Deep Recurrent Neural Network-Based Observer for Uncertain Nonlinear Systems
IFAC PAPERSONLINE(2023)
摘要
Recurrent neural networks (RNNs) have gained popularity in various applications like handwriting recognition, time series prediction, and system identification due to their ability to retain state information for later use through temporal operations present in the RNN architecture. The dynamic behavior of RNNs makes them well-suited for the identification of nonlinear systems. Several training methods for adaptive RNN observers have been previously developed, but those methods are restricted to either RNNs satisfying a linear-in-the-parameters assumption or RNNs with a single hidden layer. While deep neural networks have been shown to yield improved function approximation performance compared to neural networks with a single hidden-layer, their complex structure and nested nonlinearities make real-time weight adaptation a difficult problem. In this paper, an adaptive deep RNN observer is developed for identification of a class of nonlinear systems. The developed deep RNN architecture adjusts in real-time to system uncertainties through a Lyapunov-derived weight adaptation law, where a filtered estimation error is designed and implemented in the weight adaptation law to relax full-state feedback requirements. A Lyapunov-based stability analysis is performed to ensure asymptotic convergence of the estimation errors and stability of the adaptive deep RNN architecture. Copyright (c) 2023 The Authors. This is an open access article under the CC BY-NC-ND license (https://creativecommons.org/licenses/by-nc-nd/4.0/)
更多查看译文
AI 理解论文
溯源树
样例
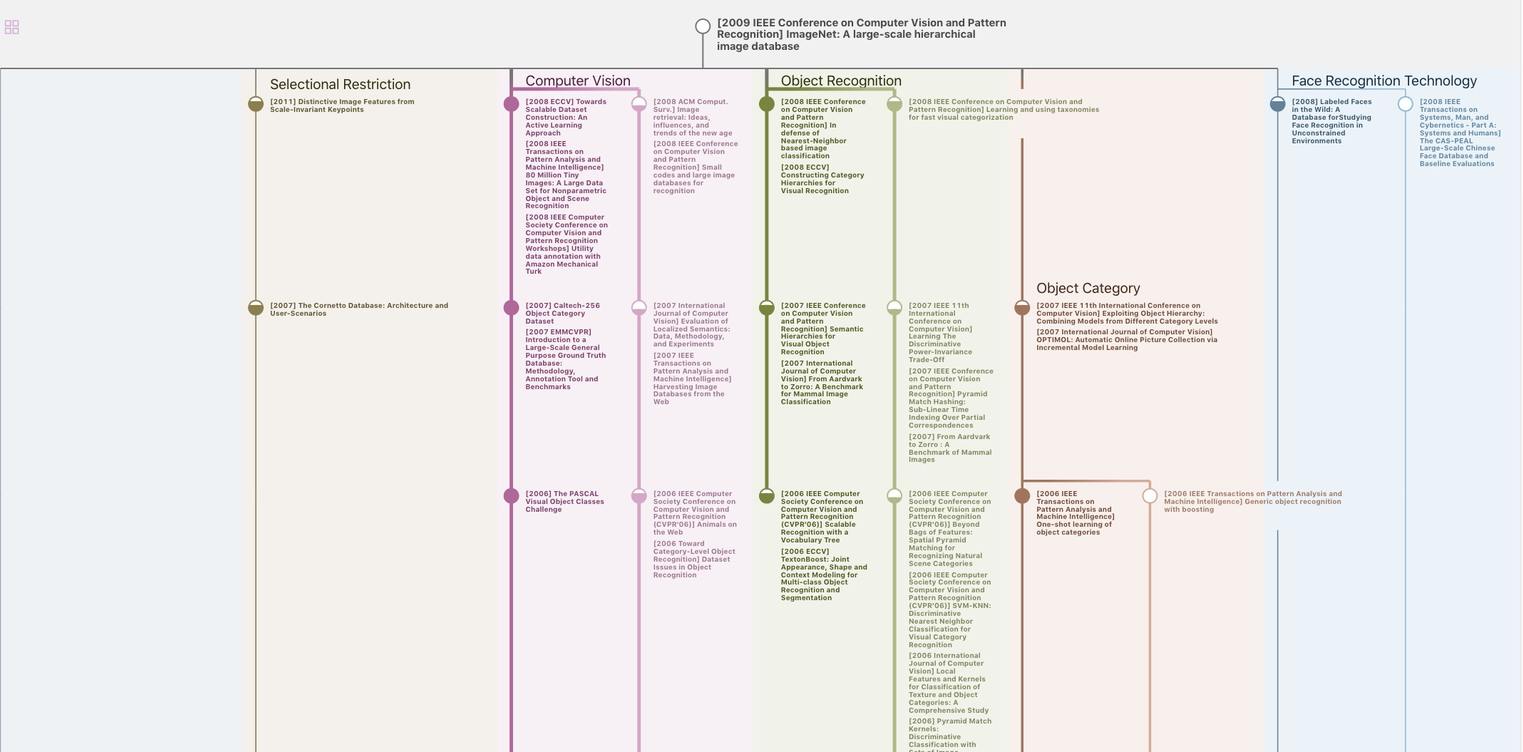
生成溯源树,研究论文发展脉络
Chat Paper
正在生成论文摘要