DRL based low carbon economic dispatch by considering power transmission safety limitations in internet of energy
INTERNET OF THINGS(2023)
摘要
Economic dispatch, as a crucial method for ensuring the normal operation of power systems, is typically modeled as an optimization problem and solved using solvers. The introduction of low-carbon requirements has increased the complexity of the optimization problem, as economic dispatch now needs to consider effectively reducing the emission of CO2. The utilization of renewable energy can mitigate carbon emissions during power system operation, but its inherent uncertainty poses safety risks to transmission lines between microgrids and the main grid. Hence, this paper explores the issue of low-carbon economic dispatch to ensure the reliability of power transmission. We introduce a two-stage low-carbon economic dispatch model grounded in deep reinforcement learning. In the first stage, we incorporate the transmission power limits between microgrids and the main grid as constraints, generate day-ahead dispatch strategies, and determine the weights of factors in the reward function. In the second stage, we employ two methods: adjusting the weight of the penalty for exceeding the transmission limits and adding conservative safety limit constraints, to solve the problem of transmission power exceeding limits caused by uncertainties of renewable energy and prediction errors. Three deep reinforcement learning algorithms, all rooted in the Actor-Critic structure, are employed to implement the two-stage economic dispatch model. Experimental results affirm the efficacy of the proposed model in mitigating the risk of transmission power exceeding its limit, reducing carbon emissions, and minimizing operational costs.
更多查看译文
关键词
Low-carbon economic dispatch,Transmission safety,Markov decision process,Deep reinforcement learning
AI 理解论文
溯源树
样例
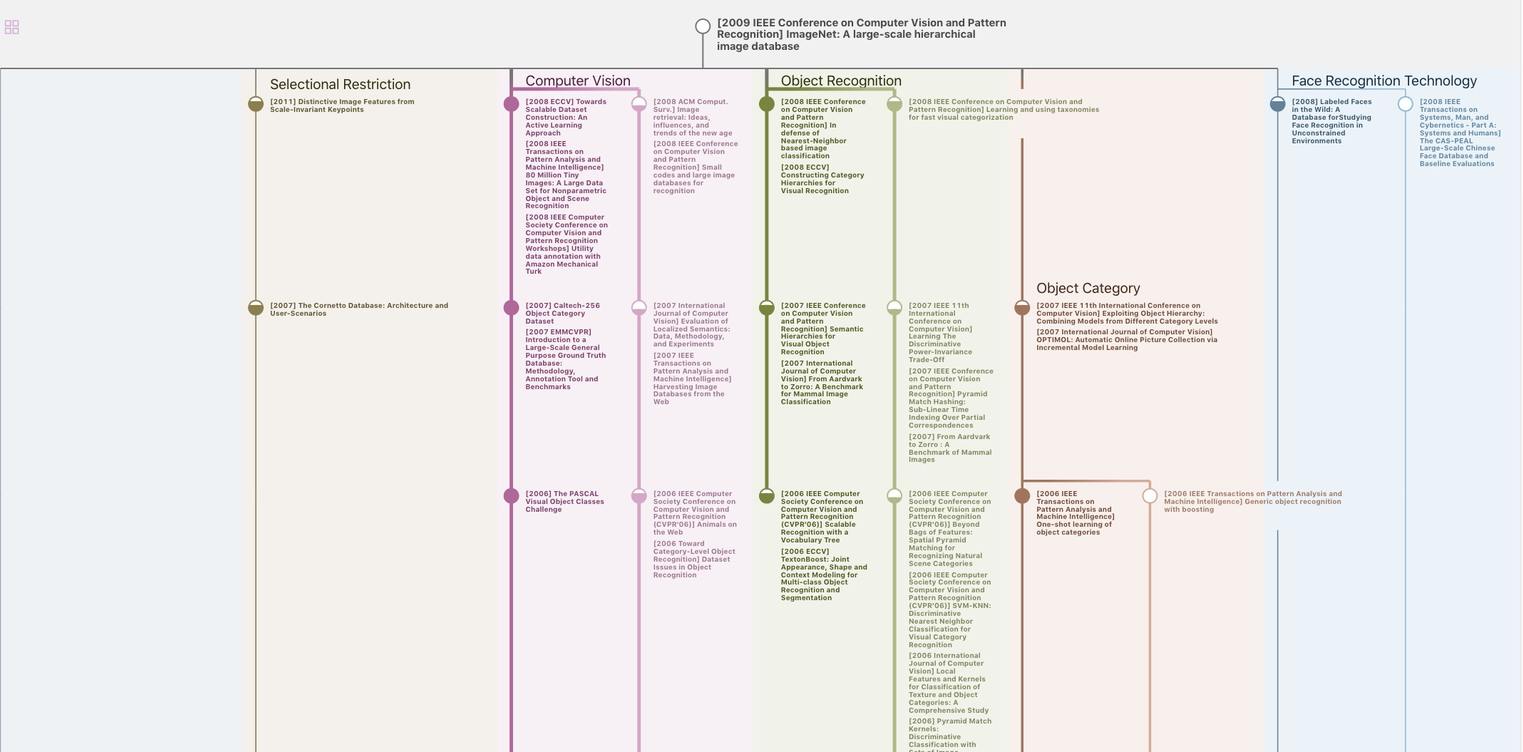
生成溯源树,研究论文发展脉络
Chat Paper
正在生成论文摘要