A reduced-order machine-learning-based method for fault recognition in tool condition monitoring
MEASUREMENT(2024)
摘要
The application of Machine Learning methodologies has been particularly noteworthy and abundant in pattern and symptom recognition across various research areas. However, Tool Condition Monitoring remains a chal-lenging subject due to the gradual wearing out of cutting tools during the machining process. Such failure leads to reduced accuracy and quality of the machined surface of the workpiece, resulting in increased costs. This research proposes an innovative ML-based method to clarify failure symptoms of cutting tools in the frequency and time-frequency domains. The study involves five cutting tools as experimental case studies during a 200 -minute machining operation. The results are validated using the Fast Fourier Transform, Short-time Fourier Transform, Empirical Mode Decomposition, and Variational Mode Decomposition methods, to demonstrate that the suggested methodology better identifies failure symptoms compared to other mentioned methods. One advantage of the proposed method is that considering a lower order of the system results in clearer frequency and time-frequency domain diagrams without sacrificing accuracy.
更多查看译文
关键词
Tool condition monitoring,Machine learning,Joint time -frequency transform,Empirical mode decomposition,Variational mode decomposition
AI 理解论文
溯源树
样例
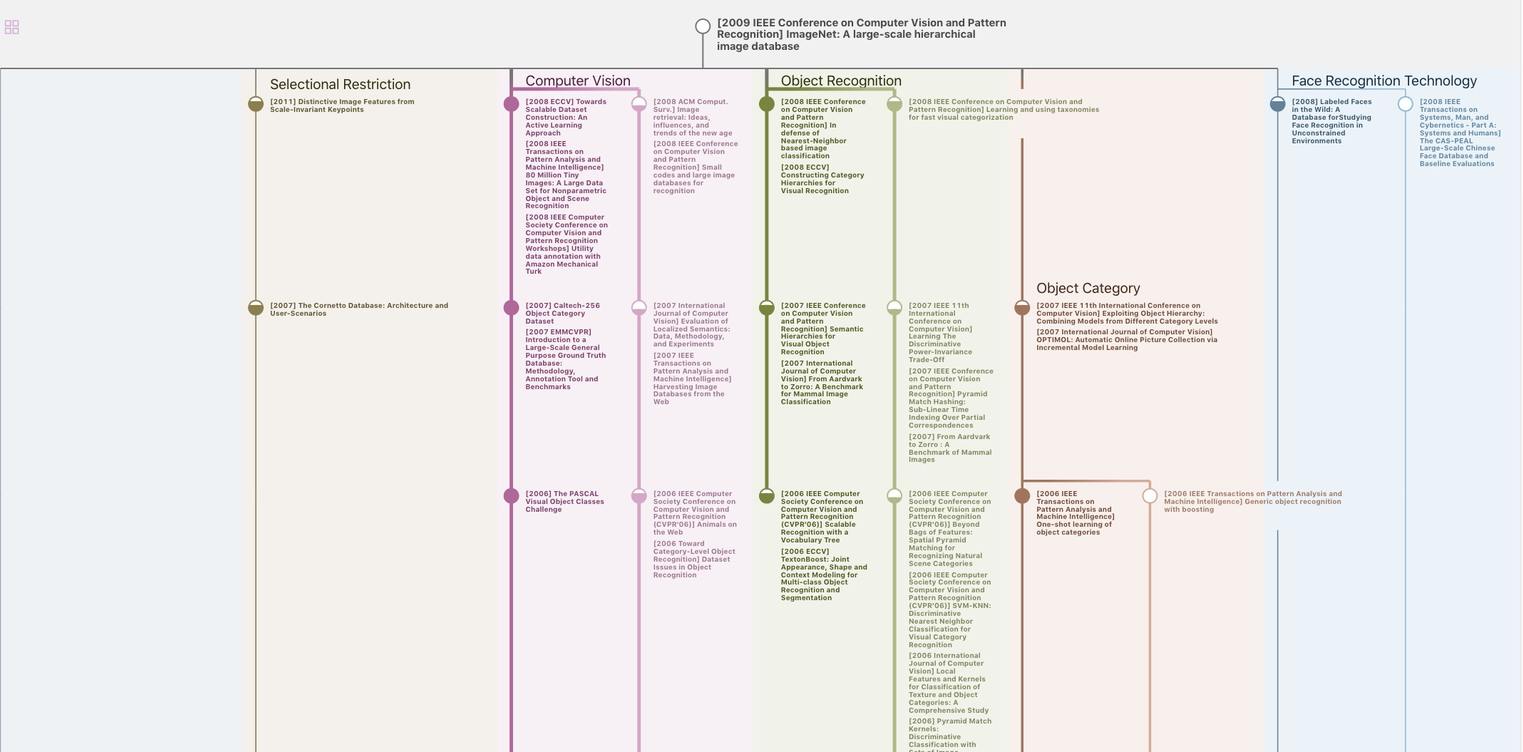
生成溯源树,研究论文发展脉络
Chat Paper
正在生成论文摘要