Physics-informed Deep 1D CNN Compiled in Extended State Space Fusion for Seismic Response Modeling
Computers & Structures(2024)
摘要
Artificial neural networks have been proven promisingly powerful in developing a data-driven surrogate model for rapid seismic response modeling, while very few of them embody a physical mechanism with explicit interpretability. Herewith, this study proposes a novel physics-informed deep 1D convolutional neural network compiled in extended state space fusion (SSM-CNN) for enhanced seismic response modeling. In the SSM-CNN, an innovative parameter-free physics-constrained mechanism is designed and embedded for performance enhancement by construing the differential nexus of state variables derived from the state-space representation of initial structural response. Both the designing philosophy and mechanism translating of the SSM-CNN are elaborated exhaustively. To fully demonstrate the capability and superiority of proposed model compared to normal CNN, two groups of filed records of earthquake events with different sources from PEER and CESMD dataset were allocated for numerical and experimental validations, respectively. The validation results confirmed the effectiveness and superiority of physics-informed SSM-CNN in seismic response prediction. The sensitivity analysis and generalization analysis were also performed on the models for better understanding their interpretability. Involved algorithms and models in present study were integrated and developed into a web program, which affirmed a promising engineering applicability for rapid seismic response prediction.
更多查看译文
关键词
Deep learning,Physics-informed,State space representation,Seismic response modeling
AI 理解论文
溯源树
样例
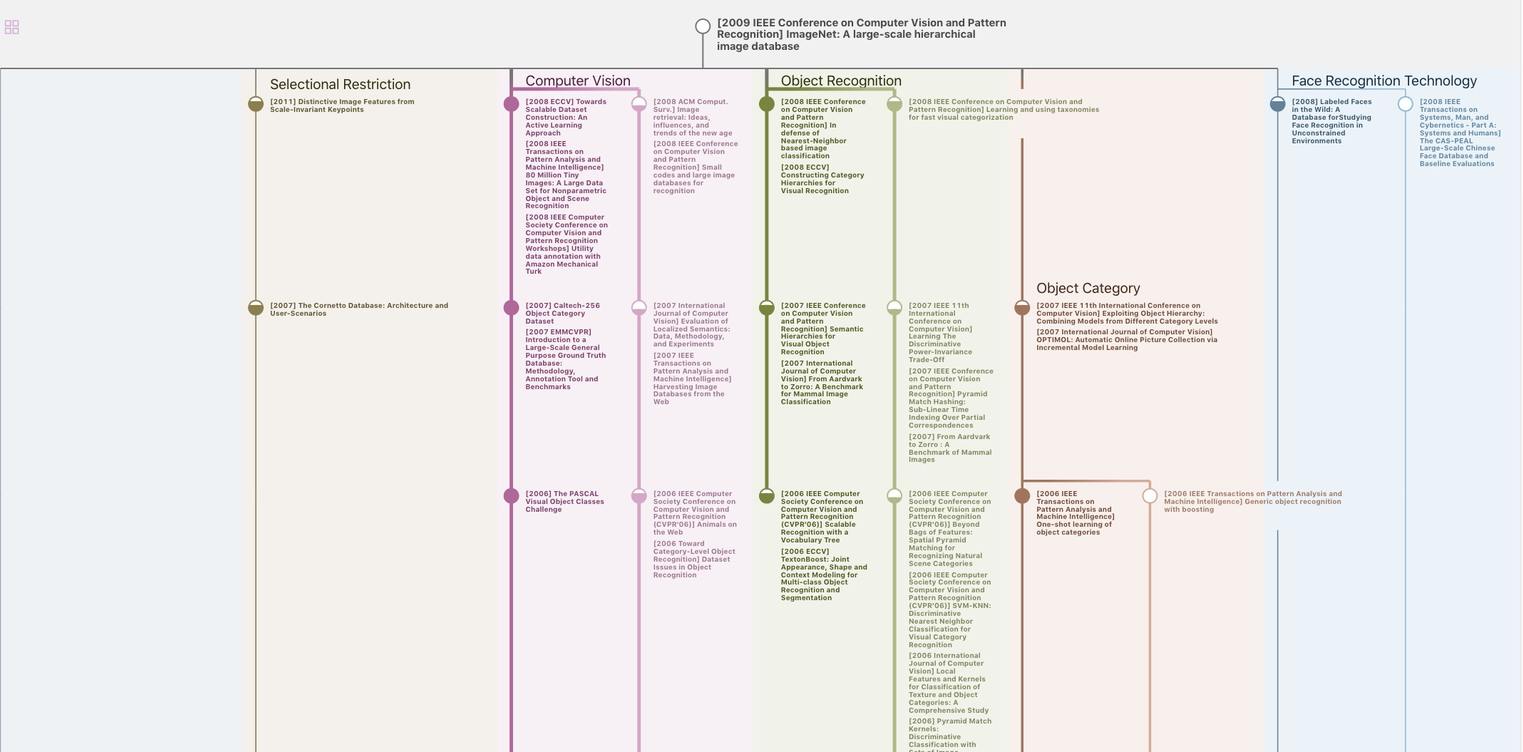
生成溯源树,研究论文发展脉络
Chat Paper
正在生成论文摘要