Multi-pass Extended Kalman Smoother with Partially-known Constraints for Estimation of Vapor Compression Cycles
IFAC-PapersOnLine(2023)
摘要
State and parameter estimation methodologies have the potential to make a significant impact in the development of broad array of capabilities for widely-used vapor compression cycles, including advanced controls, performance monitoring, data-driven modeling, and deployment of digital twin technologies. However, the nonlinearity and numerical stiffness of large physics-based models of these systems pose challenges for the practical implementation of estimators that must also satisfy the physical state constraints. We present a three-pass fixed-interval smoothing method developed in the extended Kalman estimation formalism that incorporates linear inequality and partially-known nonlinear equality constraints defined in terms of unknown parameters of the system. The smoothing method is demonstrated to have high estimation accuracy during joint state and parameter estimation of the cycle model representing a realistic system that is implemented in Julia language leveraging automatic differentiation capabilities.
更多查看译文
关键词
Constrained estimation,Kalman filtering and smoothing,nonlinear state and parameter estimation,vapor compression cycle,HVAC
AI 理解论文
溯源树
样例
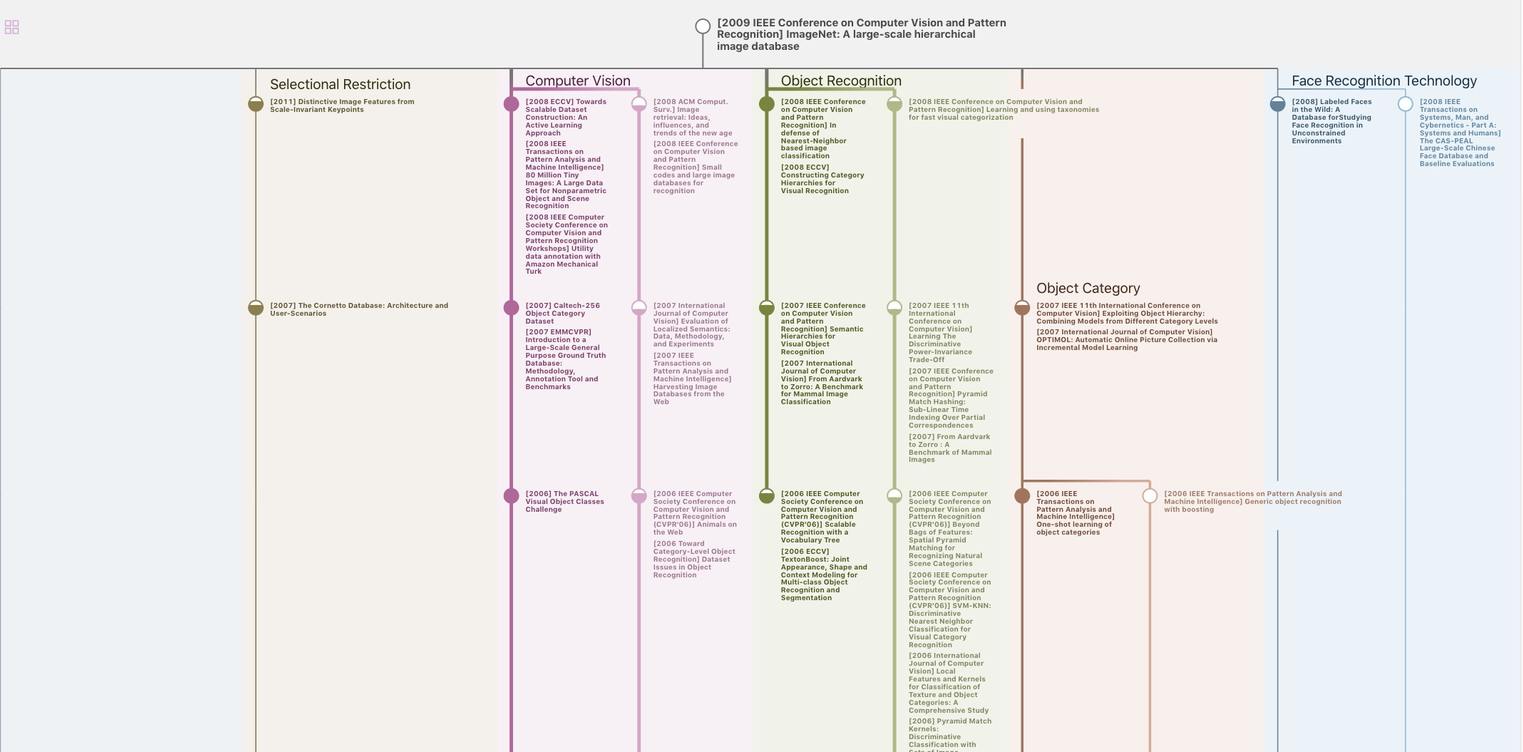
生成溯源树,研究论文发展脉络
Chat Paper
正在生成论文摘要