Dual attention transformer network for pixel-level concrete crack segmentation considering camera placement
AUTOMATION IN CONSTRUCTION(2024)
摘要
Pixel-level crack segmentation remains a challenging task due to the trade-off between computational cost and accuracy, as well as the small size of real-world cracks, typically submillimeter in width, resulting in limited pixels for analysis. To address these challenges, this paper proposes a Pixel Crack Transformer Network (PCTNet) to investigate the impact of different camera placements on network performance. PCTNet adopts a hierarchical structure with Cross-Scale PatchEmbedding Layer and Dual Attention Transformer Block, enabling the generation of multi-scale feature maps and the fusion of global and local features. PCTNet achieves a reduction of up to 64% in computational cost compared to transformer networks while outperforming both convolutional and transformer networks, achieving 95.89% precision, 93.77% recall, 94.8% F1-score, and 90.53% mIoU. Furthermore, this work introduces Crack-R dataset, which encompasses crack images captured at varying distances, facilitating the evaluation of segmentation accuracy in real-world scenarios with different crack-to-pixel ratios.
更多查看译文
关键词
Deep learning,Vision transformer,Crack detection,Semantic segmentation
AI 理解论文
溯源树
样例
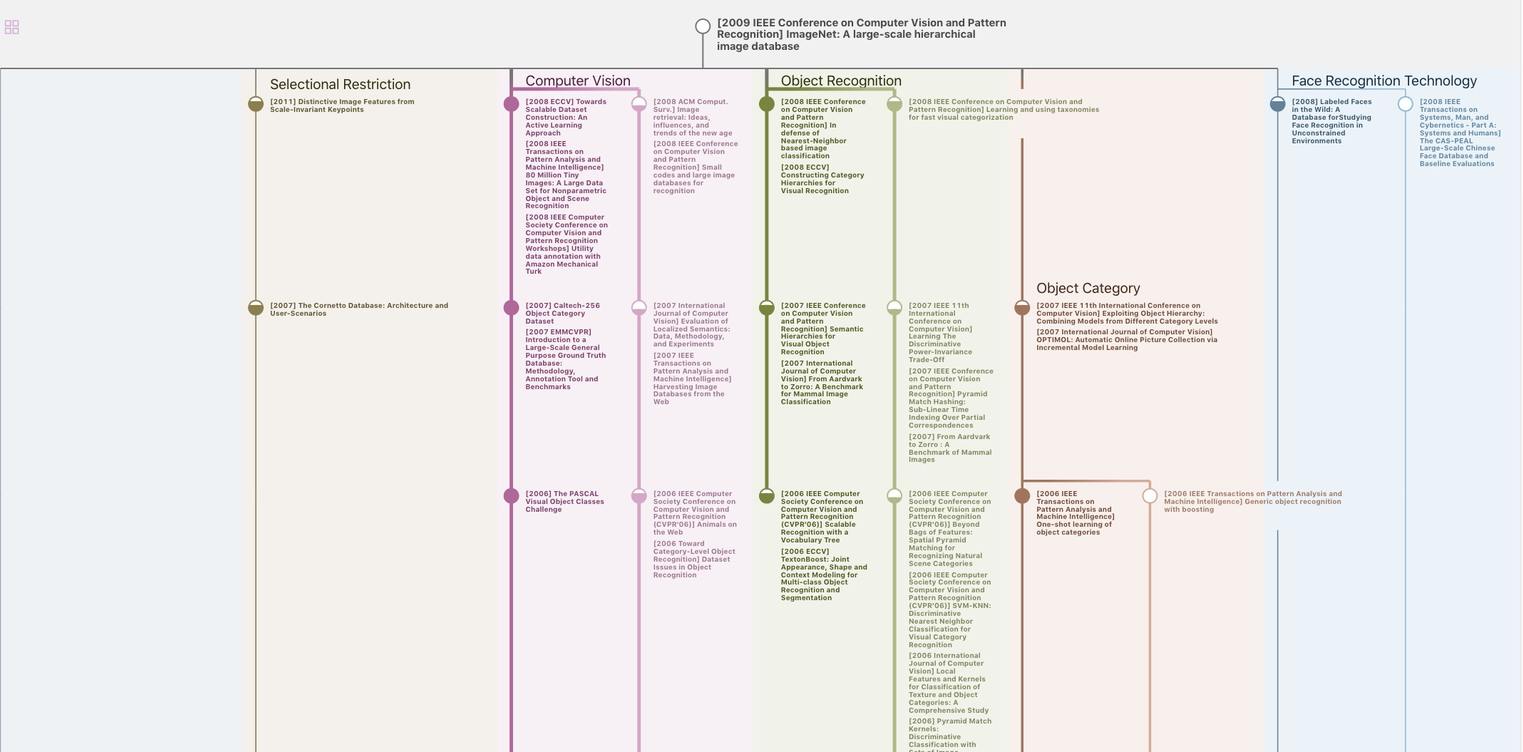
生成溯源树,研究论文发展脉络
Chat Paper
正在生成论文摘要