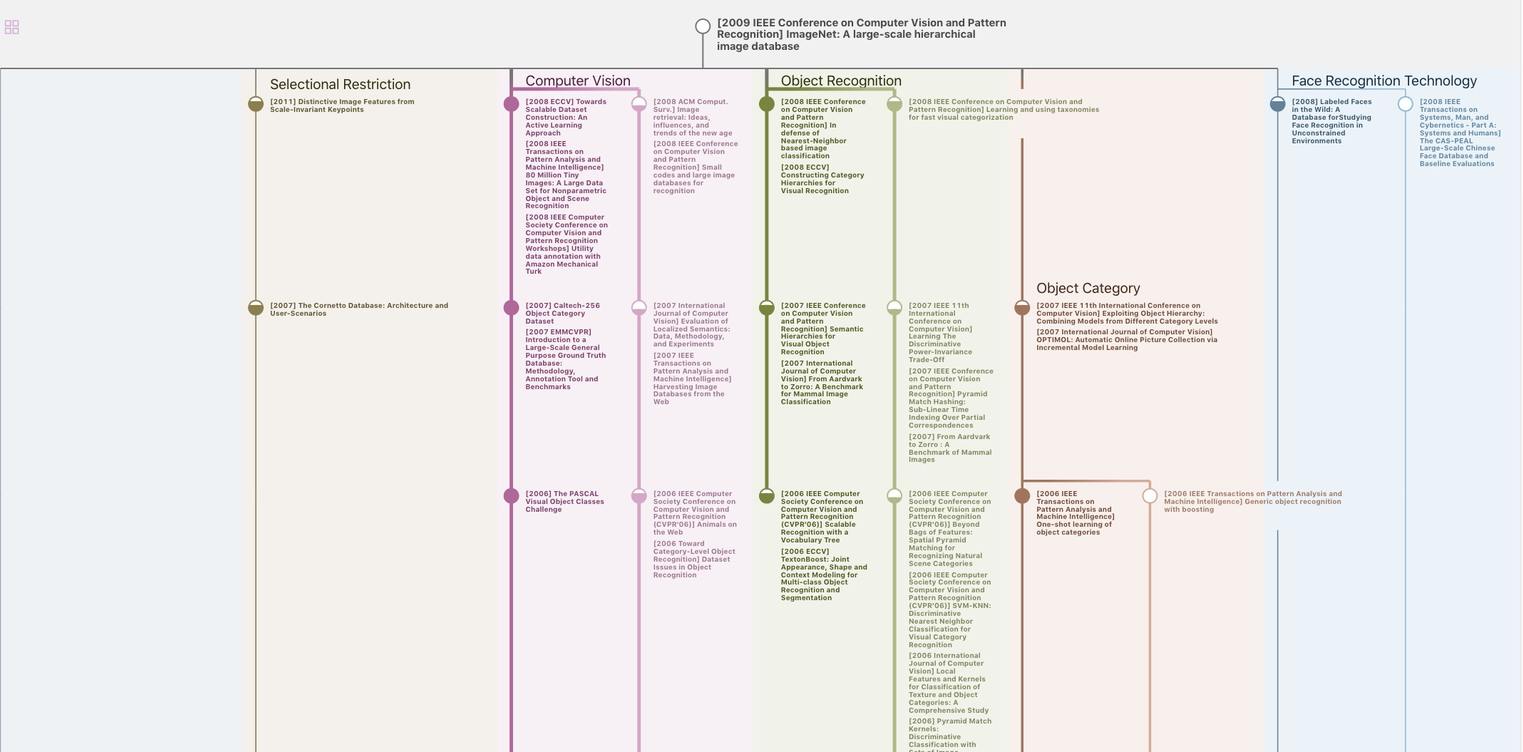
生成溯源树,研究论文发展脉络
Chat Paper
正在生成论文摘要