GOL-SFSTS based few-shot learning mechanical anomaly detection using multi-channel audio signal
KNOWLEDGE-BASED SYSTEMS(2024)
摘要
Anomaly detection has become an essential component of mechanical equipment preventive maintenance. When encountered in complicated industrial and military applications, audio signals can be captured in a non-contact manner, preserving significant engineering potential. Common systems that operate on single-channel raw sound inputs have limited anti-noise capabilities and few-shot learning (FSL) robustness. This study proposed a competitive FSL multi-channel anomaly detection framework that uses the global optimization layer-based spallation few-shot training strategy (GOL-SFSTS) on audio Mel-spectrogram samples. The transform on 8-channel audio Mel Spectrogram increases the model's feature capture ability for multi-channel signals greatly. A 'hotplug' discrepancy distance operator GOL is used to improve the model's anti-noise capacity when dealing with real-world operating situations. SFSTS is a growable learning approach designed to make the model more suitable for later classes and to increase FSL compatibility. We conduct ablation comparisons with other cuttingedge techniques, and FSL classification results on MIMII datasets show that our framework outperforms ResNet34 in full, 30-shot, 15-shot, and 5-shot by 9.6 %, 10.4 %, 9.8 %, and 5 %, respectively. The results of four trials indicate that the designed GOL-SFSTS framework is effective for anomaly detection applications. This paper's impressive achievements have opened up new opportunities for FSL multi-channel audio mechanical anomaly detection.
更多查看译文
关键词
Multichannel,Anomaly detection,Few -shot learning,Mel -spectrograms
AI 理解论文
溯源树
样例
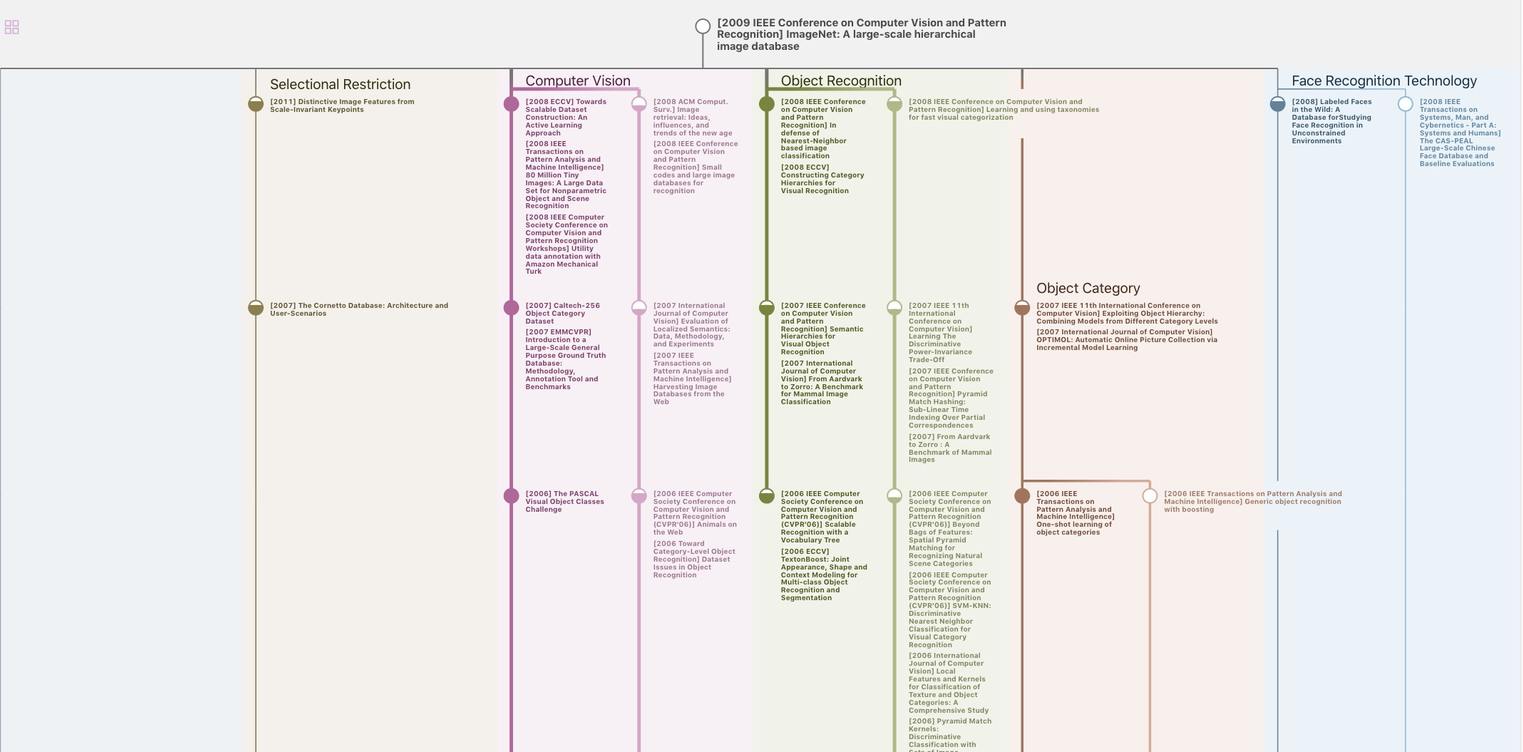
生成溯源树,研究论文发展脉络
Chat Paper
正在生成论文摘要