Evaluation and design of photothermal conversion performance for multiple "complex-morphology" nanofluids via bidirectional deep neural network
Applied Thermal Engineering(2024)
摘要
The optical absorption and scattering of plasmonic nanoparticles are crucial for optimizing photothermal con-version efficiency, which holds great potential in various applications. Evaluating the photothermal conversion performance of nanofluids with known geometry is a computationally expensive task. The design of nanofluids that exhibit optimal photothermal conversion performance poses a complex inverse problem. The necessity to explore a wide parameter space, which encompasses various factors such as the shape, size, and material of nanoparticles, contributes to this challenge. In this study, we employ a combination of machine learning with high-throughput radiative transfer calculations to conduct a comprehensive analysis of the entire photothermal conversion process for various nanofluids. The modulation of resonance absorption peaks and spectral absorption can be achieved by adjusting the shape, material constituents, and geometric parameters of nanoparticles. Meanwhile, we establish a design space dataset that encompasses 14,060 groups of nanofluids. Based on the dataset, we demonstrate as a proof of concept bidirectional deep neural network model that enables an efficient and reliable solution to both the forward and inverse problems. The results show that the absorptivity of SiO2@Au nanofluids is significantly influenced by the volume fraction, particularly when it falls within the range of 1 x 10-5 to 1 x 10-4. However, the impact is significantly reduced within the range of 1 x 10-4 to 1 x 10-3. Furthermore, the SiO2@Au nanofluids exhibit enhanced full spectral absorption characteristics at the core-shell ratio of 0.1. Our proposed model achieves a forward prediction of the solar absorption spectra with 99% accuracy and an inverse design of the geometric parameter with 93% accuracy. In comparison to the experimental results, the relative errors for the predicted and design efficiency are 0.42% and 0.82%, respectively. The design ge-ometry parameters including the effective radius and volume fraction exhibit relative errors of 3.88% and 1.6%, respectively. This work provides a widely applicable and computationally efficient method for the evaluation and design of nanofluids in photothermal conversion.
更多查看译文
关键词
Nanofluids,Plasmonic nanoparticles,Radiative properties,Deep neural network,Solar photothermal conversion
AI 理解论文
溯源树
样例
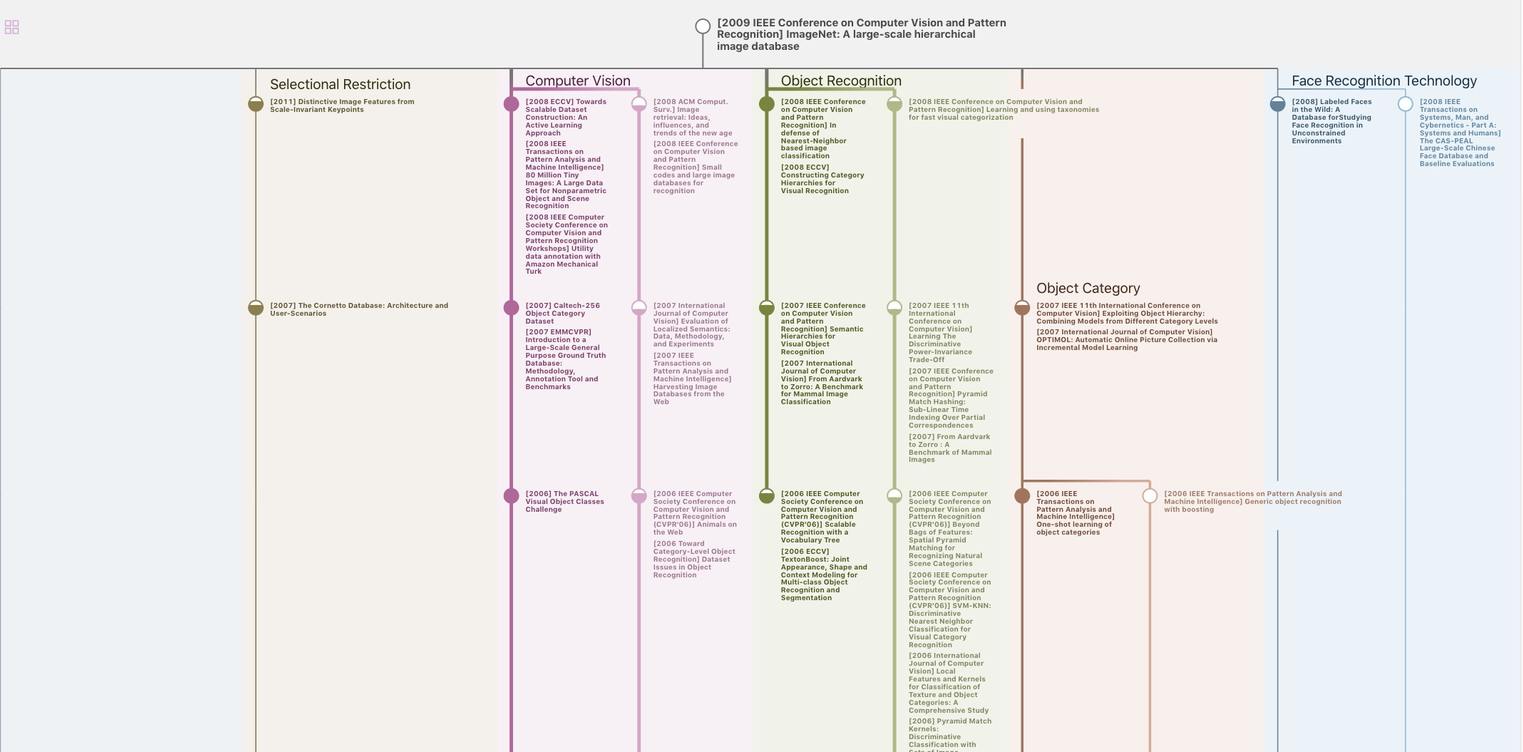
生成溯源树,研究论文发展脉络
Chat Paper
正在生成论文摘要