Optimized synthetic data and semi-supervised learning for Derived Cetane Number prediction
COMBUSTION AND FLAME(2024)
摘要
Derived Cetane Number (DCN) serves as a critical indicator for assessing the ignition quality of fuels in diesel engines. Training generalized regression models for DCN is challenging due to limited data availability. In this study, we propose a novel semi-supervised approach that combines real hydrocarbon mixtures and synthetically generated mixtures to overcome this data scarcity obstacle. The synthetic mixtures are generated using a Sequential Least Squares Programming (SLSQP) optimization method, targeting comprehensive coverage of UNIFAC chemical functional group compositions. By utilizing a dataset of real and synthetic mixtures, an Artificial Neural Network (ANN) model is trained based on the UNIFAC chemical functional group composition of fuels to improve DCN prediction accuracy and model reliability compared to models trained solely on real data. The improved model achieves excellent performance on the training and testing datasets, as indicated by high R2 Score, Mean Square Error (MSE), and Mean Absolute Error (MAE) values. Moreover, the model dem-onstrates accurate predictions on ten real fuels and their eighteen mixtures, with 100 % of samples falling within +/- 10 % of the measured DCN by an Ignition Quality Tester (IQT). The results demonstrate the potential of augmenting real data using appropriate data generation techniques to improve the representativeness and predictive capabilities of models in the presence of limited experimental data.
更多查看译文
关键词
Chemical functional groups,Ignition quality tester,Hydrocarbon fuels,Optimization,Neural networks
AI 理解论文
溯源树
样例
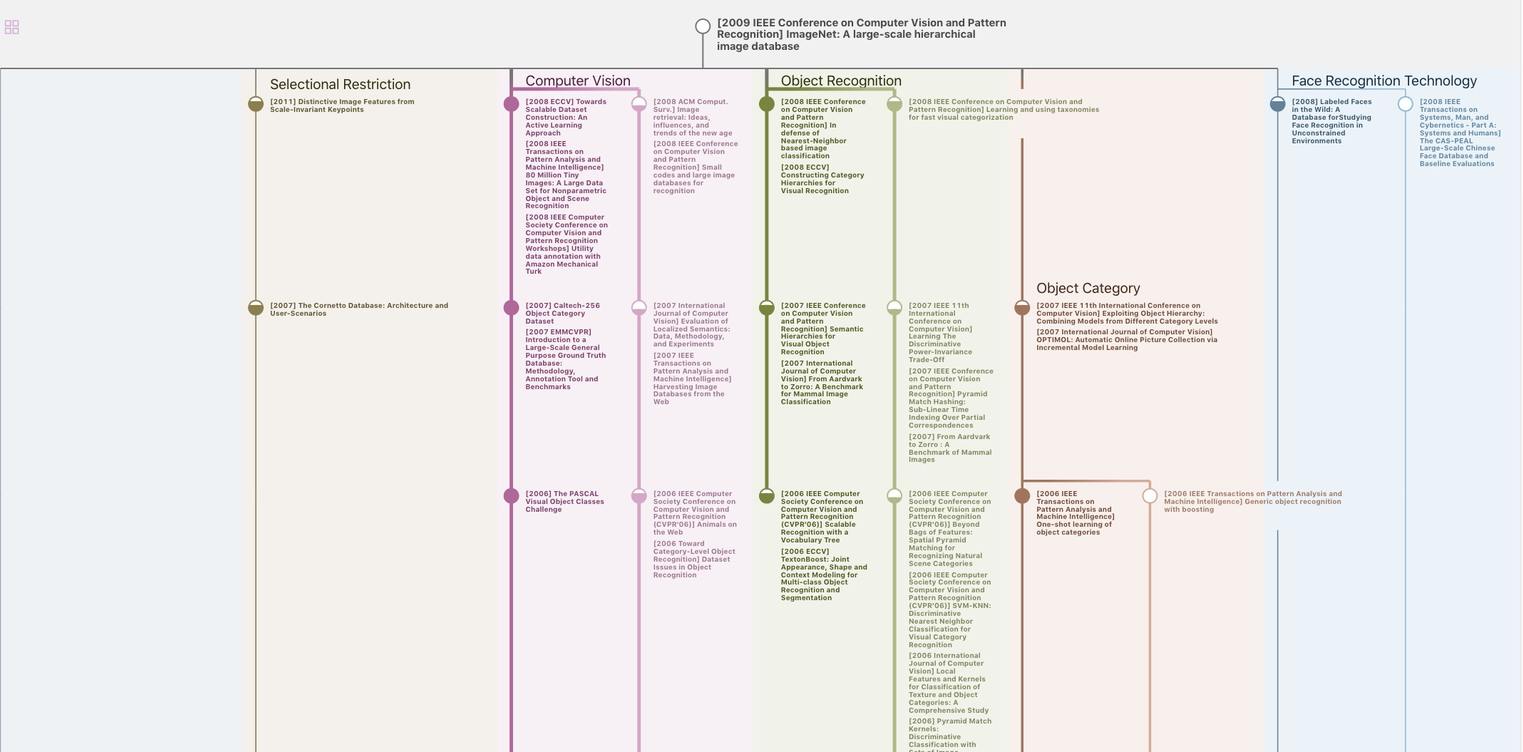
生成溯源树,研究论文发展脉络
Chat Paper
正在生成论文摘要