Parameter-state estimation for mechanical systems with small model errors
IFAC PAPERSONLINE(2023)
摘要
The performance of algorithms for parameter-state estimation, often expressed within a Bayesian filtering context, can be hindered by the need to recompute the parametric system matrices at each parameter iteration step. This work aims to alleviate the associated computational burden by expressing model errors that stem from parametric uncertainties, as additive parametric terms in the state and observation equations. For this purpose errors in the eigenstructure of a parametrized mechanical system are propagated to the physical parameters and eventually modelled as additive terms by means of perturbation analysis. A state observer is derived under the assumption that the change between the current and a true model parameter is deterministic and known. An estimate of the possible parameter discrepancy is obtained by minimizing the value of a change detection test applied on a Kalman filter innovation sequence.
更多查看译文
关键词
Parameter estimation,change detection,mechanical vibrations,Bayesian filtering.
AI 理解论文
溯源树
样例
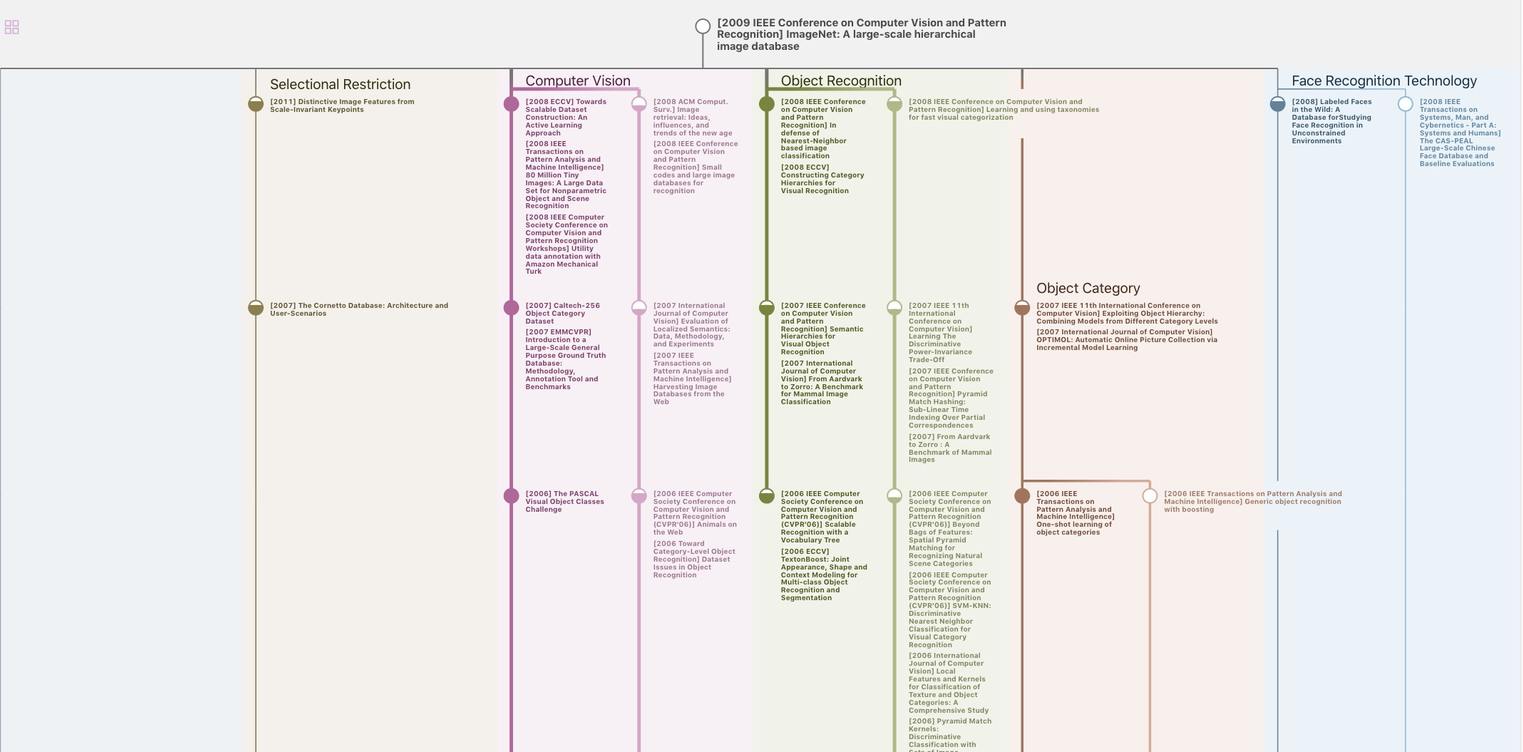
生成溯源树,研究论文发展脉络
Chat Paper
正在生成论文摘要