Evaluating the choice of radial basis functions in multiobjective optimal control applications
ENVIRONMENTAL MODELLING & SOFTWARE(2024)
摘要
Evolutionary Multi-Objective Direct Policy Search (EMODPS) is a prominent framework for designing control policies in multi-purpose environmental systems, combining direct policy search with multi-objective evolu-tionary algorithms (MOEAs) to identify Pareto approximate control policies. While EMODPS is effective, the choice of functions within its global approximator networks remains underexplored, despite their potential to significantly influence both solution quality and MOEA performance. This study conducts a rigorous assessment of a suite of Radial Basis Functions (RBFs) as candidates for these networks. We critically evaluate their ability to map system states to control actions, and assess their influence on Pareto efficient control policies. We apply this analysis to two contrasting case studies: the Conowingo Reservoir System, which balances competing water demands including hydropower, environmental flows, urban supply, power plant cooling, and recreation; and The Shallow Lake Problem, where a city navigates the trade-off between environmental and economic objectives when releasing anthropogenic phosphorus. Our findings reveal that the choice of RBF functions substantially impacts model outcomes. In complex scenarios like multi-objective reservoir control, this choice is critical, while in simpler contexts, such as the Shallow Lake Problem, the influence is less pronounced, though distinctive differences emerge in the characteristics of the prescribed control strategies.
更多查看译文
关键词
Direct policy search,Many Objective Evolutionary Algorithms,Water resources management,Global approximators
AI 理解论文
溯源树
样例
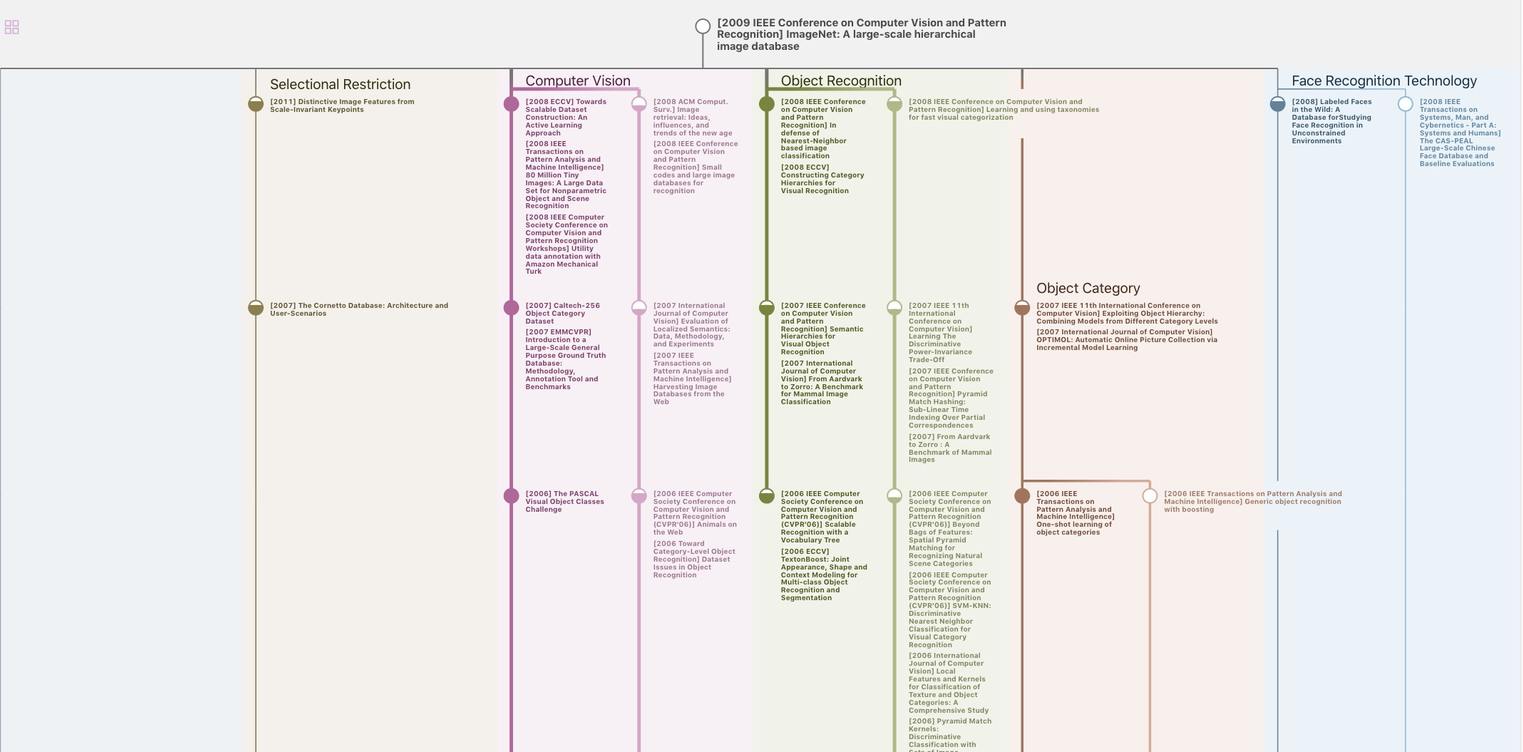
生成溯源树,研究论文发展脉络
Chat Paper
正在生成论文摘要