Data-driven friction force prediction model for hydraulic actuators using deep neural networks
MECHANISM AND MACHINE THEORY(2024)
摘要
Hydraulic actuators convert fluid pressure into mechanical motion. They are widely used in many industrial and aerospace applications due to their reliability, high speed, high force output, smooth operation, and shock compensation ability. Because of their importance and wide adoption, it is vital to enable precise modeling of such devices. Fortunately, various modeling methods exist for hydraulic actuators and hydraulically driven systems, ranging from lookup tables or simple equations reflecting the system's main features using lumped fluid theory to sophisticated and realistic fluid dynamics models. However, accurately accounting for friction that can depend nonlinearly on several state variables remains a core challenge in achieving high-fidelity hydraulic modeling. Therefore, several computational friction models are available, and their parameters must be identified or guessed. Another concern refers to simulation efficiency when complex models are considered. This study introduces a data -driven surrogate based on deep neural networks to address the challenge of practical and effective modeling of friction in hydraulic actuators. The surrogate is trained as a predictor using synthetic data generated from LuGre friction, demonstrating excellent accuracy and efficiency.
更多查看译文
关键词
Hydraulic actuator,Friction force modeling,Data-driven surrogates,Deep neural networks
AI 理解论文
溯源树
样例
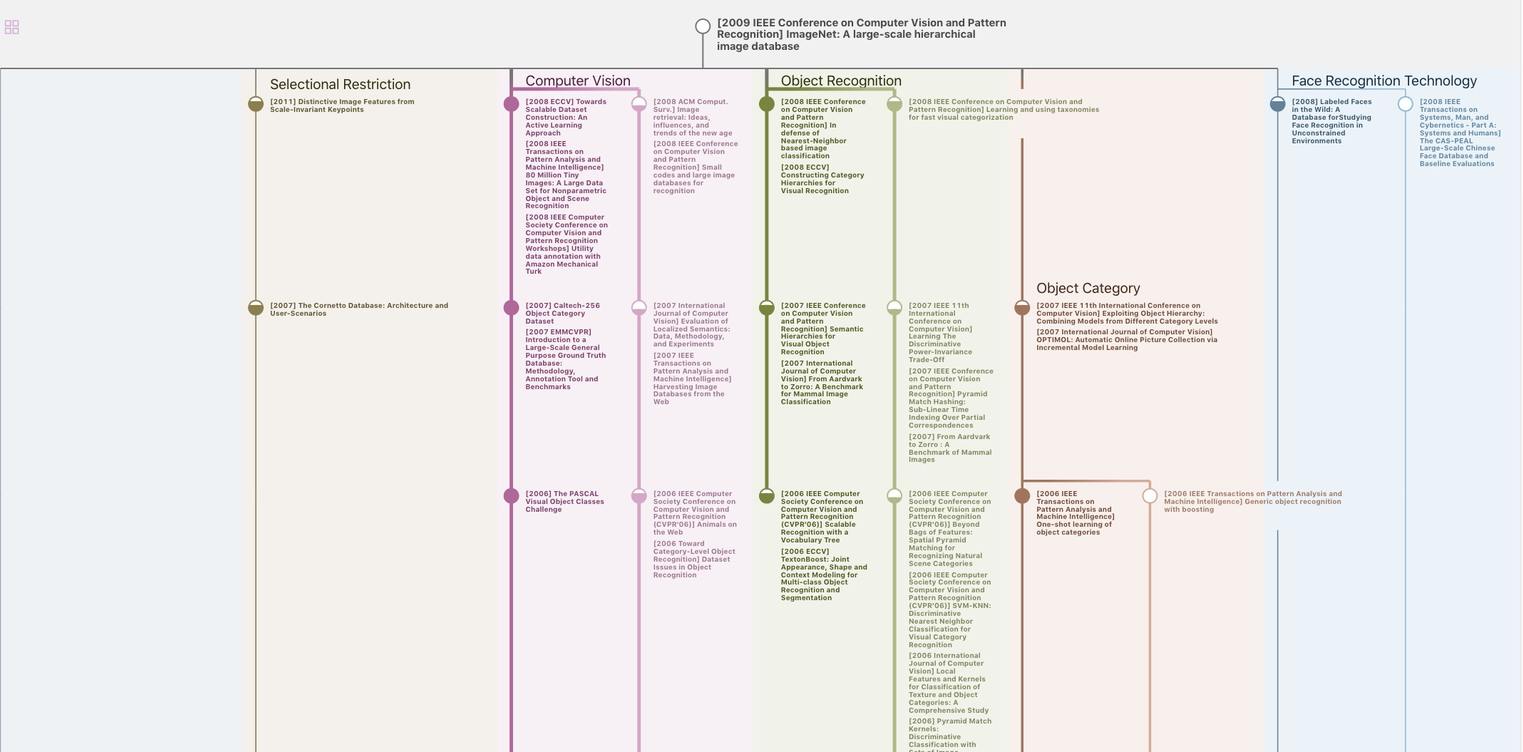
生成溯源树,研究论文发展脉络
Chat Paper
正在生成论文摘要