Enhancing Real-Time Nonintrusive Occupancy Estimation in Buildings Via Knowledge Fusion Network
Energy and buildings(2024)
摘要
Real-time nonintrusive occupancy estimation can maximize the use of existing sensors to infer occupant information in buildings with the advantages of fewer privacy concerns and fewer extra device costs. Recently, many deep learning architectures have proven effective in estimating occupancy directly from raw sensor data. However, some handcrafted features manually extracted from statistical and temporal domains might convey additional information for occupancy estimation. In this study, a novel knowledge fusion network for nonintrusive occupancy estimation is proposed to integrate knowledge from two streams, i.e. automatic knowledge stream from a deep learning architecture and handcrafted knowledge stream from manual feature engineering. Moreover, four different fusion modules are investigated to optimize the design of the fusion network. To verify the effectiveness of the proposed network, experiments are conducted in a dataset from the ASHRAE Global Occupant Behavior Database, which is collected from an office space with records of indoor environment parameters, occupant-building interactions, and contextual information. The results demonstrate the superiority of the proposed fusion network, which outperforms five representative algorithms. Furthermore, the ablation study underscores the benefits of knowledge fusion and occupant-building interaction information, showing that the proposed fusion network can enhance the occupancy estimation accuracy by 3.47 % to 9.24 %.
更多查看译文
关键词
Occupancy estimation,Nonintrusive sensing,Network fusion,Deep learning,Smart buildings
AI 理解论文
溯源树
样例
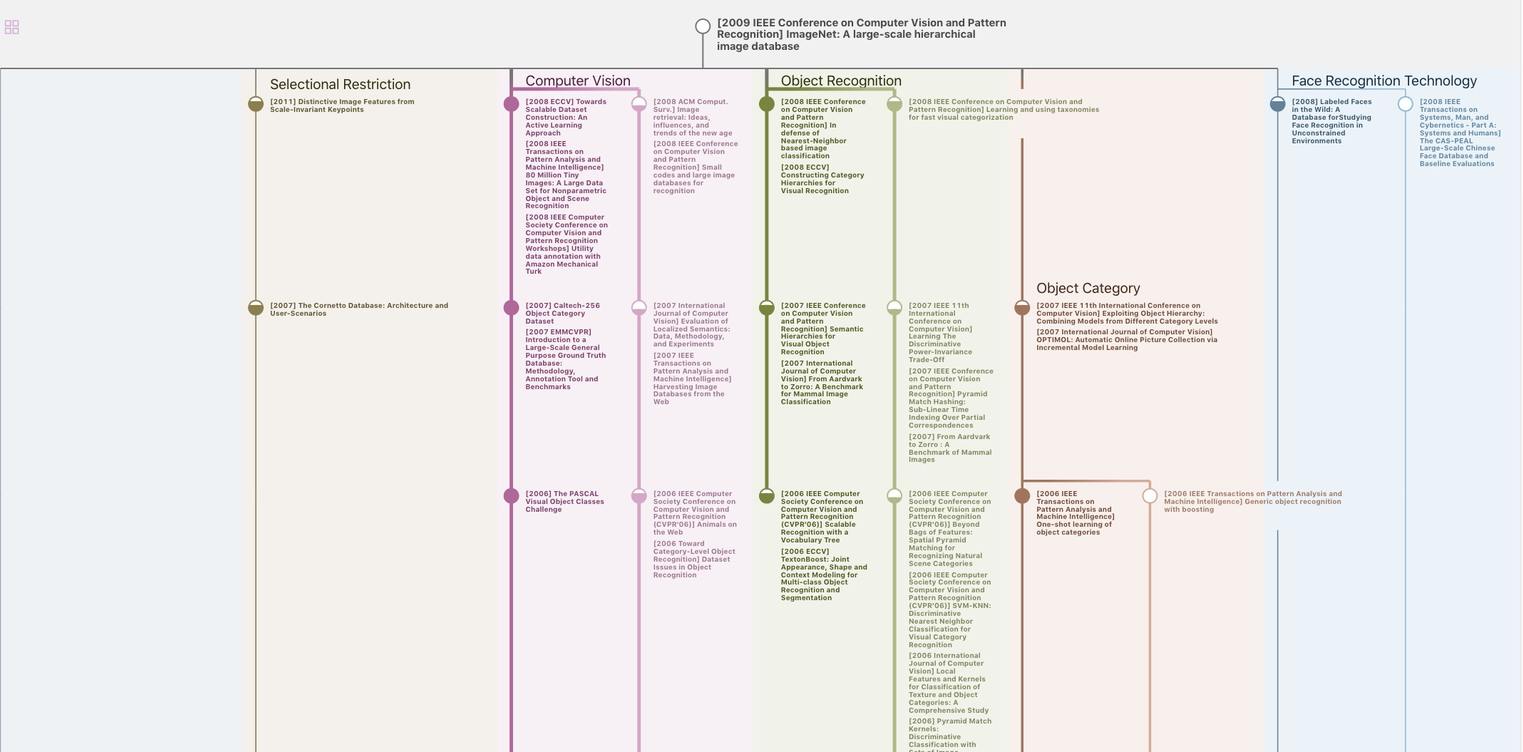
生成溯源树,研究论文发展脉络
Chat Paper
正在生成论文摘要