Quantification of hydrodynamic model sea level bias utilizing deep learning and synergistic integration of data sources
OCEAN MODELLING(2023)
摘要
This study demonstrates the use of machine learning strategies to examine and quantify the bias that often exists in sea level data from hydrodynamic models. The sea level bias is considered to consist of two components: (i) hydrodynamic modelling errors due to numerical modelling limitations, and (ii) a bias related to the difference between vertical datums. The goal is to accurately quantify these components, enabling the determination of absolute dynamic topography from coastal to offshore areas. The method is tested in the Baltic Sea employing a synergy of hydrodynamic models, tide gauges, and satellite altimetry.Firstly, a multivariate deep neural network approach inspired by WaveNet is used to identify and quantify hydrodynamic modelling errors. A wrapper-type sequential feature elimination algorithm identifies seven rele-vant variables out of the initially considered sixteen for training the deep leaning model in the Baltic Sea region. The model is trained using sixteen tide gauge records. As a result, the model predicts hydrodynamic modelling errors with a root mean squared error of 3.2 cm and 3.4 cm, and an R-Squared value of 0.82 and 0.77 for the training and test sets, respectively. Comparing the predicted and observed errors reveals localized areas where other sea level dynamics, such as seiches in the Gulf of Riga, may be of interest but were not incorporated into the deep learning model. Secondly, once the hydrodynamic modelling errors are quantified, the method allows for the determination of the vertical reference bias by comparing known and reliable observations, such as tide gauge and satellite altimetry data. The vertical reference bias is calculated to be 18.1 +/- 2.9 cm.The method significantly improves the accuracy of dynamic topography, resulting in an average root mean squared error of 4.1 cm compared with satellite altimetry and a correlation of 0.98 compared with tide gauges. This approach presents a novel way to integrate modelled and observed dynamic topography using machine learning techniques for enhancing our understanding and its applications.
更多查看译文
关键词
Absolute dynamic topography,Hydrodynamic model,Deep learning network,Vertical reference datum,Hydrogeodesy,Sea level
AI 理解论文
溯源树
样例
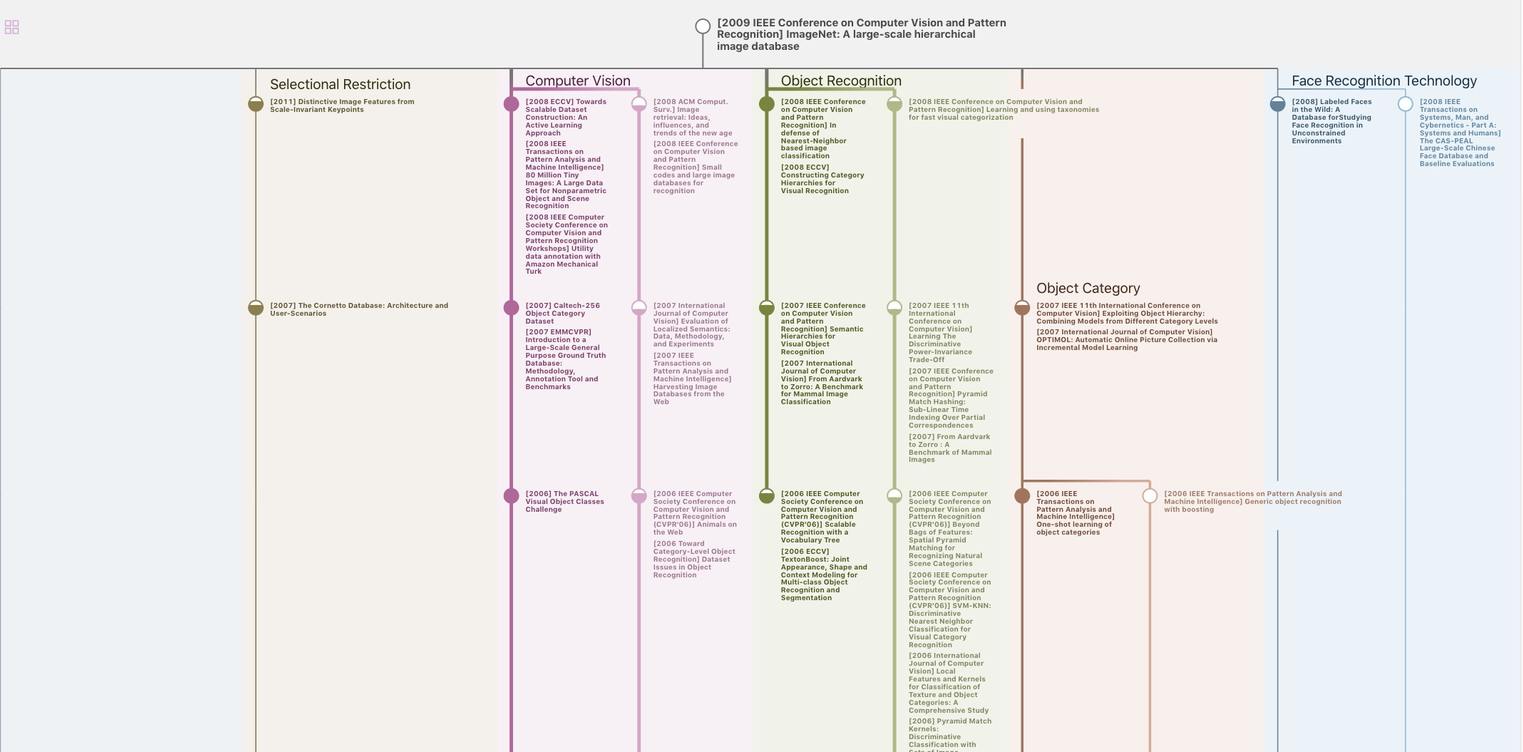
生成溯源树,研究论文发展脉络
Chat Paper
正在生成论文摘要