Microstructure and Dynamic-Mechanical Property of a Selective Laser Melting AISI 316 L Stainless Steel
MATERIALS TODAY COMMUNICATIONS(2023)
Cent South Univ | Spallat Neutron Source Sci Ctr SNSC
Abstract
Dynamic mechanical properties are the key indicators for the application of the selective laser melting AISI 316 L stainless steel (SLM AISI 316 L SS) in impact-related fields. The a precipitate can improve the dynamic mechanical properties of the SLM AISI 316 L SS during high-speed deformation, and promote the application of the SLM AISI 316 L SS in field of the high-speed deformation. Dynamic mechanical properties of the as-built and quenched specimens are tested by using a split Hopkinson pressure bar (SHPB). The specimens exhibit excellent impact energy and ultimate compressive strength, reaching 210.33 MJ/m3 and 1411 MPa, respectively. Microstructure of the SLM AISI 316 L SS is observed by using scanning electron microscopy (SEM) and transmission electron microscopy (TEM). The melt pool boundary and cellular substructure disappear, and the rodlike a precipitates are mainly distributed at the columnar grain boundary after quenching process. Quantitative analysis of the a precipitate is obtained by small angle neutron scattering (SANS). Size and volume fraction of the a precipitates in the quenched specimens can reach 31.5 nm and 2.7%, respectively. Quenched specimens after dynamic deformation mainly produce deformation bands with about 20 mu m width. The a precipitates distributing at the columnar grain boundaries suppress grain growth and dislocation movement by pinning grain boundaries and dislocations, leading to the optimization of the dynamic mechanical properties of the SLM AISI 316 L SS. This work provides theoretical guidance for the strengthening and toughening of dynamic mechanical properties of the SLM AISI 316 L SS.
MoreTranslated text
Key words
Selective laser melting,Stainless steel,Precipitates,Mechanical property
求助PDF
上传PDF
View via Publisher
AI Read Science
AI Summary
AI Summary is the key point extracted automatically understanding the full text of the paper, including the background, methods, results, conclusions, icons and other key content, so that you can get the outline of the paper at a glance.
Example
Background
Key content
Introduction
Methods
Results
Related work
Fund
Key content
- Pretraining has recently greatly promoted the development of natural language processing (NLP)
- We show that M6 outperforms the baselines in multimodal downstream tasks, and the large M6 with 10 parameters can reach a better performance
- We propose a method called M6 that is able to process information of multiple modalities and perform both single-modal and cross-modal understanding and generation
- The model is scaled to large model with 10 billion parameters with sophisticated deployment, and the 10 -parameter M6-large is the largest pretrained model in Chinese
- Experimental results show that our proposed M6 outperforms the baseline in a number of downstream tasks concerning both single modality and multiple modalities We will continue the pretraining of extremely large models by increasing data to explore the limit of its performance
Upload PDF to Generate Summary
Must-Reading Tree
Example
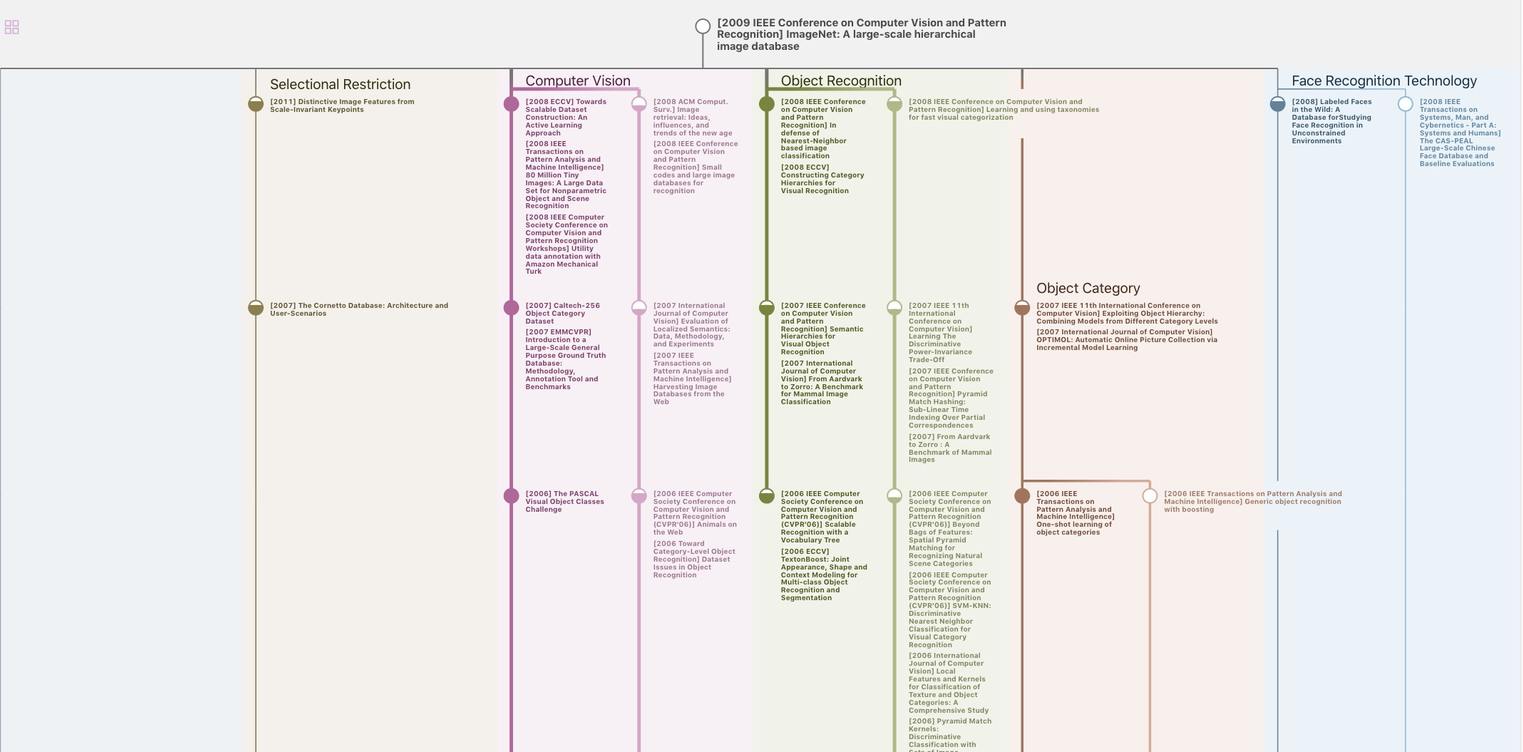
Generate MRT to find the research sequence of this paper
Related Papers
2018
被引用461 | 浏览
2017
被引用209 | 浏览
2018
被引用119 | 浏览
2019
被引用223 | 浏览
2021
被引用8 | 浏览
2021
被引用19 | 浏览
2021
被引用23 | 浏览
2022
被引用10 | 浏览
2023
被引用13 | 浏览
2022
被引用14 | 浏览
2023
被引用3 | 浏览
2023
被引用8 | 浏览
Data Disclaimer
The page data are from open Internet sources, cooperative publishers and automatic analysis results through AI technology. We do not make any commitments and guarantees for the validity, accuracy, correctness, reliability, completeness and timeliness of the page data. If you have any questions, please contact us by email: report@aminer.cn
Chat Paper
GPU is busy, summary generation fails
Rerequest