Deep autoencoder architecture with outliers for temporal attributed network embedding
EXPERT SYSTEMS WITH APPLICATIONS(2024)
摘要
Temporal attributed network embedding aspires to learn a low-dimensional vector representation for each node in each snapshot of a temporal network, which can be capable of various network analysis tasks such as link prediction and node classification. In temporal attributed networks, attribute similarities or link structures of certain nodes may deviate from the regular nodes of the community they belong to, which are called community outlier nodes. However, many existing embedding methods consider only the link structures and their attributes of the nodes adhere to the community structure of the network while ignoring outlier nodes, this can affect the embedding performance of the regular nodes. In this paper, we propose a temporal attributed network embedding framework with outliers, based on autoencoders, to solve the problem. In particular, we propose an outlier-aware autoencoder to model the node information, which combines the current snapshot and previous snapshots to jointly learn embedded vectors of nodes in the current snapshot of a temporal network. In feature preprocessing, we propose a simplified higher graph convolutional mechanism to incorporate attribute information into link structure information, which can leverage attribute features into link structure. Experimental results on node classification and link prediction reveal that our model is competitive against various baseline models.
更多查看译文
关键词
Temporal attributed networks,Network embedding,Outlier nodes,Autoencoders
AI 理解论文
溯源树
样例
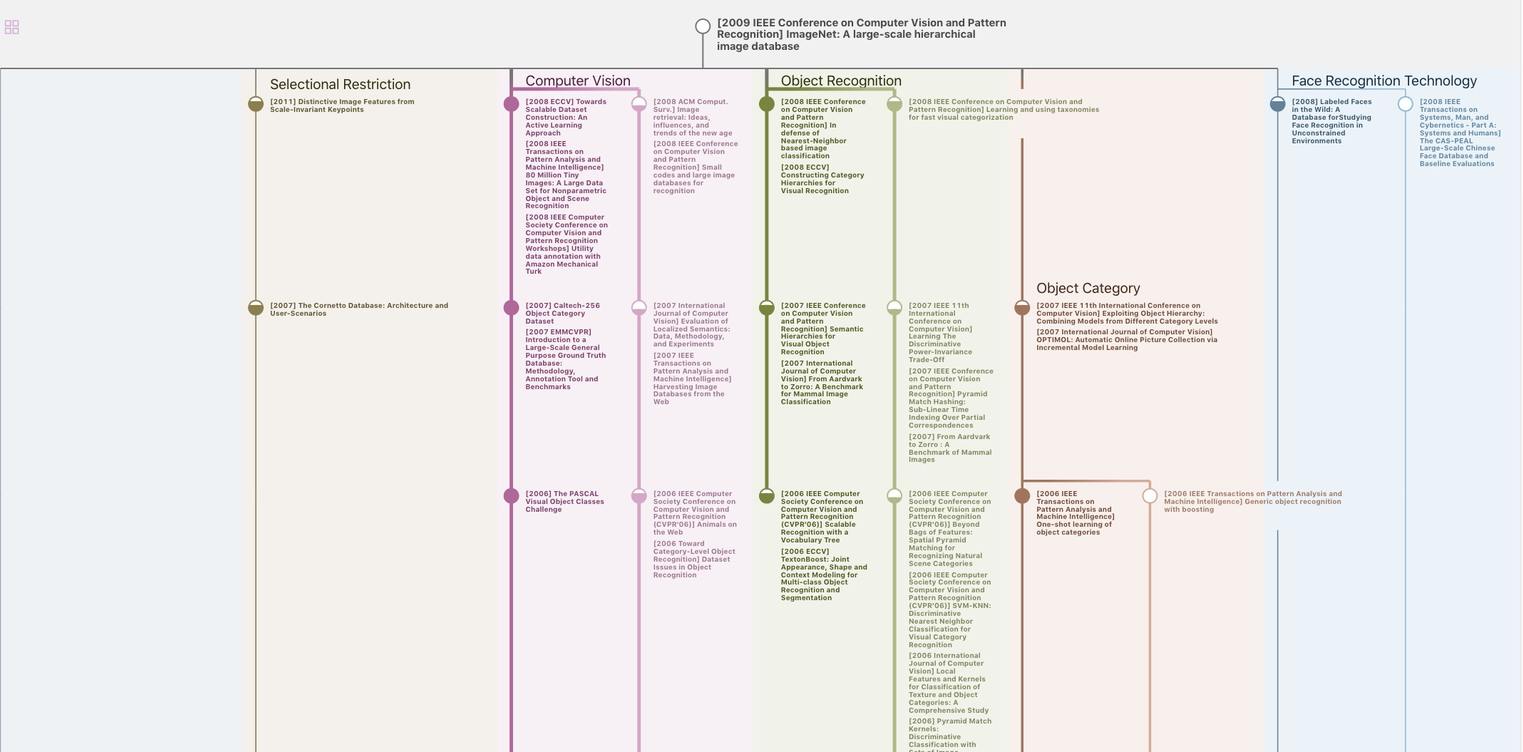
生成溯源树,研究论文发展脉络
Chat Paper
正在生成论文摘要