High-precision cutterhead torque prediction for tunnel boring machines using an attention-based embedded LSTM neural network
MEASUREMENT(2024)
摘要
For avoiding the serious accident caused by the cutterhead jamming of tunnel boring machines, high-precise cutterhead torque (CHT) prediction has important implications for adjusting operational parameters. For accurately predicting the CHT, a novel CHT prediction approach is presented. Firstly, correlation analysis based on cosine similarity are performed to select the monitoring data highly correlated to CHT. Secondly, a cell state extraction module (CSEM) is designed to replace the saturation-prone forget gate for reducing the saturation rate of forget gate. With CSEM, a novel embedded long short-term memory (ELSTM) network with dual-memory structure is proposed and its state transition updating formulas are derived. Lastly, an attention-based deep neural network is designed for fusing the sequential features learned by ELSTM at all instants into the predicted CHTs. Compared with the current approaches, the contrast experiments on an in-situ datasets show that the proposed method has stronger predictive ability under various geological conditions.
更多查看译文
关键词
Cutterhead torque prediction,Long short-term memory (LSTM) neural,network,Multi-source data,Tunnel boring machines
AI 理解论文
溯源树
样例
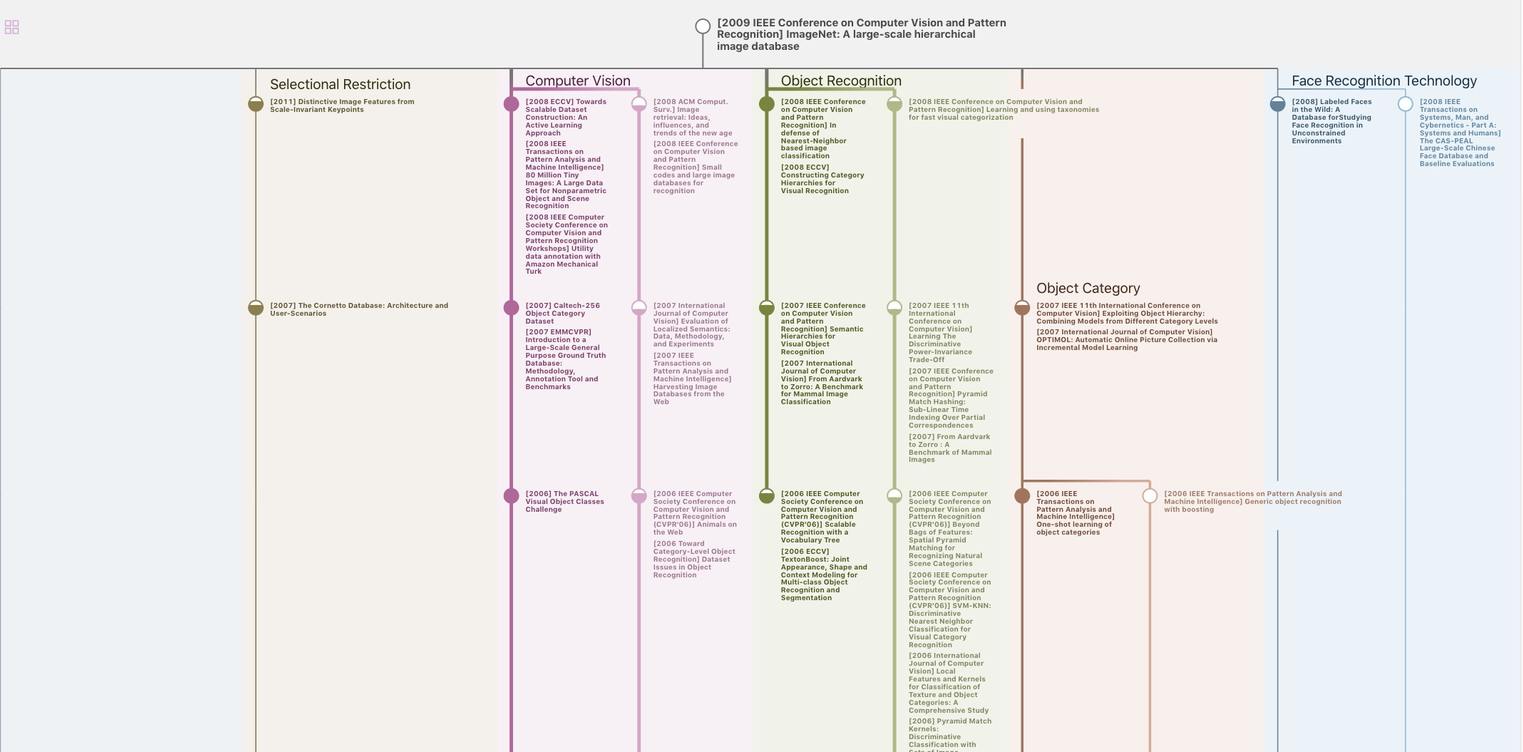
生成溯源树,研究论文发展脉络
Chat Paper
正在生成论文摘要