Generating probability distributions on intervals and spheres: Convex decomposition
COMPUTERS & MATHEMATICS WITH APPLICATIONS(2024)
摘要
This work studies the convexity of a class of positive definite probability measures with density functions rho = c(lambda) Sigma(infinity)(n=0) g(n)C(n)(lambda)w(lambda), that are generated through the interval characteristic sequences. We discuss when such measures supported in an interval are convex and demonstrate how convexity is preserved under multiplication by certain multipliers and under the intertwining product. A key message to be delivered from this work is: any integrable function f on the interval with a polynomial expansion that has relatively fast absolute convergence, can be decomposed into a non-unique pair of positive convex interval probabilities (f(+),f(-)) is an element of epsilon(lambda)'X epsilon(lambda)' up to normalization, in the sense that f =f(+),f(-), where epsilon(lambda)' is a class of convex functions. Consequently not only the study of an interval distribution is reduced to the study of the properties of class. '.., but also the discontinuous probabilistic Galerkin schemes can be simplified. As concrete examples the decomposition of polynomials and truncated normal distribution are computed and graphically illustrated. Such interval measures lead to localized spherical probability measures, and as a convex expression of probability measure is a Jensen type inequality for spherical random variables. Based on the spherical information entropy models, one dependent on the domain partition and the other not, we propose three minimization formulations for the probabilistic finite element method, including one that mixes the least-squares and entropy models.
更多查看译文
关键词
Interval characteristic sequence,Interval probability measure,Convex decomposition,Probabilistic finite element method,Spherical information entropy
AI 理解论文
溯源树
样例
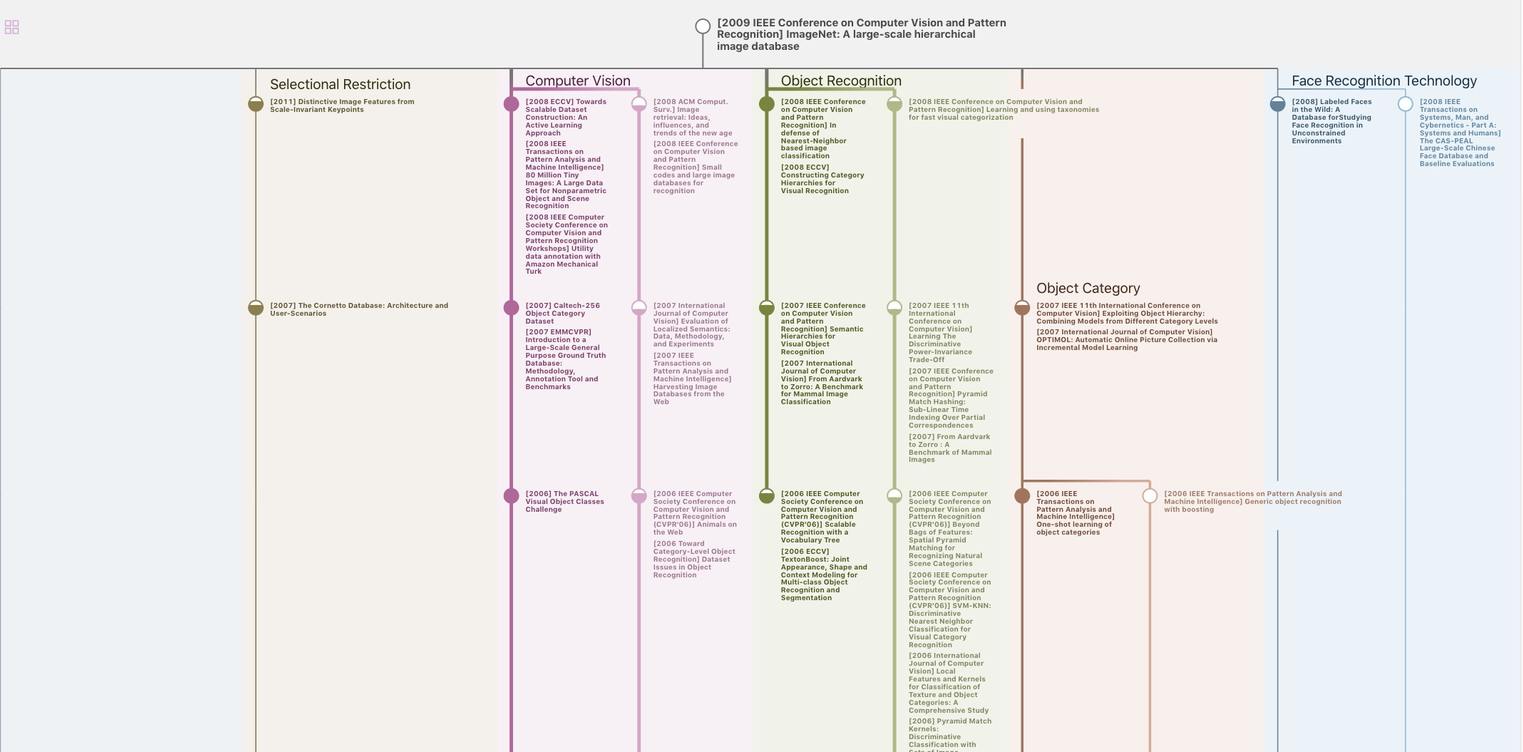
生成溯源树,研究论文发展脉络
Chat Paper
正在生成论文摘要