Learning multi-granular worker intentions from incomplete visual observations for worker-robot collaboration in construction
AUTOMATION IN CONSTRUCTION(2024)
摘要
Construction is often considered a dangerous and labor-intensive industry, with safety and workforce shortages plaguing the industry globally. Owing to its flexibility, Worker-Robot Collaboration (WRC) could combine the strengths of humans and robots, alleviating human workers from dangerous and tedious tasks and boosting productivity. WRC envisions robots assisting workers appropriately, which necessitates the ability to understand workers' intentions. However, challenges arise when robots recognize workers' intentions in construction. First, the highly unstructured and dynamic construction workplace prevents robots from capturing consistent longterm worker activities, which is detrimental to the information accumulation and disambiguation processes in intention recognition. Second, the long-horizon tasks with implicit steps make it difficult for robots to find a suitable granularity to capture workers' intentions. Considering these challenges, this study seeks to (1) explore whether it is feasible to recognize worker intentions with temporally incomplete observations and (2) investigate which granularity is best suited for recognizing worker intentions. To tackle the first challenge, a data-andknowledge fusion method is proposed, where the contextual information from visual data is combined with task knowledge to mitigate the information loss due to incomplete observations. To address the second challenge, the best-suited granularity is determined by comparing the recognition accuracy and intention modeling ability of different granularities after defining and recognizing multi-granular worker intentions using a hierarchical task structure and Multi-Task Learning (MTL), respectively. Results show that the proposed method can recognize multi-granular worker intentions with macro-F1 scores higher than 0.85, and that the intermediate activity is the best-suited granularity as it strikes a good balance between intention recognition accuracy and intention modeling capability. This study contributes to the existing body of knowledge by recognizing multi-granular worker intentions and determining the appropriate granularity under temporally incomplete observations. The findings will help facilitate seamless WRC in construction.
更多查看译文
关键词
Worker-robot collaboration,Intention recognition,Construction task modeling,Task primitive learning,Worker behavior prediction
AI 理解论文
溯源树
样例
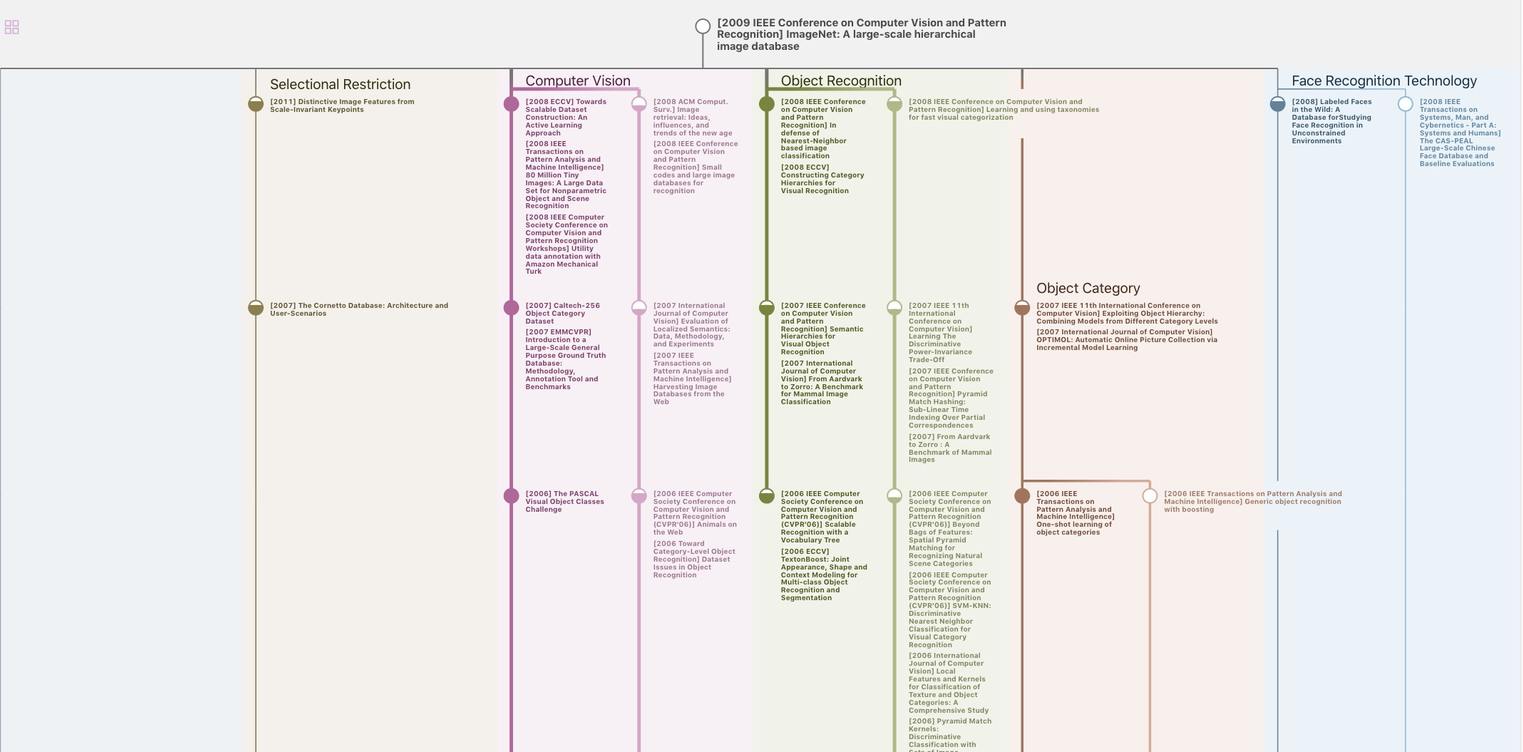
生成溯源树,研究论文发展脉络
Chat Paper
正在生成论文摘要