Testing homogeneity in high dimensional data through random projections
JOURNAL OF MULTIVARIATE ANALYSIS(2024)
摘要
Testing for homogeneity of two random vectors is a fundamental problem in statistics. In the past two decades, numerous efforts have been made to detect heterogeneity when the random vectors are multivariate or even high dimensional. Due to the "curse of dimensionality", existing tests based on Euclidean distance may fail to capture the overall homogeneity in high dimensional settings while can only capture the moment discrepancy. To address this issue, we propose a fully nonparametric test for homogeneity of two random vectors. Our method involves randomly selecting two subspaces consisting of components of the vectors, projecting the subspaces onto one-dimensional spaces, respectively, and constructing the test statistic using the Cramer-von Mises distance of the projections. To enhance the performance, we repeatedly implement this procedure to construct the final test statistic. Theoretically, if the replication time tends to infinity, we can avoid potential power loss caused by lousy directions. Owing to the U-statistic theory, the asymptotic null distribution of our proposed test is standard normal, regardless of the parent distributions of the random samples and the relationship between data dimensions and sample sizes. As a result, no re-sampling procedure is needed to determine critical values. The empirical size and power of the proposed test are demonstrated through numerical simulations.
更多查看译文
关键词
Cramer-von Mises test,High dimension,High Random projections,Two-sample test
AI 理解论文
溯源树
样例
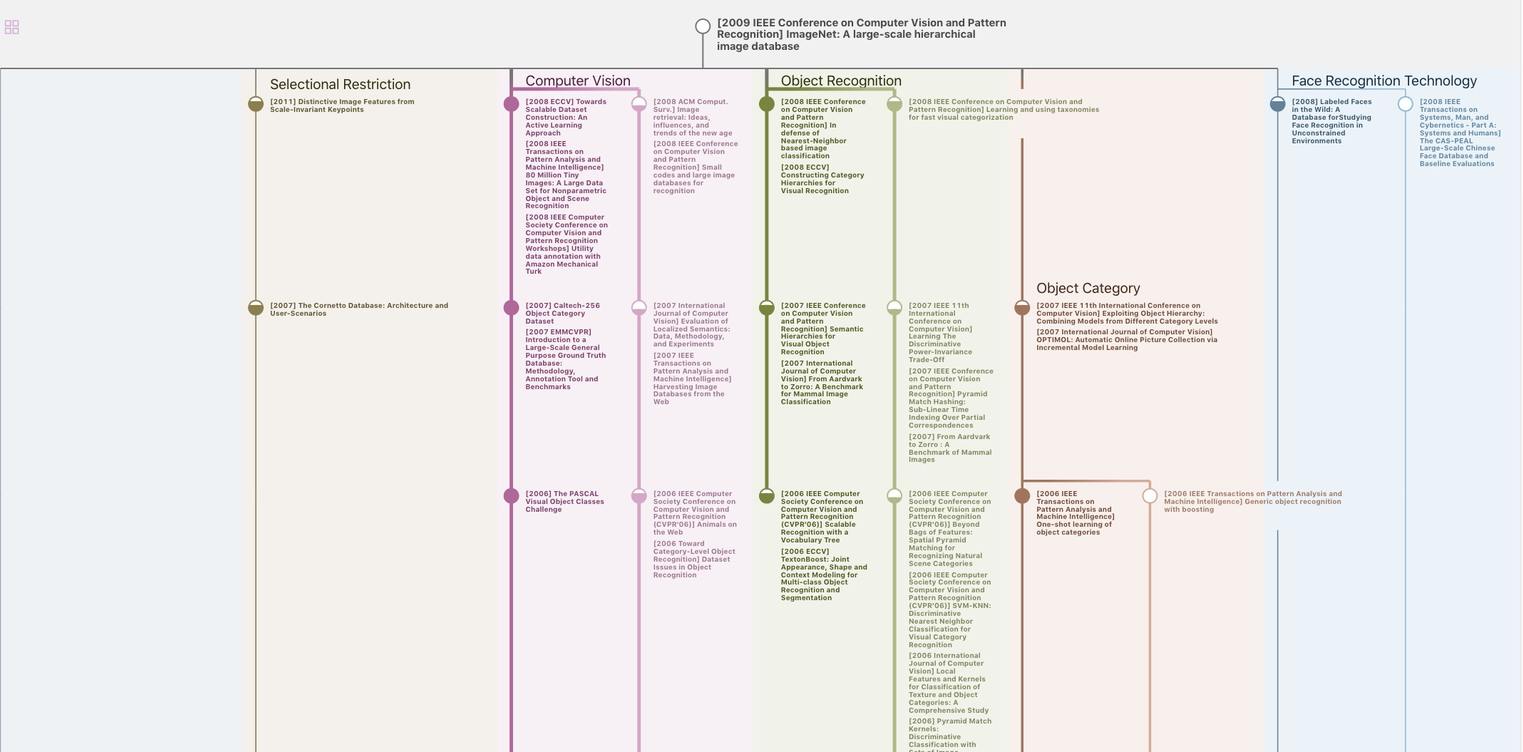
生成溯源树,研究论文发展脉络
Chat Paper
正在生成论文摘要