Integrating data-informativity conditions in predictor models for single module identification in dynamic networks
IFAC PAPERSONLINE(2023)
摘要
For consistent identification of a target module in a dynamic network with the local direct method, basically two prime conditions need to be satisfied: (a) a set of structural conditions on the choice of the predictor model, i.e. a set of input and output node variables, and (b) conditions on data-informativity. While for conditions (a) constructive algorithms for node selection have been presented that appropriately guarantee that the identified object can indeed reveal the target module, the requirements for satisfying (b) have not yet been integrated fully. In this paper, we will present simplified path-based results for generic data-informativity, and show how they can be integrated in constructive algorithms for predictor model selection that provide consistent target module estimates. It is shown that data-informativity not only requires a sufficient number of external excitation signals to be present in the network, but also puts restrictions on the structure of the predictor model, i.e. the selection of input and output node variables. Some examples are presented to illustrate the new results. Copyright (c) 2023 The Authors.
更多查看译文
关键词
System identification,identifiability,dynamic networks,interconnected systems
AI 理解论文
溯源树
样例
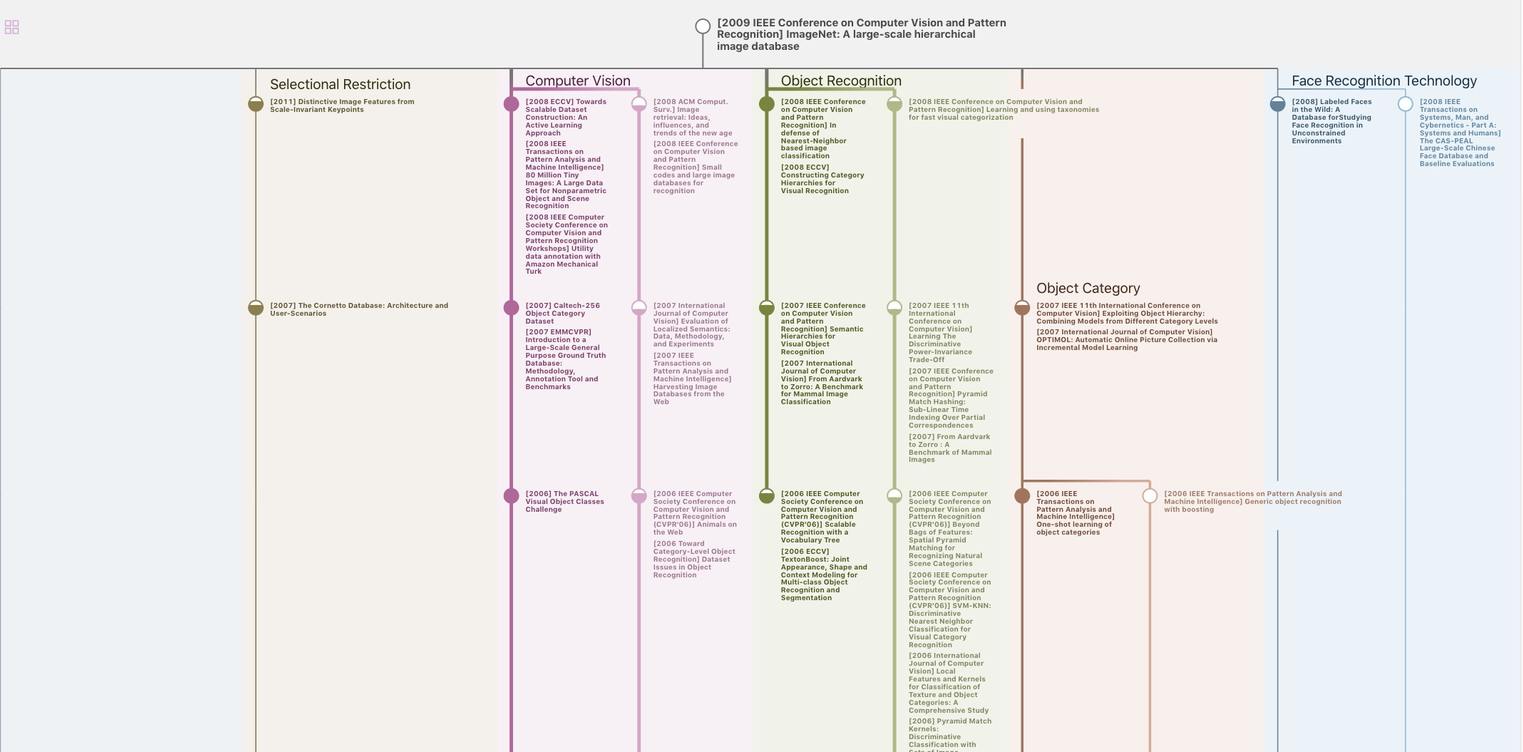
生成溯源树,研究论文发展脉络
Chat Paper
正在生成论文摘要