Physics-reserved spatiotemporal modeling of battery thermal process: Temperature prediction, parameter identification, and heat generation rate estimation
JOURNAL OF ENERGY STORAGE(2024)
摘要
The battery thermal process, as a typical distributed parameter system (DPS), is critical to battery management. In practice, the process information is often partially known. Data-driven spatiotemporal modeling is always used to approximate the battery thermal process. However, purely data-driven spatiotemporal modeling methods suffer from expensive costs for data acquirement, unsatisfactory accuracies for long-term prediction, and poor generalization performance. These inherent shortcomings significantly limit the applications of this kind of method in DPS modeling. To address these issues, we propose a novel physics-reserved spatiotemporal modeling method that embeds the partially known physics into deep learning architecture. Specifically, the proposed method constructs a composite network with the battery current and terminal voltage as extra control inputs to model the battery thermal process. By fully using the information conveyed by the partially known physics and the observed temperature data, it can predict the battery temperature accurately. Meanwhile, it can identify the unknown parameters and estimate the heat generation rate of the battery thermal process, which has not yet been achieved by data-driven spatiotemporal modeling methods. This paper makes an attempt to bridge the gap between physics and observed data for spatiotemporal modeling. The effectiveness of the proposed method has been demonstrated by extensive experiments.
更多查看译文
关键词
Distributed parameter system,Spatiotemporal modeling,Deep learning,Physics,Battery thermal process
AI 理解论文
溯源树
样例
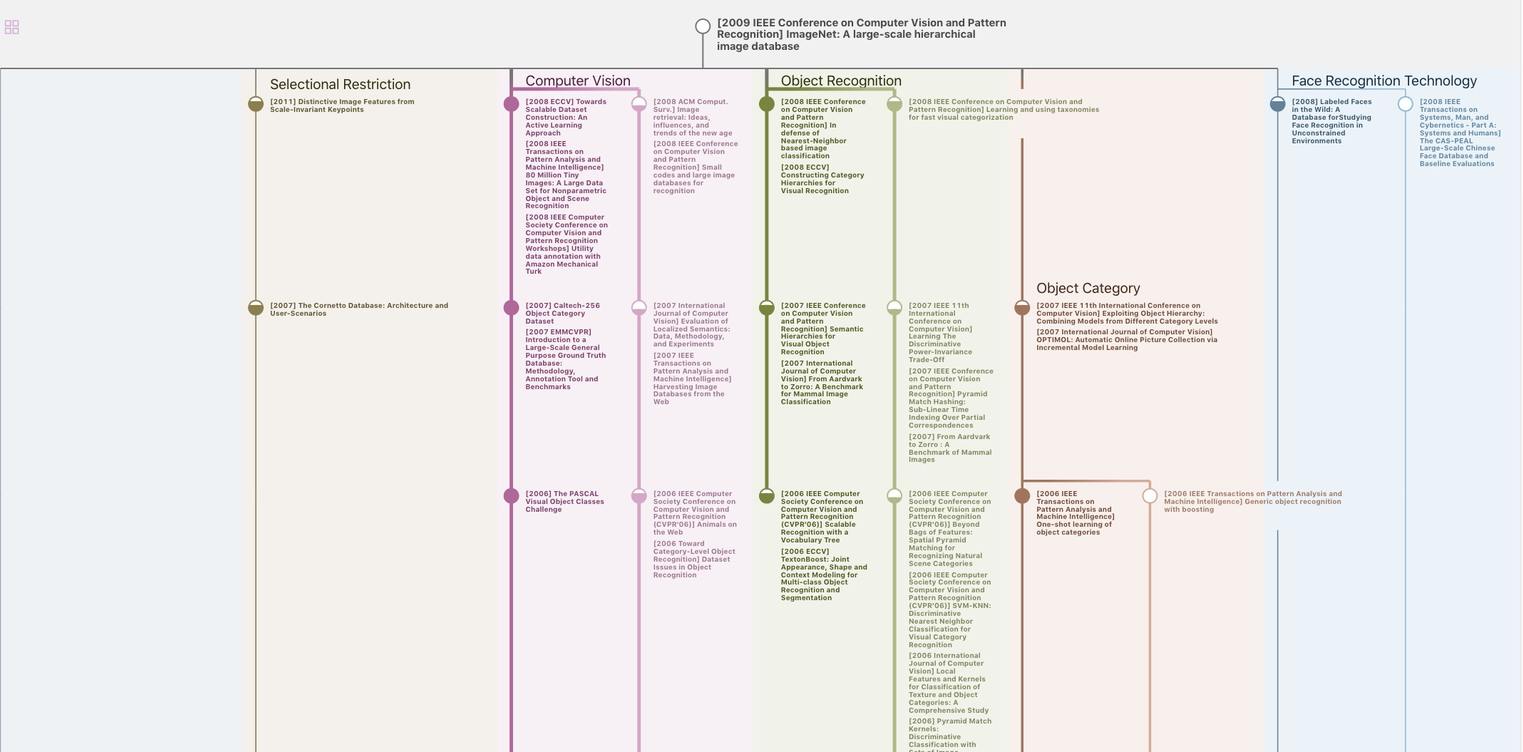
生成溯源树,研究论文发展脉络
Chat Paper
正在生成论文摘要