Cov-Fed: Federated learning-based framework for COVID-19 diagnosis using chest X-ray scans
ENGINEERING APPLICATIONS OF ARTIFICIAL INTELLIGENCE(2024)
摘要
SARS-CoV-2, a member of the coronavirus family, causes COVID-19, which can range from the ordinary cold to the rare but fatal Severe Acute Respiratory Syndrome (SARS) and Middle East Respiratory Disease (MERS). Radiologists can better diagnose COVID-19 using deep learning and computer vision techniques. However, training requires large centralized datasets. In addition, medical data protection prevents centralized server training with medical data. In this study, we propose a federated learning-based framework, Cov-Fed, to facilitate decentralized training of medical data. Cov-Fed is characterized by the proposed Multi-Efficient Channel Attention Network (MECAN) and a multi-model user-server weight update mechanism, respectively. In addition, Cov-Fed leverages PySyft encryption and decryption mechanisms for privacy preservation. Due to the salient nature of features corresponding to viruses, the classification of x-ray scans is challenging. Hence, we propose a multi-ECA attention mechanism embedded in the Cov-Fed to enhance feature maps of chest X-ray scans for classification without compromising performance. Extensive experiments are conducted on several public medical datasets and compared with some state-of-the-art models. Based on several evaluation metrics, Cov-Fed exhibited satisfactory performance with an average accuracy of 87.65%, an inference time of 92.8s, and ROC-AUC of 92.46% compared with some state-of-the-art models.
更多查看译文
关键词
Federated learning,Medical diagnosis,Deep learning,Attention mechanism
AI 理解论文
溯源树
样例
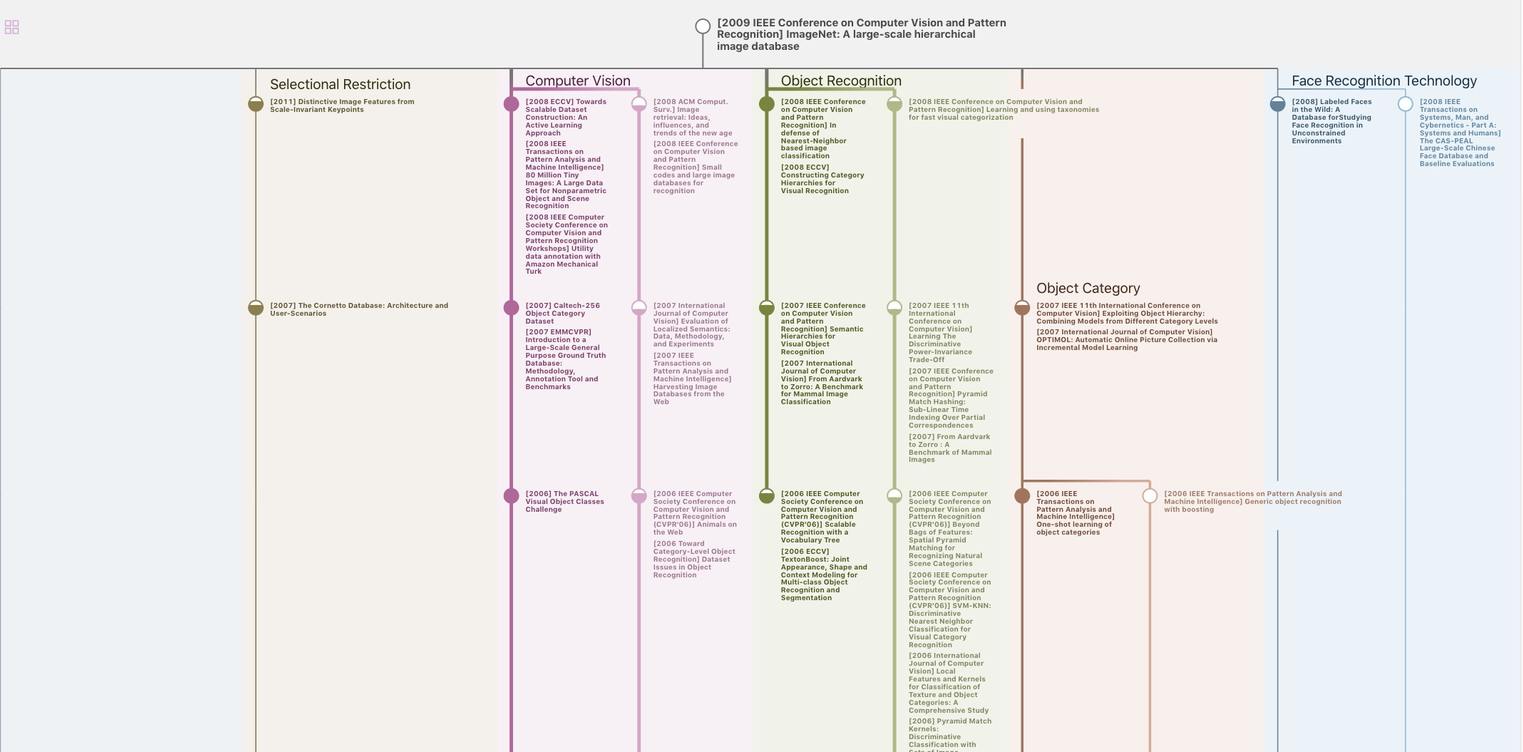
生成溯源树,研究论文发展脉络
Chat Paper
正在生成论文摘要