Characterization of the neuronal and network dynamics of liquid state machines
PHYSICA A-STATISTICAL MECHANICS AND ITS APPLICATIONS(2024)
摘要
Reservoir computing (RC) is a relatively new machine-learning framework that uses an abstract neural network model, called reservoir. The reservoir forms a complex system with high dimensionality, nonlinearity, and intrinsic memory effect due to recurrent connections among individual neurons. RC manifests a best-in-class performance in processing information generated by complex dynamical systems, yet little is known about its microscopic/macroscopic dynamics underlying the computational capability. Here, we characterize the neuronal and network dynamics of liquid state machines (LSMs) using numerical simulations and Modified National Institute of Standards and Technology (MNIST) database classification tasks. The computational performance of LSMs largely depends on a dynamic range of neuronal avalanches whereby the avalanche patterns are determined by the neuron and network models. A larger dynamic range leads to higher performance-the MNIST classification accuracy is highest when the avalanche sizes follow a slowly decaying power-law distribution with an exponent of similar to 1.5, followed by the power-law statistics with a larger exponent and the mixture of power-law/log-normal distributions. Network-theoretic analysis suggests that the formation of large functional clusters and the promotion of dynamic transitions between large and small clusters may contribute to the scaleinvariant nature. This study provides new insight into our understanding of the computational principles of RC concerning the actions of the individual neurons and the system-level collective behavior.
更多查看译文
关键词
Reservoir computing (RC),Liquid state machine (LSM),Neuronal avalanche,Network clustering,Scale invariance,Self-organized criticality (SOC)
AI 理解论文
溯源树
样例
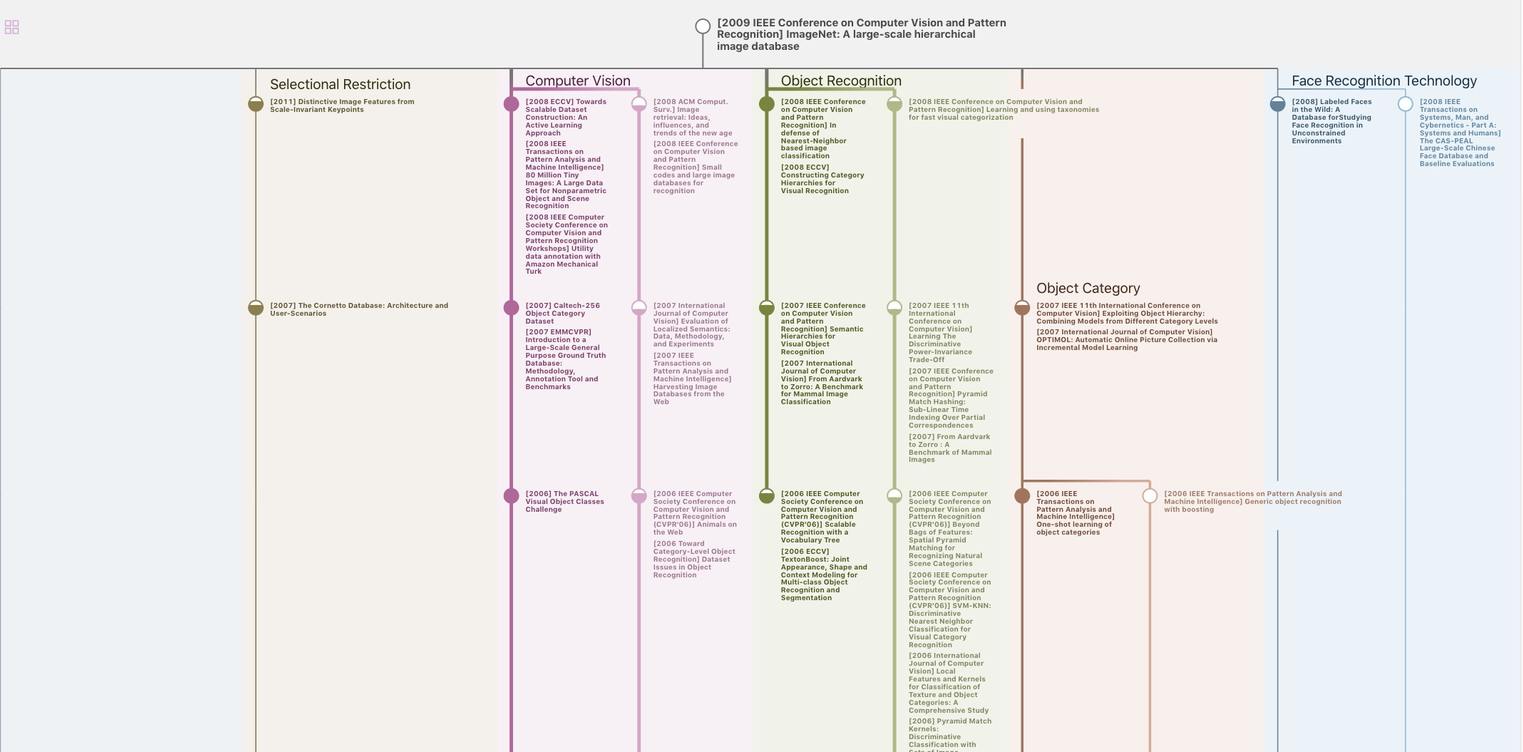
生成溯源树,研究论文发展脉络
Chat Paper
正在生成论文摘要