Machine learning approach for microbial growth kinetics analysis of acetic acid-producing bacteria isolated from organic waste
BIOCHEMICAL ENGINEERING JOURNAL(2024)
摘要
This study proposes novel hybrid methodology that combines machine learning (ML) techniques with experi-mental strategies to analyse microbial growth-kinetics of acetic acid-producing bacteria isolated from fruit waste. This work employs ML algorithms to create different models such as multivariate linear regression (MLR), partial least square regression (PLSR), Kernel ridge regression (KRR), support vector regression (SVR), Gradient boosting regression (GBR) that captures time-dependent patterns of bacterial growth dynamics. Experiments for microbial growth kinetic analysis were conducted on best isolate of acid producing bacteria with different glucose con-centrations (1-5 %) at predefined operating conditions. It is found significant growth rate (mu) was obtained at 4 % and 5 % concentration of glucose from experimental work. 0.0588 h-1 and 0.0571 h-1 are the specific growth rate obtained at 4 % and 5 % glucose concentration respectively. Proposed ML models employed to predict growth rate kinetics theoretically at varied glucose concentrations. Comparative results indicate that GBR model exhibits superior performance in predicting growth kinetics than other models. GBR model fits the experimental results approximately with lower RMSE (0.004) than other models. This enables more accurate representation of growth patterns that is difficult to discernible through conventional analytical methods. This approach will help to understand growth kinetics of acetic acid-producing bacteria for resource recovery, wastewater treatment, and bioremediation.
更多查看译文
关键词
Acetic acid-producing bacteria,Growth curve,Gradient boosting regression,Fruit waste,Kinetics
AI 理解论文
溯源树
样例
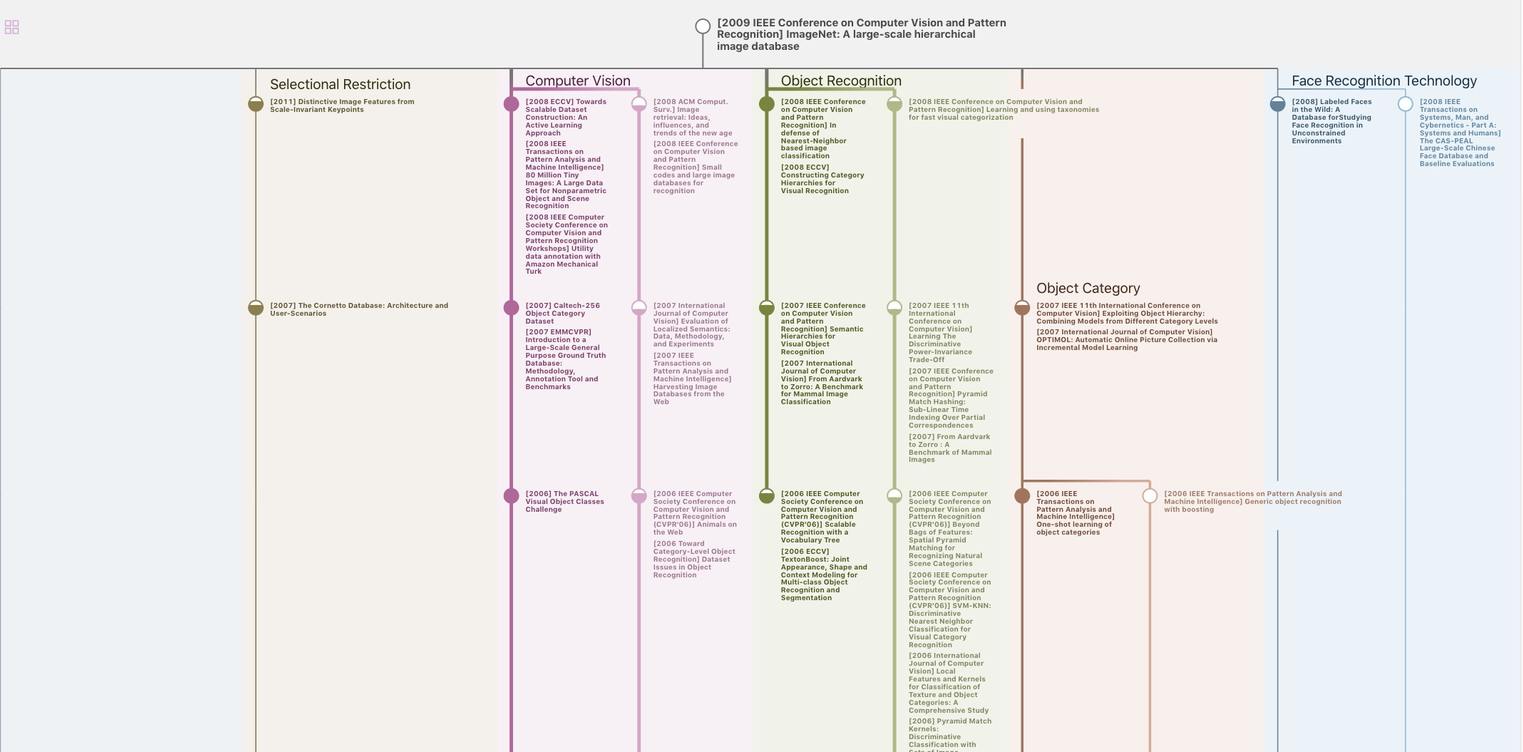
生成溯源树,研究论文发展脉络
Chat Paper
正在生成论文摘要