Quantifying mating behaviour using accelerometry and machine learning: challenges and opportunities
ANIMAL BEHAVIOUR(2024)
摘要
Accelerometry and machine learning are powerful tools for gaining indepth information about animal behaviour. However, such methods are rarely used to quantify behaviours that contribute to mating success, such as intrasexual competition, courtship and copulation. In part, this is probably due to the inherent challenges of classifying behaviours that can be brief and infrequent, and that are not necessarily exhibited by all individuals. In this study, we used triaxial accelerometry and machine-learning methods to quantify the mating behaviours of captive male ruffs, Calidris pugnax. The ruff is a poly-morphic, lekking shorebird with highly skewed mating success. Mating behaviour in ruffs includes ritualized postures that can be distinguished during observations. Using this system as a case study, we (1) describe possible approaches to classifying mating behaviour; (2) compare the classification performance of three supervised machine-learning methods: random forests, hidden Markov models and neural networks; (3) highlight potential pitfalls that can cause overestimation of model performance; and (4) offer suggestions for avoiding these pitfalls. In our study, some models distinguished mating behaviours from nonmating behaviours with high precision and sensitivity (>75%), but only when trained and tested on the same individuals within the same timeframe. Estimates of model performance were much poorer when models were tested on future data or data from different individuals. Nevertheless, even when tested on different individuals, the hidden Markov model provided a reasonably accurate estimate of which males invested more time in mating behaviour overall. Here, we provide an end-to-end workflow for classifying behaviour from accelerometry, including recommendations, con-siderations and code. Although posture-based mating behaviours in ruffs proved challenging to distinguish, the methods that we describe show promise for displays associated with distinctive, dynamic movements.(c) 2023 Published by Elsevier Ltd on behalf of The Association for the Study of Animal Behaviour.
更多查看译文
关键词
accelerometry,behaviourclassification,biologging,courtship,deep learning,hidden Markov,random forest
AI 理解论文
溯源树
样例
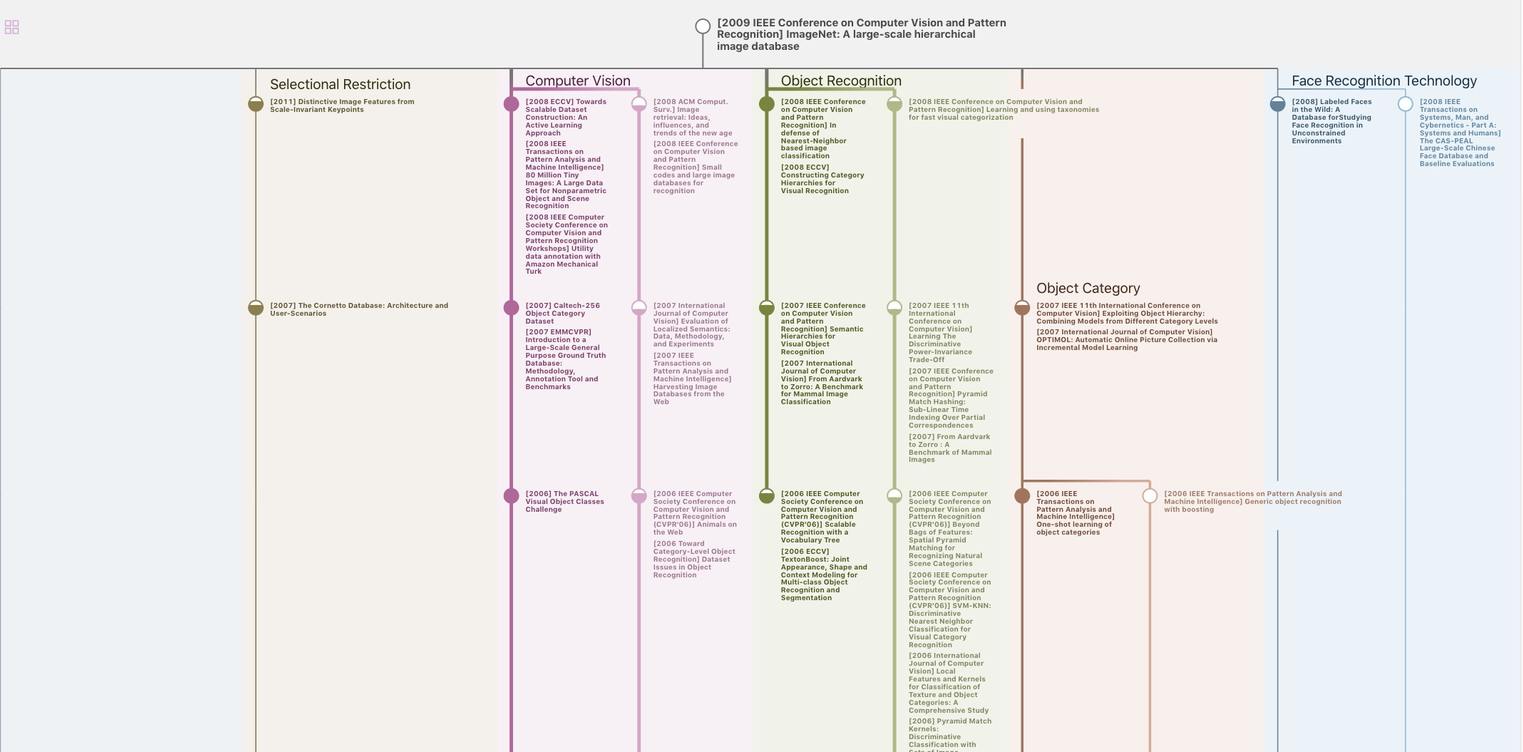
生成溯源树,研究论文发展脉络
Chat Paper
正在生成论文摘要