Adaptive Parameterized Control for Coordinated Traffic Management Using Reinforcement Learning
IFAC PAPERSONLINE(2023)
摘要
Traffic control is essential to reduce congestion in both urban and freeway traffic networks. These control measures include ramp metering and variable speed limits for freeways, and traffic signal control for urban traffic. However, current traffic control methods are either too simple to respond to complex traffic environment, or too sophisticated for real-life implementation. In this paper, we propose an adaptive parameterized control method for traffic management by using reinforcement learning algorithms. This method takes advantage of the simple structure of parameterized state-feedback controllers for traffic; meanwhile, a reinforcement learning agent is employed to adjust the parameters of the controllers on-line to react to the varying environment. Therefore, the proposed method requires limited real-time computational efforts, and is adaptive to external disturbances. Furthermore, the reinforcement learning agent can coordinate multiple local traffic controllers when adjusting their parameters. The method is validated by a numerical case study on a freeway network. Results show that the proposed method outperforms conventional controllers when the system is exposed to a changing environment. Copyright (c) 2023 The Authors.
更多查看译文
关键词
Parameterized control,adaptive control,reinforcement learning,coordinated control,traffic network system
AI 理解论文
溯源树
样例
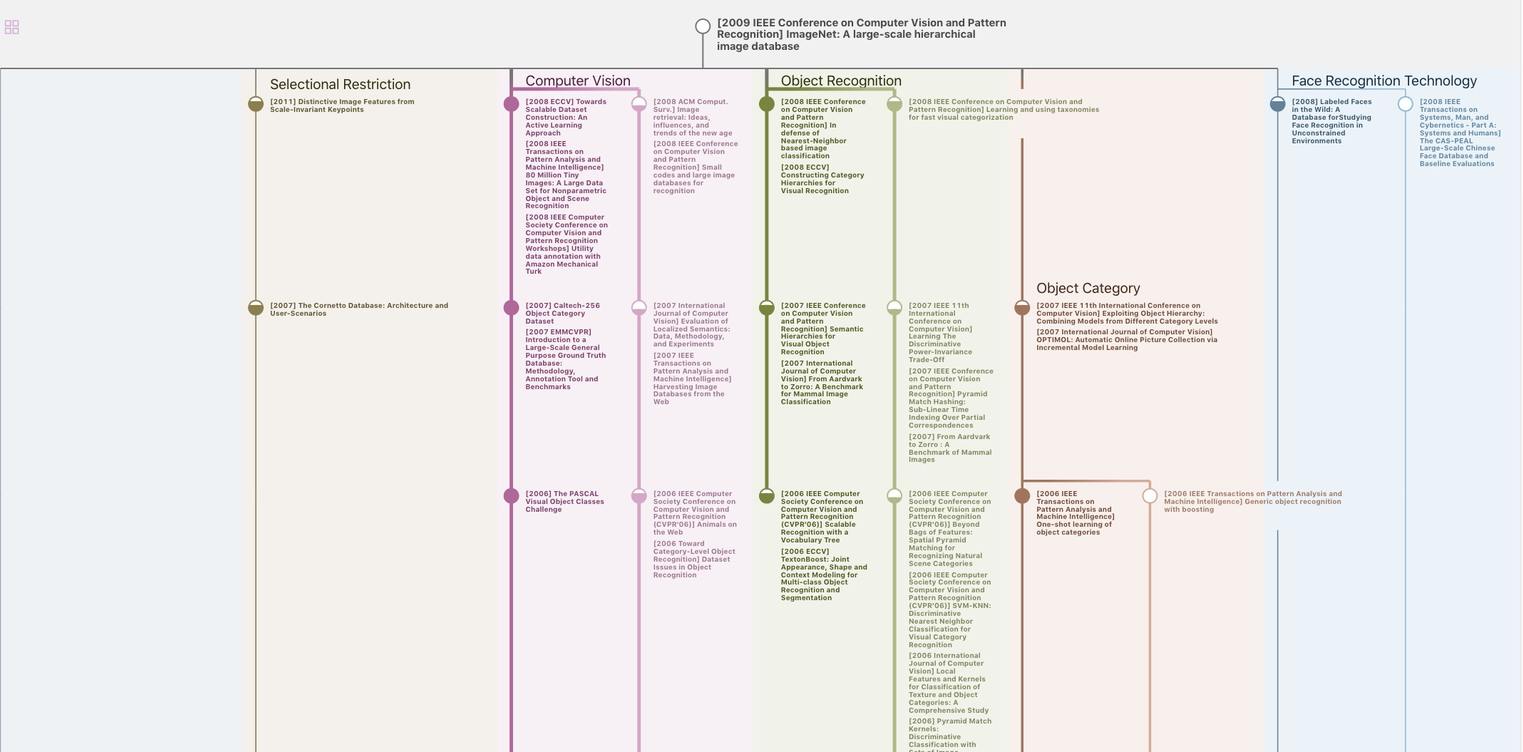
生成溯源树,研究论文发展脉络
Chat Paper
正在生成论文摘要