A deep learning-based approach for predicting oil production: A case study in the United States
ENERGY(2024)
摘要
The accuracy of oil production predictions is crucial in the field of petroleum engineering. However, due to the time series characteristics of oil production and the complex relationship among multiple influencing factors, traditional methods, and time series prediction techniques have limitations in fully extracting time series features and exploring the internal relationships between variables. Deep learning techniques possess unique advantages in solving nonlinear problems and time-series problems but require a significant amount of data and can only conduct short-term oil production predictions due to the nonlinear and chaotic nature of yield. Therefore, this work aims to establish a model that can overcome these limitations. A modified GRU (M-GRU) is proposed to extract time series features of variables and external economic information. Then, the autoregressive (AR) model is integrated into importing priori knowledge to enhance the model's capability in extracting time series in-formation. In addition, feature mapping is used to improve convergence performance. Finally, A loss function that can dynamically adjust the weights is proposed, which can enhance the model's ability to fit from error-prone samples. By validating the proposed model using oil production data, it has been proven that the model can predict oil production accurately and outperform other models with a correlation coefficient reaching 0.99874. Further tests show that the model can provide accurate prediction results even with limited sample sizes and during coincidental events such as financial crises and COVID-19 epidemics, providing strong support for decision-making for reservoir engineers.
更多查看译文
关键词
Oil production prediction,Feature fusion,Feature mapping,Modified GRU,Autoregressive model
AI 理解论文
溯源树
样例
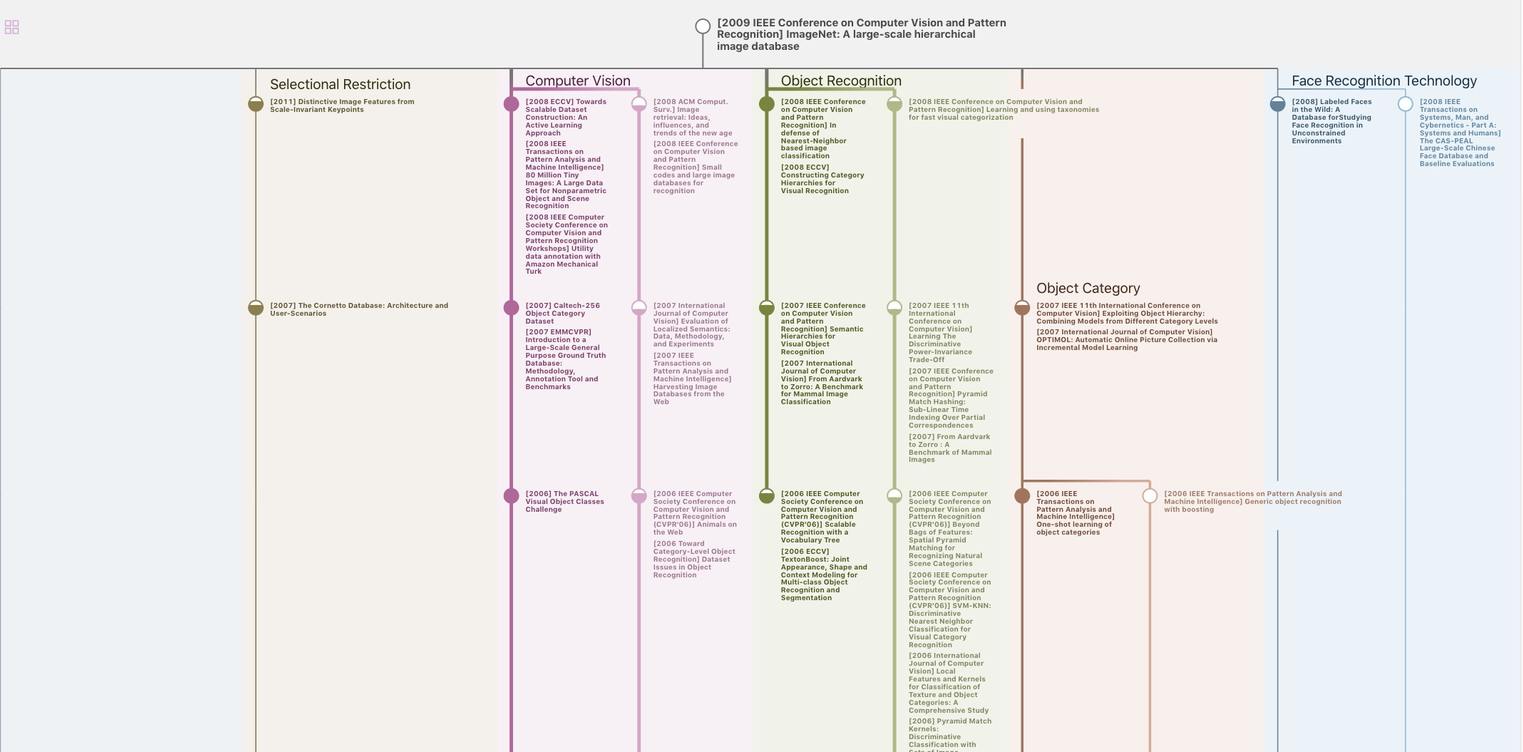
生成溯源树,研究论文发展脉络
Chat Paper
正在生成论文摘要