Nonmonotone variable projection algorithms for matrix decomposition with missing data
PATTERN RECOGNITION(2024)
摘要
This paper investigates algorithms for matrix factorization when some or many components are missing, a problem that arises frequently in computer vision and pattern recognition. We demonstrate that the Jacobian used in the damped Wiberg (DW) method is exactly the same as that of Kaufman's simplified variable projection (VP) algorithm. Our analysis provides a novel perspective on the efficiency of VP algorithms by improving the strong convexity of the approximate function. To enhance numerical stability, we set a lower bound on the damping parameter instead of adding a null space like the DW algorithm. Another challenge of low-rank matrix decomposition with missing data is the existence of many sharp local minima, which are often distributed in narrow valleys of the landscape of objection functions. Falling into such minima tends to result in poor reconstruction results. To address this issue, we design a non-monotonic VP algorithm, which can facilitate the algorithm to escape from sharp minima and converge to flatter minima. Numerical experiments confirm the effectiveness and efficiency of the proposed nonmonotone VP algorithm.
更多查看译文
关键词
Low-rank matrix factorization,Missing data,Damped wiberg method,Variable projection
AI 理解论文
溯源树
样例
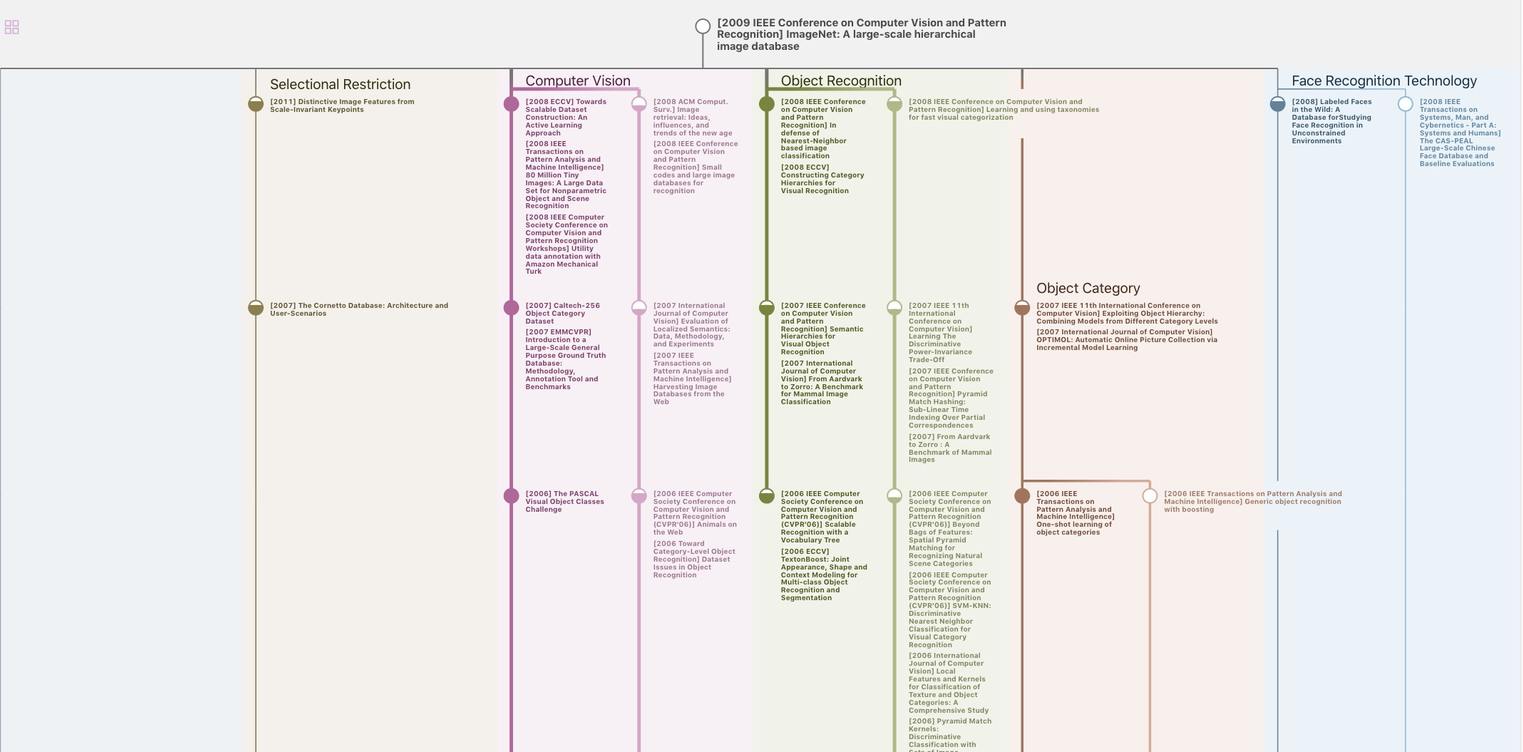
生成溯源树,研究论文发展脉络
Chat Paper
正在生成论文摘要