Vital information is only worth one thumbnail: Towards efficient human pose estimation
PATTERN RECOGNITION(2024)
摘要
In pursuit of impressive performance, existing DCNN-based approaches of human pose estimation usually use massive networks and large -size images to train a deep model. When applying these deep based methods in real-time systems, current works try to compress the deep network by reducing the number of layers and channels, but such approaches are complex and poorly generalized since they require elaborate design of small-scale network structures. Based on the fact that large -size images contain redundant information, in this paper, we explore the influence of image -size on system complexity and propose a novel framework called ThumbPose to accelerate and compress deep models by inferring on thumbnail representations in the task of human pose estimation. In our framework, we first propose a style supervised online downscaler to reduce an input image into a thumbnail image. Furthermore, a training strategy of dual -branch auto -encoding is designed to obtain effective and accurate thumbnail representation in a knowledge distillation manner, which is further used to maintain the performance of thumbnail images as the original -size input images. For heatmap based human pose estimation, ThumbPose is an orthogonal and implementation -friendly method, that can not only compress and accelerate the inference network but also obtain an image downscaler in a supervised manner that can be used in other high-level tasks (e.g. detection, segmentation, etc. in practical applications). Extensive experiments on MS COCO dataset demonstrate the effectiveness of our proposed method, and ThumbPose achieves superior performance (+ 1.3% AP and + 0.7% AR) with negligible additional cost (<0.2 GFLOPs) compared to previous state-of-the-art methods when using small -size images as inputs. Moreover, experiments on MPII show that our model achieves higher accuracy (+ 0.2% Mean@0.5) with minimal computation (2.5 GFLOPs) compared to superior lightweight models obtained by the network compression methods.
更多查看译文
关键词
Human pose estimation,Small-size input,Knowledge distillation,Network compression and acceleration
AI 理解论文
溯源树
样例
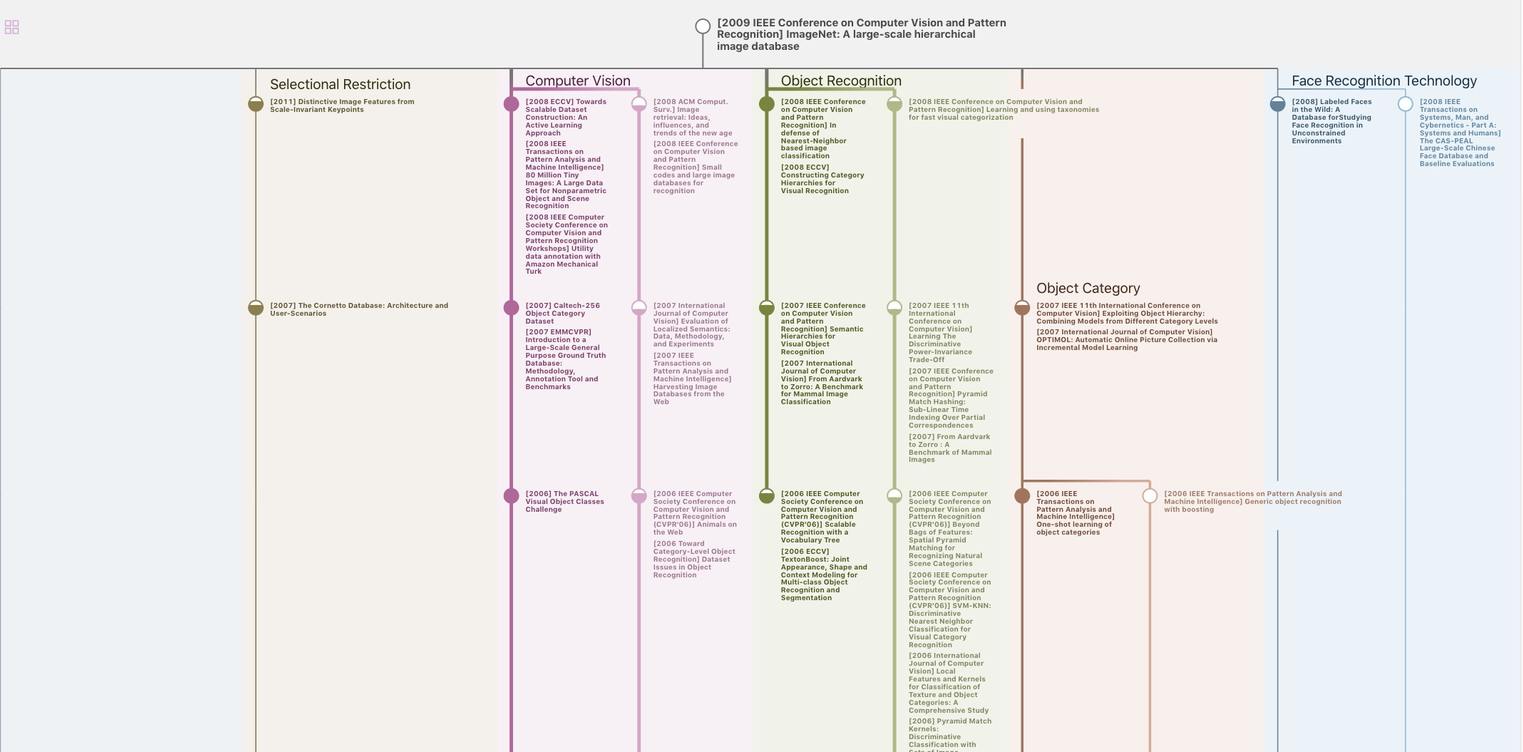
生成溯源树,研究论文发展脉络
Chat Paper
正在生成论文摘要