A two-stage denoising framework for zero-shot learning with noisy labels
INFORMATION SCIENCES(2024)
摘要
Although zero-shot learning (ZSL) has gained widespread concern due to its excellent capacity of recognizing new object classes without seeing any visual instances, most existing methods assume that all seen-class instances used for training are correctly labeled. In some real application scenarios, when it comes to noisy labels, ZSL will inevitably suffer accuracy collapse. To address the issue, a two-stage denoising framework (TSDF) is proposed for ZSL in this work. First, an ZSLoriented Joint training with co-regularization (JoCoR) is developed, which includes a tailored loss function that helps remove suspected noisy-label instances prior to training a ZSL model. Second, a ramp-style loss function is designed to reduce negative impact brought by the remaining noisy labels. In order to facilitate incorporating the ramp-style loss into deep-architecture based ZSL models, a matched dynamic screening strategy (DSS) is also developed. Unlike the traditional concave-convex procedure (CCCP) framework, DSS handles the nonconvexity of the ramp-style loss without requiring an additional iterative loop, demonstrating notable advantages in efficiency. In addition, DSS could work without a predetermined truncating point in the ramp-style loss. Experimental results show that our proposed method achieves exciting results in various noisy-label environments.
更多查看译文
关键词
Zero shot learning,Noisy labels,Instance filter,Ramp-style loss,Dynamic screening strategy
AI 理解论文
溯源树
样例
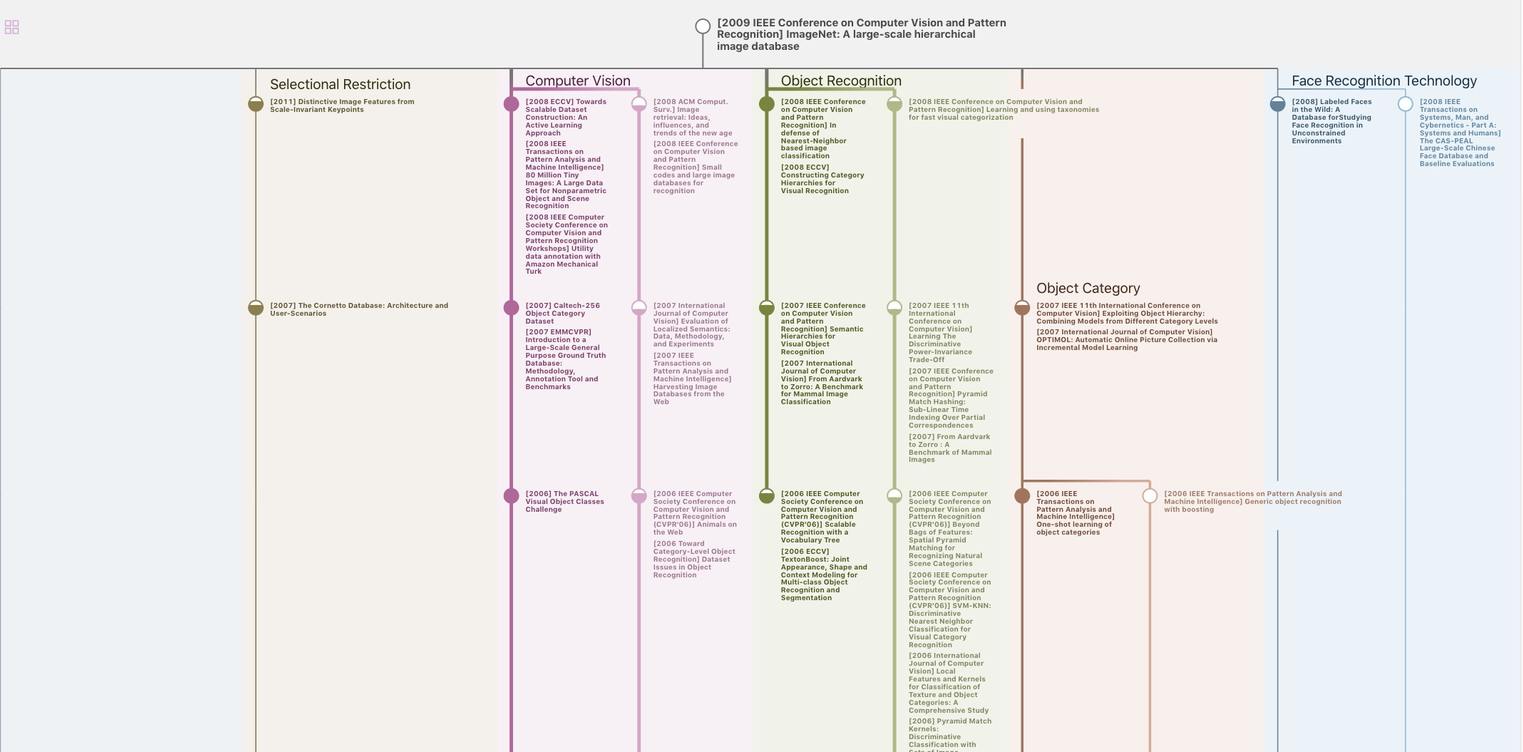
生成溯源树,研究论文发展脉络
Chat Paper
正在生成论文摘要