Largest Connected-ERFNet for Autonomous Railway Track Detection and Real-time Tracking
IFAC PAPERSONLINE(2023)
摘要
Unmanned aerial vehicle (UAV) is expected to have the potential to complete the collection of defect information in track areas with lower labor costs and higher efficiency. In this field, autonomous railway track detection and real-time tracking to guide UAVs are quite essential. However, the limited computation resources of the UAV onboard computer make it difficult to maintain high accuracy in realtime detection and tracking using the deep learning model with a complex structure. Concerning this issue, for the daily detection scene of the track, this paper proposes a novel autonomous railway track detection and real-time tracking algorithm named Largest Connected-ERFNet, which combines ERFNet and the largest connected component labeling to ensure the accuracy of the track area detection and tracking. A comprehensive set of experiments on UAV onboard computer are conducted for verification. Experiments demonstrate the superior performance of the algorithm proposed in this paper. Under the condition of limited training data and computation resources, the detection precision of the algorithm reaches 89.2%, the detection speed reaches 5.5 fps, and the smoothness reaches 99.4%. It is proven that the proposed method can meet the practical needs of using UAVs for railway track inspection. Copyright (c) 2023 The Authors.
更多查看译文
关键词
fault information collection,railway track inspection,UAV,Semantic segmentation,largest connected component labeling,remote sensing images
AI 理解论文
溯源树
样例
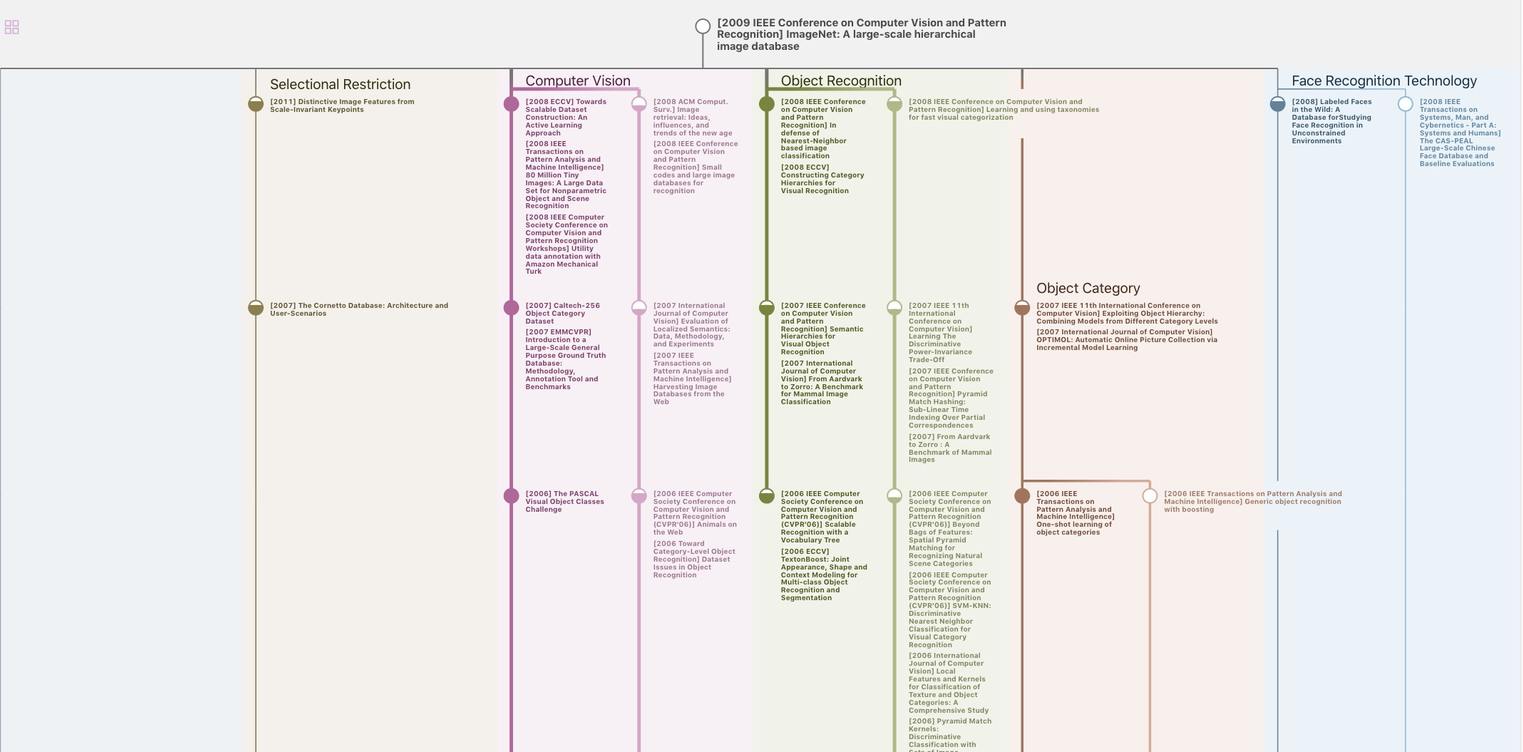
生成溯源树,研究论文发展脉络
Chat Paper
正在生成论文摘要