Query-focused summarization with the context-graph information fusion transformer
EXPERT SYSTEMS WITH APPLICATIONS(2024)
摘要
Query-Focused Summarization (QFS) is a system that understands important information from a long document and generates them as a summary that can responds to the query. In QFS, how to properly utilize query information to generate a summary is a challenging problem. Existing Transformer-based QFS models, which is attending all words in the concatenation of a query and a document, result in inaccurate concentration on some unimportant words and they consequently cannot generate a good query-focused summary. This study proposes a Query-attentive Semantic Graph (QSG) that assists in identifying words related to the query, and a novel QFS model that generates a query-focused summary by appropriately fusing the contextual information of the language model with the structural information of QSG. In addition, we propose a novel personalized PageRank based graph neural network that computes each node's importance score for the query inside QSG and utilizes it for node representation calculation. Experimental results on two QFS benchmarks show that the performance of the proposed model outperforms the simple Transformer-based model by large margins, as well as other state-of-the-art QFS.
更多查看译文
关键词
Query-focused summarization,Graph neural networks,Graph-based method
AI 理解论文
溯源树
样例
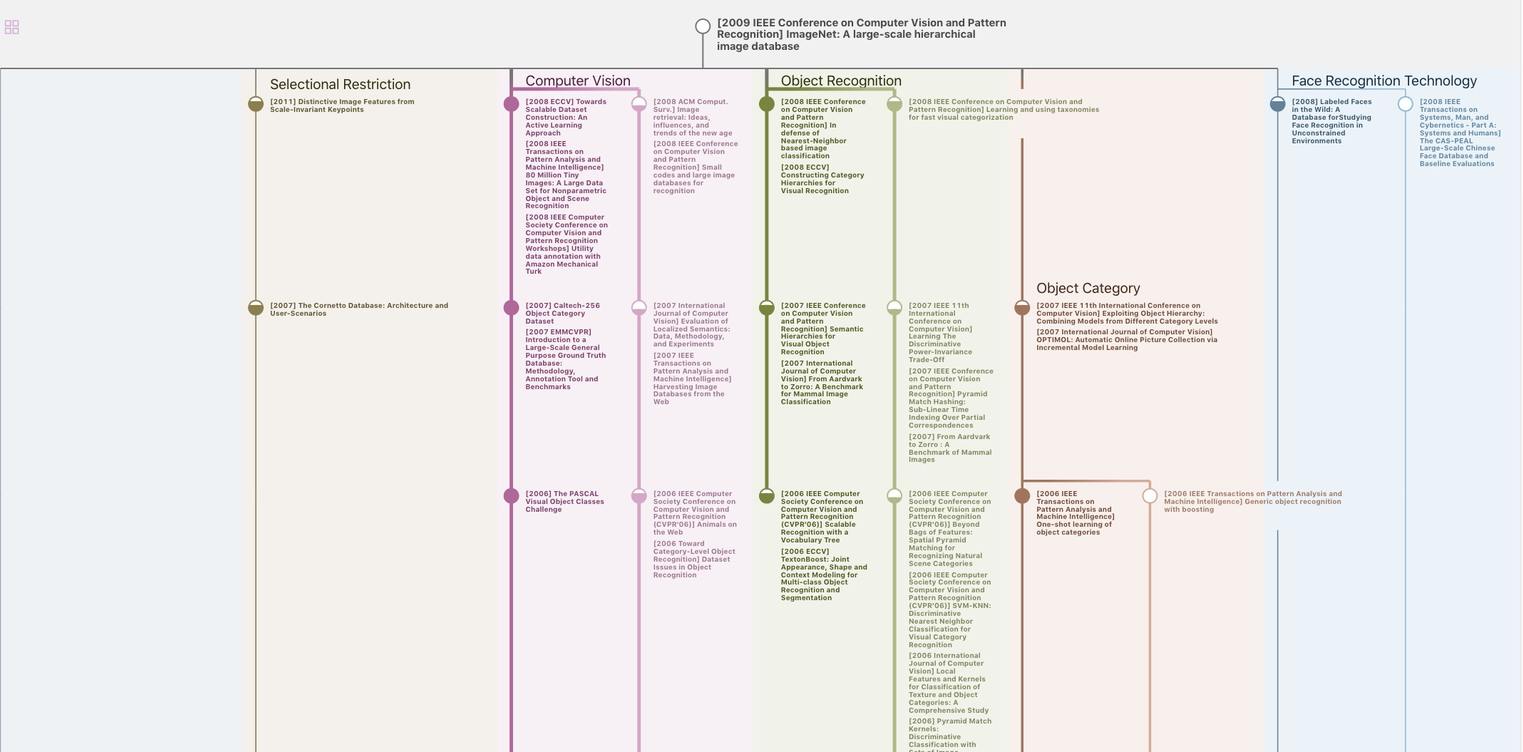
生成溯源树,研究论文发展脉络
Chat Paper
正在生成论文摘要