Mixed H2/H∞-Policy Learning Synthesis
IFAC-PapersOnLine(2023)
摘要
A robustly stabilizing optimal control policy in a model-free mixed H2/H∞-control setting is here put forward for counterbalancing the slow convergence and non-robustness of traditional high-variance policy optimization (and by extension policy gradient) algorithms. Leveraging Itô’s stochastic differential calculus, we iteratively solve the system's continuous-time (closed-loop) generalized algebraic Riccati equation(GARE) whilst updating its admissible controllers in a two-player, zero-sum differential game setting. Our new results are illustrated by learning-enabled control systems which gather previously disseminated results in this field in one holistic data-driven presentation with greater simplification, improvement, and clarity.
更多查看译文
关键词
Robust control,Data-driven optimal control,Machine learning in modelling, prediction, control and automation
AI 理解论文
溯源树
样例
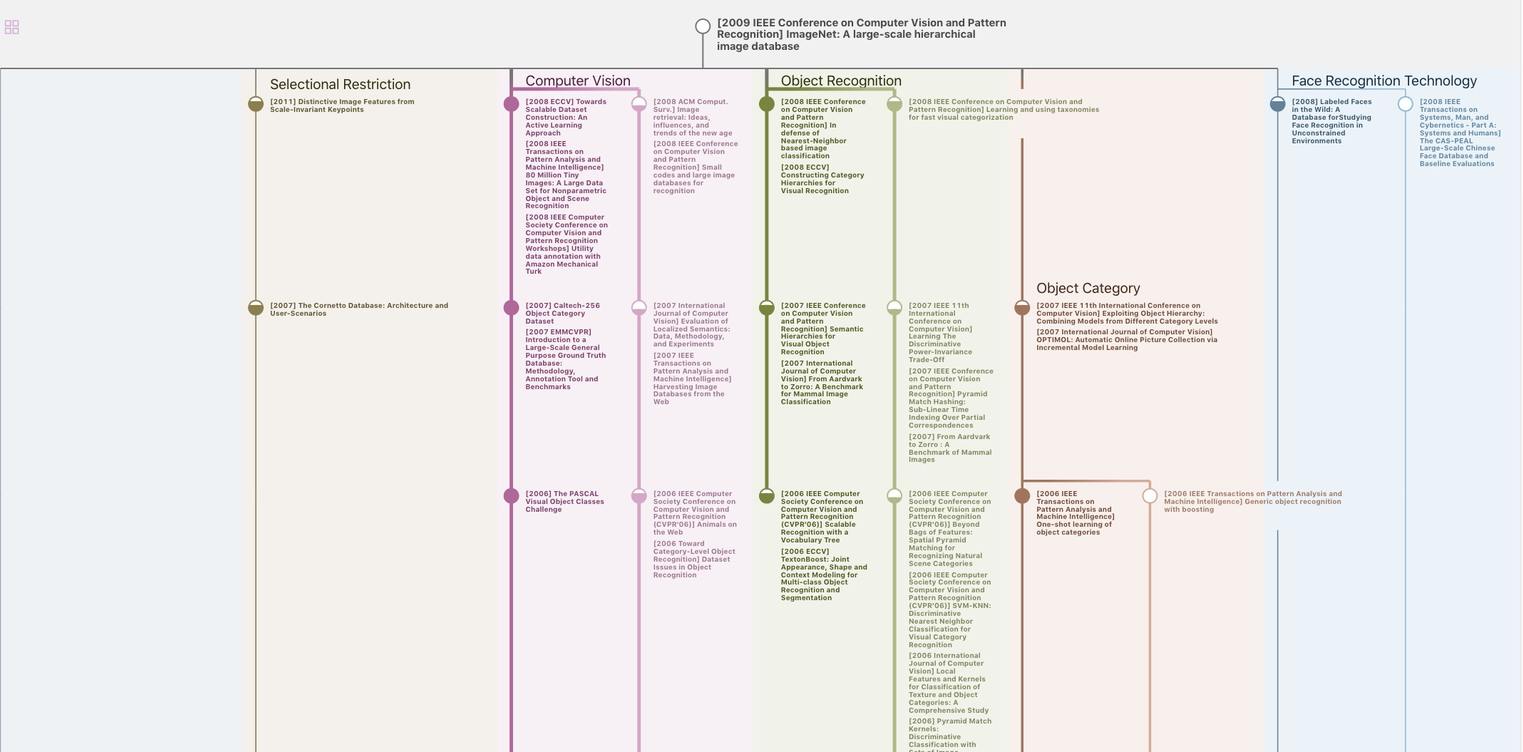
生成溯源树,研究论文发展脉络
Chat Paper
正在生成论文摘要