Multi-objective Optimization of Thermal Power and Outlet Steam Temperature for a Nuclear Steam Supply System with Deep Reinforcement Learning
ENERGY(2024)
摘要
The nuclear steam supply system (NSSS) is a critical component of a nuclear power plant that produces steam for electricity or cogeneration. However, the performance of current control strategies in the NSSS is limited since the coupling in the system is too complex and non-linear to be modeled precisely. Thus, there is an urgent need to study the model-free optimization method of NSSS. Motivated by this, this article proposes a novel multi-objective optimization approach based on deep reinforcement learning (DRL). With a hierarchical structure, the proposed method improves the response performances of both thermal power and outlet steam temperature by dynamically adjusting the reference values of existing proportional–integral–differential (PID) controllers within NSSS. This structure combines the stability of PID’s closed-loop control and the optimization capabilities of DRL for a safe and efficient operation. Moreover, a safe DRL method with an event-triggered mechanism is proposed to further ensure safety throughout the optimization process. The numerical simulations demonstrate the effectiveness and superiority of the proposed method, which results in significant improvements in the transient response compared to traditional PID controllers.
更多查看译文
关键词
Nuclear power plant,Coordinated control,Multi-objective optimization,Safe deep reinforcement learning
AI 理解论文
溯源树
样例
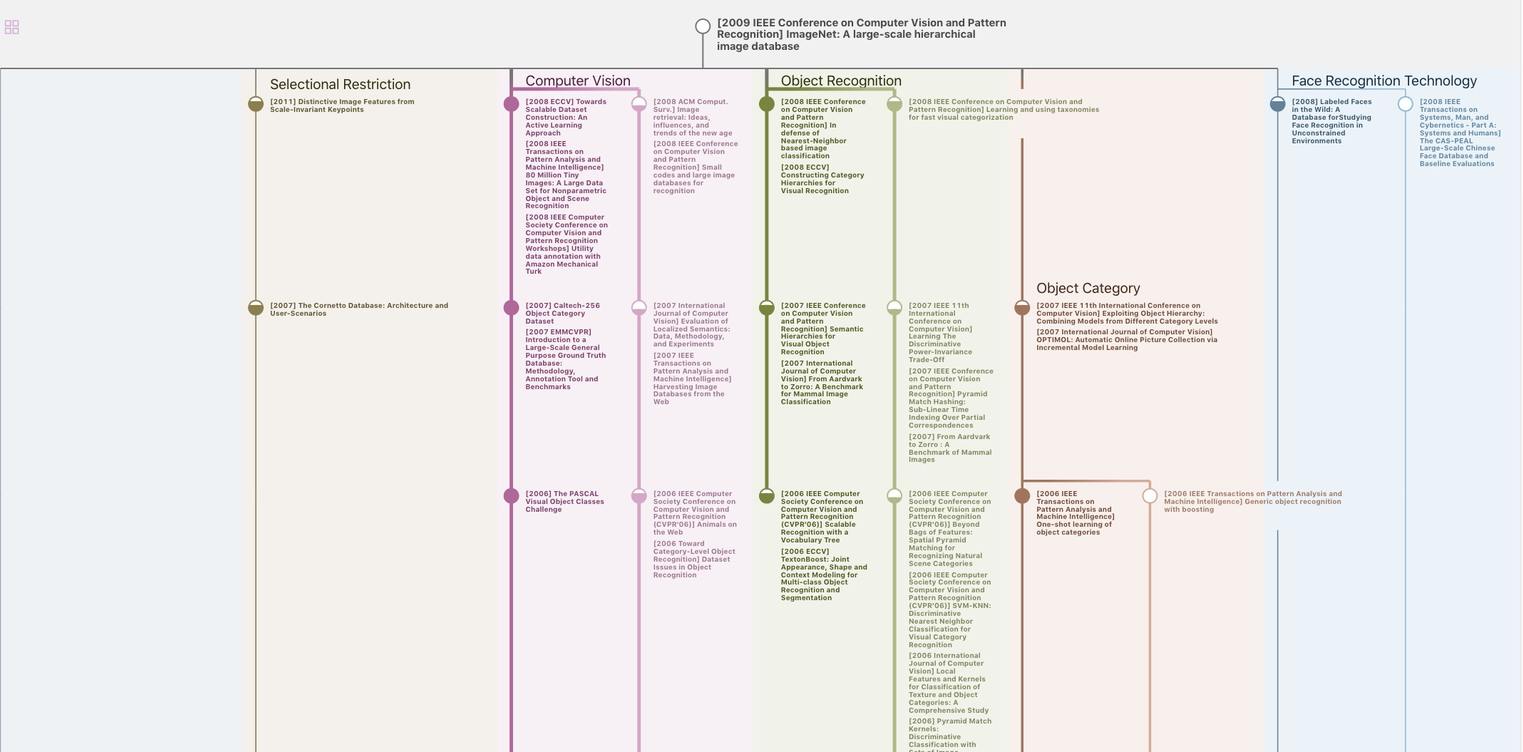
生成溯源树,研究论文发展脉络
Chat Paper
正在生成论文摘要