Network-wide short-term inflow prediction of the multi-traffic modes system: An adaptive multi-graph convolution and attention mechanism based multitask-learning model
TRANSPORTATION RESEARCH PART C-EMERGING TECHNOLOGIES(2024)
摘要
Network-wide short-term inflow prediction is important in efficiently managing the urban transportation system. Nowadays, all kinds of traffic modes gradually become interconnected and form a complex multi-traffic modes system, while extensive studies focus on the single-traffic mode and ignore the correlations among different traffic modes. There exist some challenges for short-term inflow prediction of multi-traffic modes: (1) the interaction mechanism among multi-traffic modes is difficult to learn and few studies explore the mechanism, (2) the data of multi-traffic modes are usually heterogenous due to the different spatial units of different traffic modes, and (3) it is challenging to extract the complex and dynamic features of the multi-traffic modes and most existing methods apply static spatiotemporal correlations among multi-traffic modes, while the genuine correlations among different traffic modes might be missing. To tackle these challenges, this study proposed a multitask-learning-based model called MultiModeformer (M2-former) with the encoder-decoder structure for network-wide short-term inflow prediction of the multi-traffic modes system. Specifically, the encoder is designed to learn and capture the complex and dynamic spatiotemporal correlations of multi-traffic modes, and the decoder is designed to extract the features of the target traffic mode and share knowledge among multi-traffic modes. Extensive experiments are conducted based on the real-world multi-traffic modes system data of Beijing, China. Results prove the superiority of the M2-former. In addition, the spatial and temporal information interaction mechanisms among multi-traffic modes are also explored. This paper can provide a reliable method and critical insights for the management and understanding of a multi-traffic modes system.
更多查看译文
关键词
Multi-traffic modes,Short-term passenger flow prediction,Multi-task learning,Transformer,Deep learning
AI 理解论文
溯源树
样例
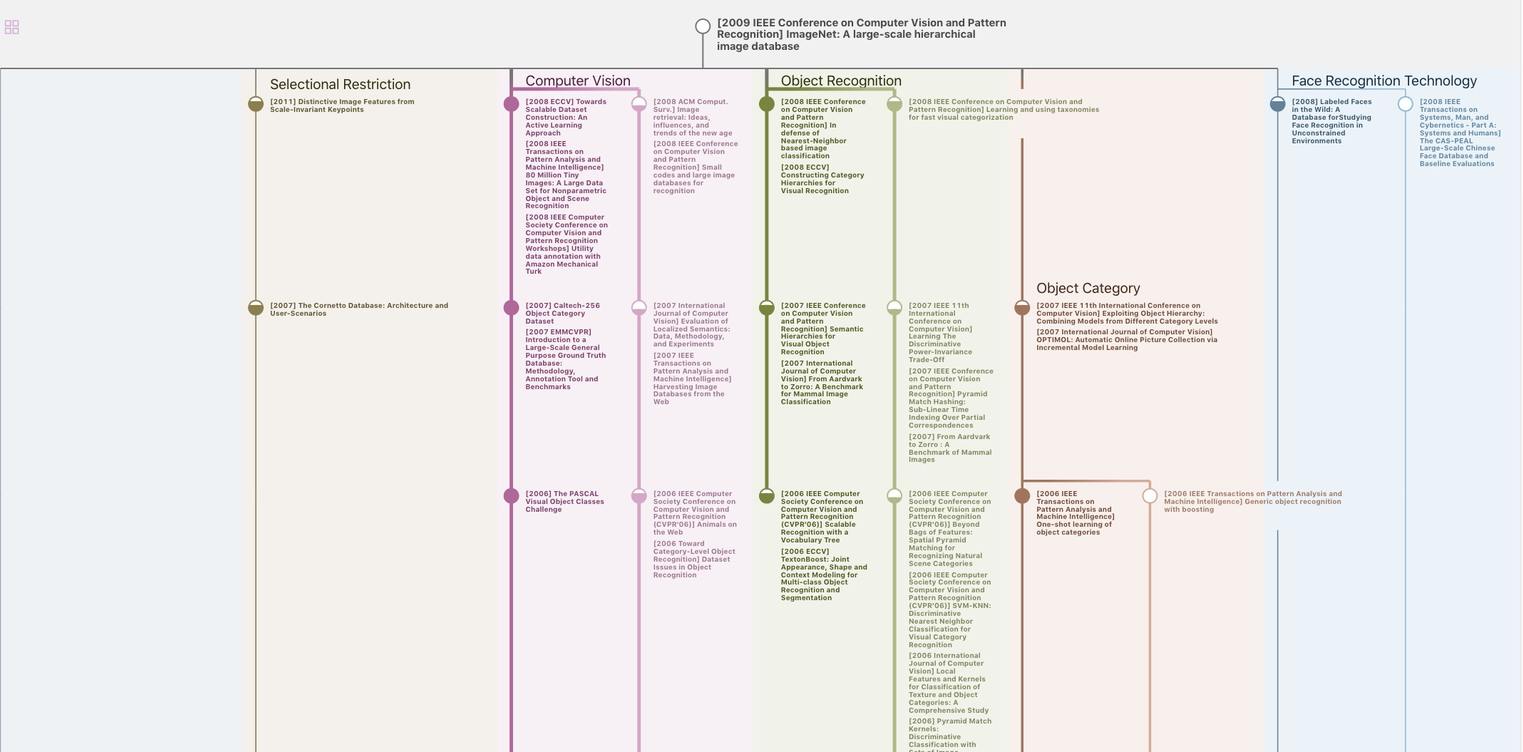
生成溯源树,研究论文发展脉络
Chat Paper
正在生成论文摘要