Conflict Forecasting Using Remote Sensing Data: an Application to the Syrian Civil War
INTERNATIONAL JOURNAL OF FORECASTING(2024)
Inst Stat | Ludwig Maximilians Univ Munchen | Univ Bath | Tech Univ Munich
Abstract
Conflict research is increasingly influenced by modern computational and statistical techniques. Combined with recent advances in the collection and public availability of new data sources, this allows for more accurate forecasting models in ever more fine-grained spatial areas. This paper demonstrates the utilization of remote sensing data as a potential solution to the lack of official data sources for conflict forecasting in crisis-ridden countries. We evaluate and quantify remote sensing data’s differentiated impact on forecasting accuracy across fine-grained spatial grid cells using the Syrian civil war as a use case. It can be shown that conflict, particularly its onset, can be forecasted more accurately by employing publicly available remote sensing datasets. These results are consistent across a range of established statistical and machine learning models, which raises the hope to get closer to reliable early-warning systems for conflict prediction.
MoreTranslated text
Key words
Remote sensing,Satellite imagery,Conflict prediction,Forecasting,Machine learning,Statistical modeling,Syria
求助PDF
上传PDF
View via Publisher
AI Read Science
AI Summary
AI Summary is the key point extracted automatically understanding the full text of the paper, including the background, methods, results, conclusions, icons and other key content, so that you can get the outline of the paper at a glance.
Example
Background
Key content
Introduction
Methods
Results
Related work
Fund
Key content
- Pretraining has recently greatly promoted the development of natural language processing (NLP)
- We show that M6 outperforms the baselines in multimodal downstream tasks, and the large M6 with 10 parameters can reach a better performance
- We propose a method called M6 that is able to process information of multiple modalities and perform both single-modal and cross-modal understanding and generation
- The model is scaled to large model with 10 billion parameters with sophisticated deployment, and the 10 -parameter M6-large is the largest pretrained model in Chinese
- Experimental results show that our proposed M6 outperforms the baseline in a number of downstream tasks concerning both single modality and multiple modalities We will continue the pretraining of extremely large models by increasing data to explore the limit of its performance
Upload PDF to Generate Summary
Must-Reading Tree
Example
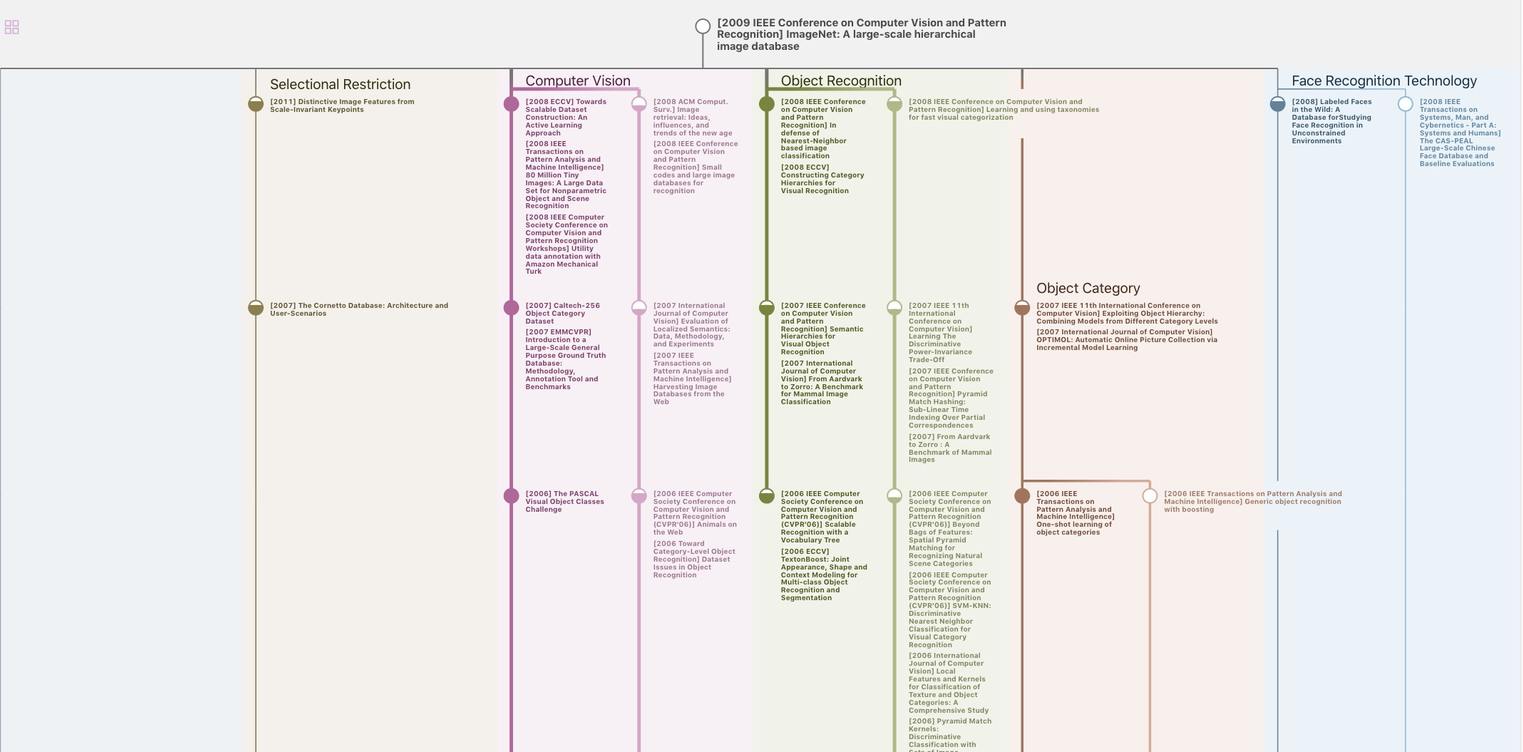
Generate MRT to find the research sequence of this paper
Related Papers
Data Disclaimer
The page data are from open Internet sources, cooperative publishers and automatic analysis results through AI technology. We do not make any commitments and guarantees for the validity, accuracy, correctness, reliability, completeness and timeliness of the page data. If you have any questions, please contact us by email: report@aminer.cn
Chat Paper