Learning transferable targeted universal adversarial perturbations by sequential meta-learning
COMPUTERS & SECURITY(2024)
摘要
Recently, the transferability of adversarial perturbations in non-targeted scenarios has been extensively studied. However, changing the predictions of an unknown model to a pre-defined 'targeted' class still remains challenging. In this study, we aim to learn the targeted universal adversarial perturbations (UAPs) with higher transferability by the ensemble of multiple models. First, we observe the phenomenon that the logit of the target class will bias to a specific white-box model in existing ensemble-based attacks. To deal with the issue, we propose a normalized logit loss to narrow the margin of the targeted class's logits among different models. Besides, we introduce a novel sequential meta-learning optimization strategy to further increase transferability, consisting of the inner loop and the outer loop. In the inner loop, we sequentially learn task-specific targeted UAPs for each source model by jointly considering the perturbation from the previous model. In the outer loop, we optimize the task-agnostic targeted UAP by combining the targeted UAPs from the inner loop. Experimental results demonstrate the mutual benefits of the normalized logit loss and the sequential meta-learning optimization strategy for learning targeted adversarial perturbations, outperforming existing ensemble attacks in both white box and black-box settings. The source code of this study is available at: Link.
更多查看译文
关键词
Targeted adversarial attacks,Model-agnostic meta-learning,Data-free universal adversarial perturbations,Transfer-based black-box attacks
AI 理解论文
溯源树
样例
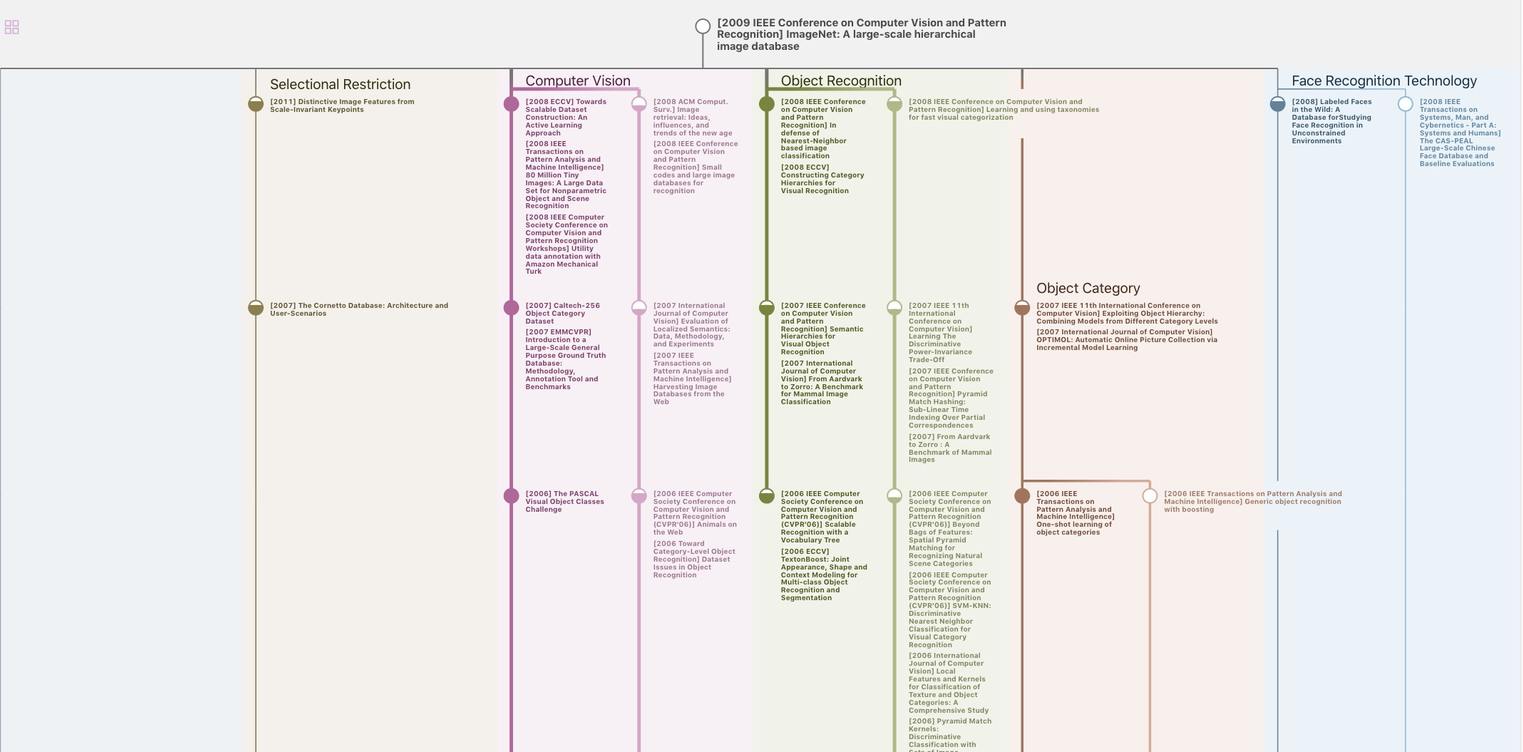
生成溯源树,研究论文发展脉络
Chat Paper
正在生成论文摘要