Transferrable contextual feature clusters for parking occupancy prediction
PERVASIVE AND MOBILE COMPUTING(2024)
摘要
Recently, real-time parking availability prediction has attracted much attention since the rapid development of sensor technologies and urbanisation. Most existing works have applied various models to predict long and short-term parking occupancy using historical records. However, historical records are not available for many real-world scenarios, such as new urban areas, where parking lots are fast adjusted and extended. In this paper, we aim to predict parking occupancy using historical data in other areas and contextual information within the targeted area that lacks historical data. We propose a two-step framework to first learn the important contextual features from areas where parking records already existed. Then we transfer these features to the other new areas without historical data records. Through conducting a real-world dataset with various clustering methods combined with different regression models, we observe that multiple contextual features are likely to influence parking availability prediction. We find the best combination (i.e., k-shape clustering algorithm and LSTM regression model) to build parking occupancy prediction model based on the subsequent quantitative correlation analysis between contextual features and parking occupancy. The experimental results show that (1) the conventional internal clustering evaluation does not work well for spatio-temporal data clustering for the prediction purpose; (2) our proposed approach achieves approximately 3% error rate in 30 minutes of prediction, which is significantly better than the estimation of the occupancy rate using the rate in the adjacent regions (13.3%).
更多查看译文
关键词
Parking occupancy prediction,Spatio-temporal data mining
AI 理解论文
溯源树
样例
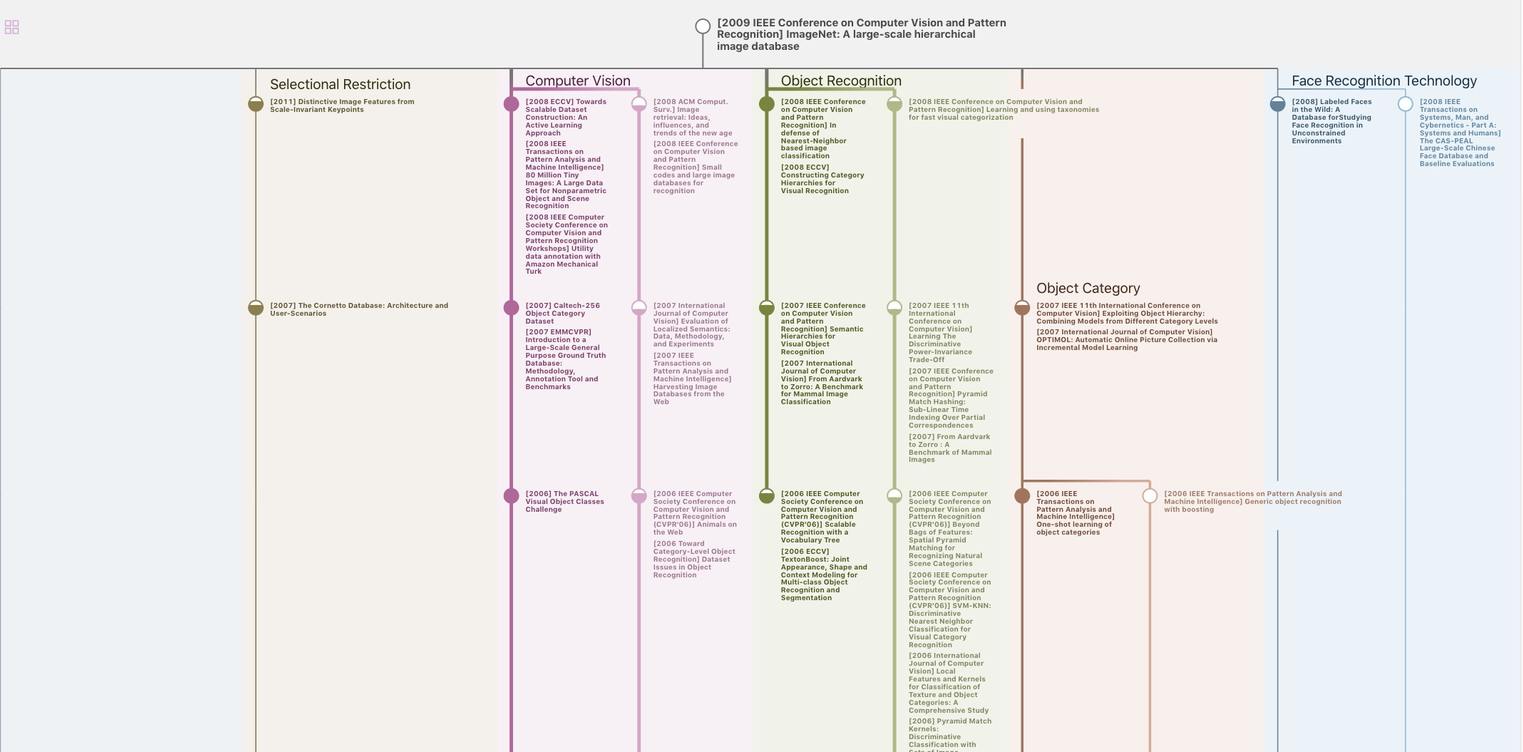
生成溯源树,研究论文发展脉络
Chat Paper
正在生成论文摘要