An adaptive classification and updating method for unknown network traffic in open environments
COMPUTER NETWORKS(2024)
摘要
In recent years, to address the classification problem of unknown traffic in open network environments, numerous deep learning (DL)-based classification techniques have been proposed. Among these techniques, the single threshold-based DL method has gained significant popularity. However, the classification boundary constructed by this method is inaccurate, thus leading to the misclassification of known and unknown traffic. In this paper, we propose an adaptive classification and updating method for accurate application-level classification of known and unknown traffic in open environments. We adaptively construct individualized and accurate decision boundaries for each class of known traffic, which avoids misclassification cases caused by a single threshold and achieves accurate separation of known and unknown traffic. To provide a more accurate feature representation for the construction of the decision boundary, we also introduce an additional angular loss function to guide the model in learning more accurate flow features. In addition, to cope with the constant emergence of new classes of traffic in real network environments, our proposed method adaptively updates the model to respond to changes in network traffic classes. The proposed method is evaluated on two datasets, and the experimental results show that our proposed method has excellent classification accuracy and outperforms the state-of-the-art unknown traffic classification methods.
更多查看译文
关键词
Network traffic classification,Unknown network traffic,Decision boundary,Multimodal features
AI 理解论文
溯源树
样例
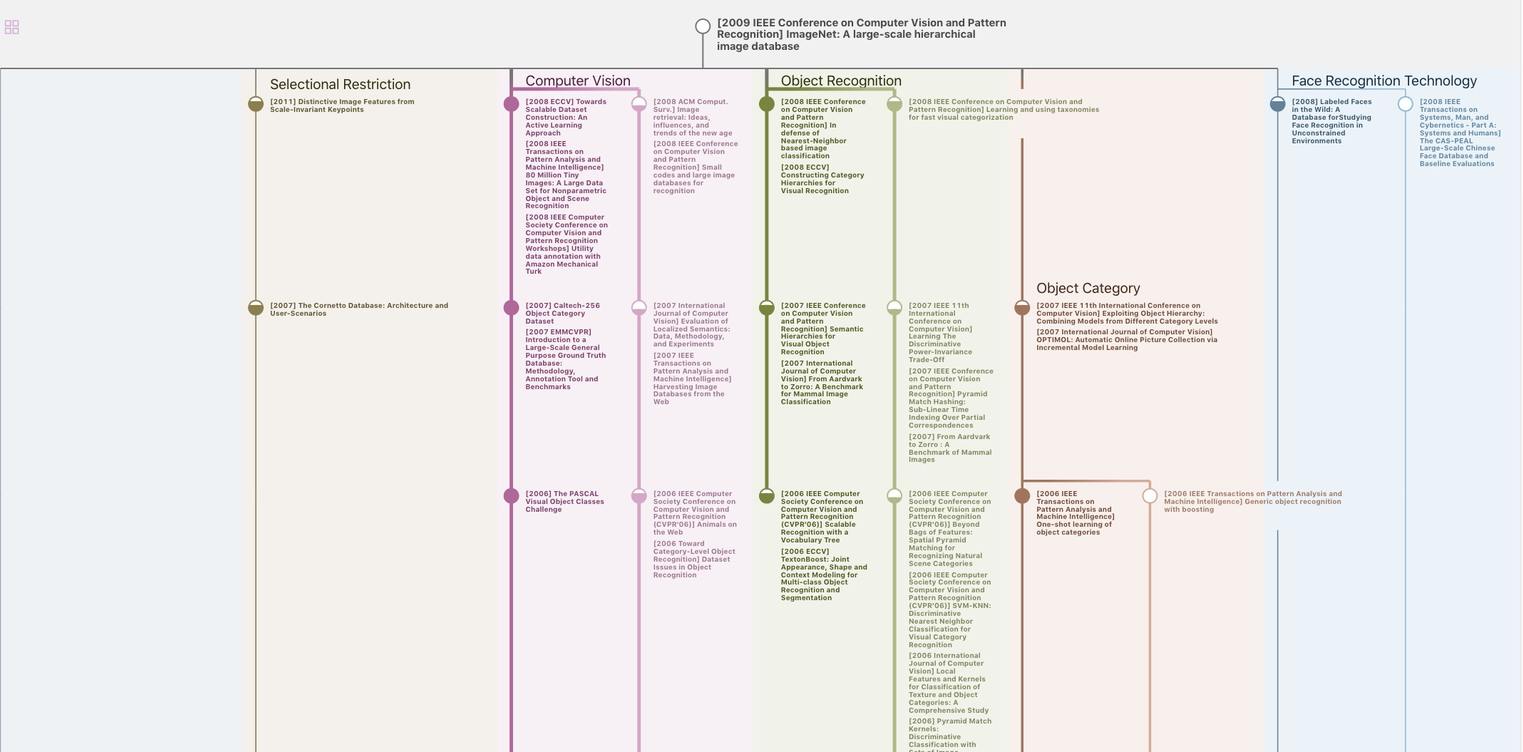
生成溯源树,研究论文发展脉络
Chat Paper
正在生成论文摘要