Transformer models for mining intents and predicting activities from emails in knowledge-intensive processes
ENGINEERING APPLICATIONS OF ARTIFICIAL INTELLIGENCE(2024)
摘要
Process mining is an interdisciplinary field that combines Artificial Intelligence and Business Process Man-agement to extract insights from historical event data. Knowledge-intensive processes, which predominantly involve knowledge work, are often inadequately monitored by process-aware information systems. Conse-quently, the event data necessary for applying process mining techniques are frequently unavailable. Emails are widely used in knowledge-intensive processes for scheduling meetings, sharing documents, and reporting on the completion of outstanding tasks, which makes them suitable candidates for replacing event logs as the primary data source. In this work, we focus on the task of extracting the set of next recommended activities from incoming emails. Yet, we face two major challenges. Firstly, emails do not express process information explicitly but rather contain subtext that implies what the next best actions would be. Secondly, email data lacks domain-specific labels that would enable the use of machine learning. We overcome these limitations by utilizing an email taxonomy to represent user intents, thus bridging the gap between textual information and process semantics, as well as leveraging pre-trained transformer models applied in zero-shot and few-shot settings that require little to no labeled email data. An evaluation of our method on real-world unlabeled email communications demonstrates its effectiveness in recognizing intents and extracting activities.
更多查看译文
关键词
Process mining,Knowledge-intensive processes,Intent recognition in emails,Pre-trained transformers,Zero-shot/few-shot learning
AI 理解论文
溯源树
样例
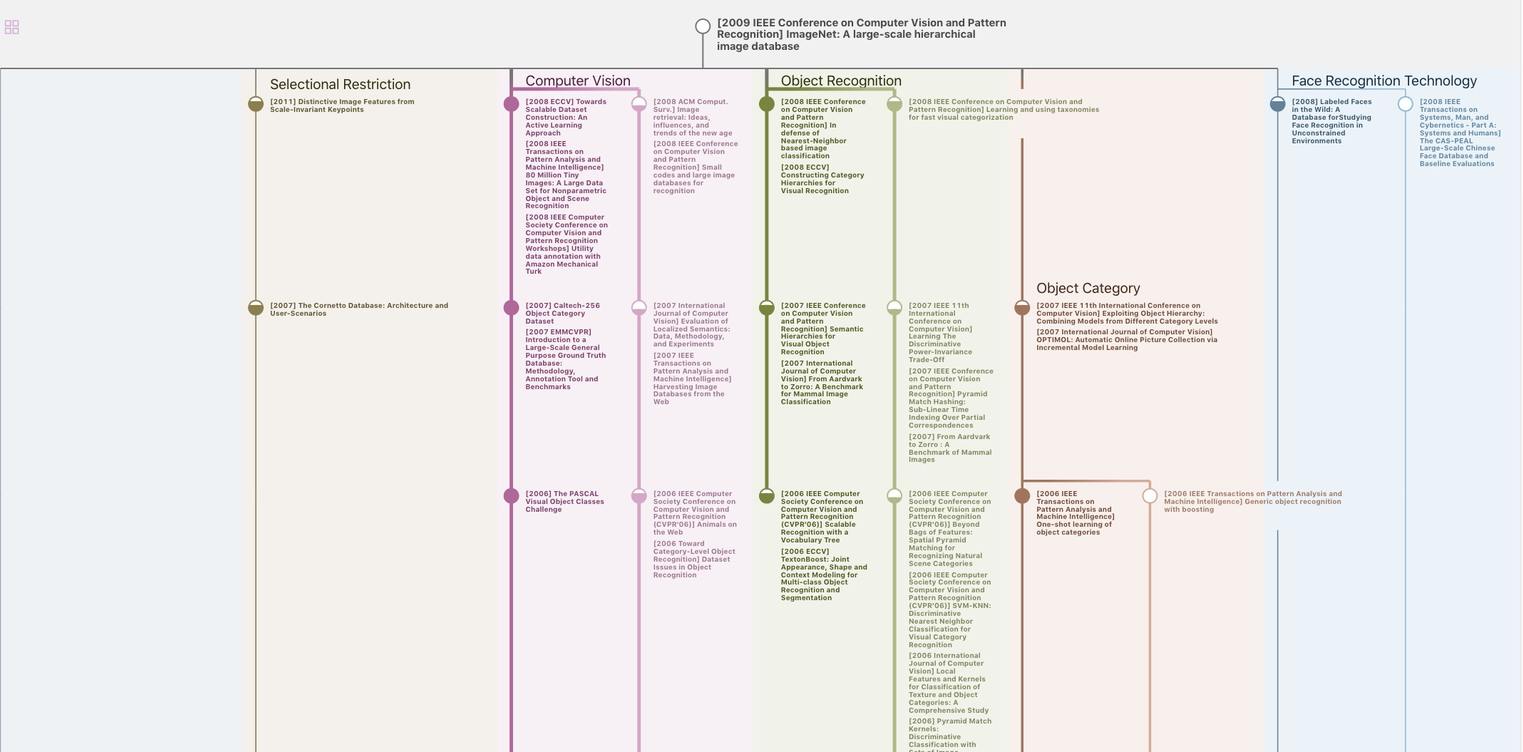
生成溯源树,研究论文发展脉络
Chat Paper
正在生成论文摘要