Predicting anti-SARS-CoV-2 activities of chemical compounds using machine learning models
Artificial Intelligence Chemistry(2023)
摘要
To accelerate the discovery of novel drug candidates for Coronavirus Disease 2019 (COVID-19) therapeutics, we reported a series of machine learning (ML)-based models to accurately predict the anti-SARS-CoV-2 activities of screening compounds. We explored 6 popular ML algorithms in combination with 15 molecular descriptors for molecular structures from 9 screening assays in the COVID-19 OpenData Portal hosted by NCATS. As a result, the models constructed by k-nearest neighbors (KNN) using the molecular descriptor GAFF+RDKit achieved the best overall performance with the highest average accuracy of 0.68 and relatively high average area under the receiver operating characteristic curve of 0.74, better than other ML algorithms. Meanwhile, The KNN model for all assays using GAFF+RDKit descriptor outperformed using other descriptors. The overall performance of our developed models was better than REDIAL-2020 (R). A web server (https://clickff.org/amberweb/covid-19-cp) was developed to enable users to predict anti-SARS-CoV-2 activities of arbitrary compounds using the COVID-19-CP (P) models. Besides the descriptor-based machine learning models, we also developed graph-based Attentive FP (A) models for the 9 assays. We found that the Attentive FP models achieved a comparable performance to that of COVID-19-CP and outperformed the REDIAL-2020 models. The consensus prediction utilizing both COVID-19-CP and Attentive FP can significantly boost the prediction accuracy as assessed by comparing its performance with other three individual models (R, P, A) utilizing the Wilcoxon signed-rank test, thus can ultimately improve the success rate of COVID-19 drug discovery.
更多查看译文
关键词
COVID-19,Machine Learning,In Silico Screening,Antiviral Compounds,SHAP Analysis,Attentive FP
AI 理解论文
溯源树
样例
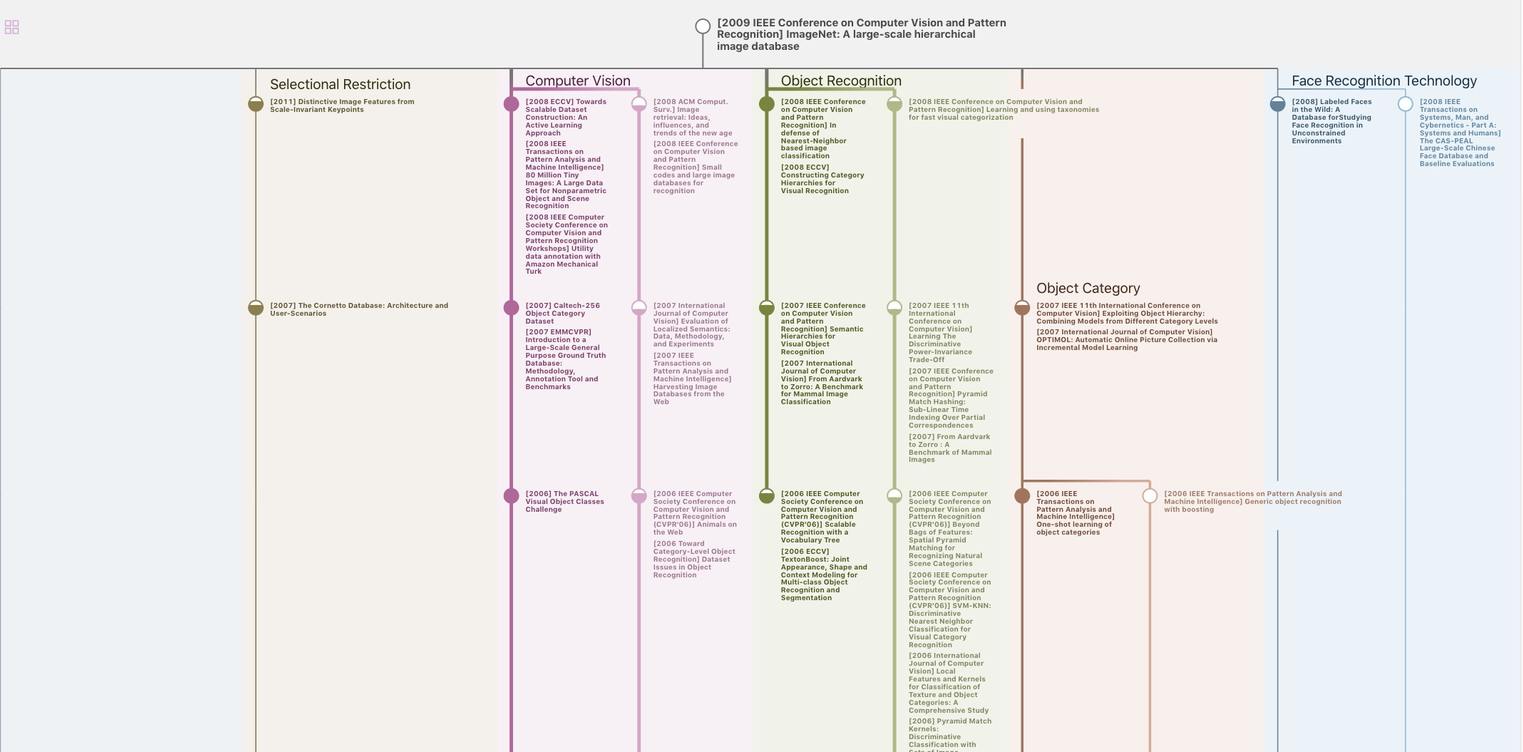
生成溯源树,研究论文发展脉络
Chat Paper
正在生成论文摘要