On the regularization in DeePC
IFAC PAPERSONLINE(2023)
摘要
Data-enabled predictive control (DeePC) is an approach to data-driven direct control that has gained considerable interest recently. In this work we show that the main equations of DeePC can be derived from a linear regression model based on the input/output equations for a linear system that can be estimated using linear least squares. Our main contribution is an analysis showing that DeePC-using different types of regularization-gives predictions that equals those of an estimated model if the regularization weight is large enough. The numerical example indicate that the optimal weights are sufficiently large for all considered types of regularization. This suggests that the use of an indirect method based on the linear regression model implicitly estimated by DeePC can be beneficial, since this avoids tuning of weights, does not distort the control criterion and makes the online optimization considerably faster. Furthermore, a slightly modified estimate is considered, that reduces the number of unknown parameters in the linear regression model by taking causality into account. Copyright (c) 2023 The Authors.
更多查看译文
关键词
Data-driven control,Predictive control,Regularization,Finite-horizon control.
AI 理解论文
溯源树
样例
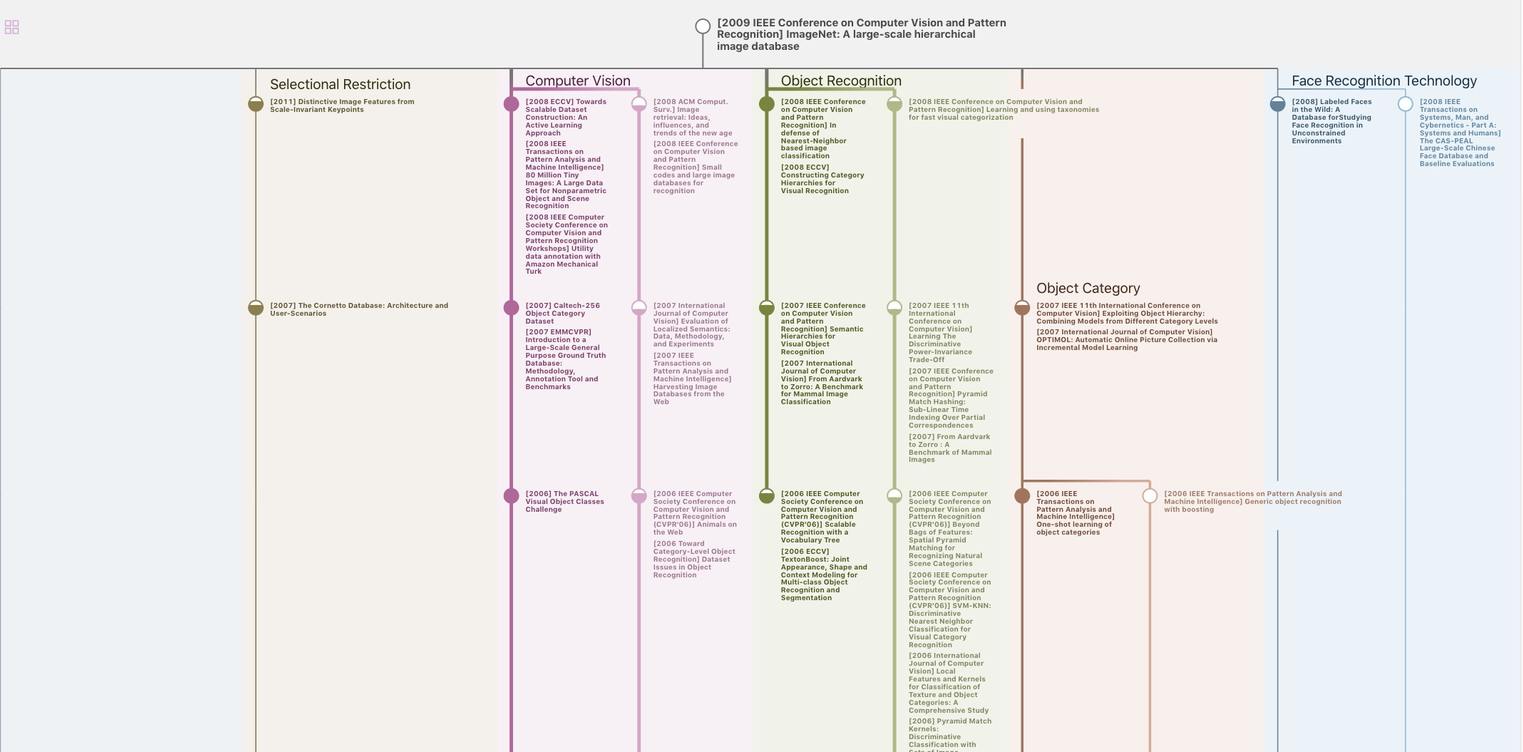
生成溯源树,研究论文发展脉络
Chat Paper
正在生成论文摘要