A Deep Learning Based Surrogate Model for Reservoir Dynamic Performance Prediction
GEOENERGY SCIENCE AND ENGINEERING(2024)
摘要
Predicting the reservoir dynamic performance is an important basis for exploiting the remaining oil and improving the recovery. Traditional prediction techniques primarily include numerical simulation and reservoir engineering methods, which show technical drawbacks such as low computational efficiency and too simplified assumptions. To tackle this challenge, we propose a surrogate model for reservoir dynamic performance prediction based on a Variety of View deep convolutional encoder-decoder (VoV-DCED) network. The influence of production time is accounted for using dimension expansion. Our model directly predicts the remaining oil saturation distribution with known reservoir permeability maps and varying well schedules. We examine the performance of our model in 2D and 3D waterflooding reservoirs, which shows that the prediction results are in good consistence with the reservoir numerical simulation. The model can be extended to account for the impacts of other factors, such as initial oil saturation distribution, well patterns, and non-frequent heterogeneities. Although deep learning takes a longer time for dataset preparation in comparison to the traditional simulation, the prediction efficiency of our trained model is almost two orders of magnitude higher than that of numerical simulation. Therefore, this surrogate model can act as an efficient tool for automatic history matching and production optimization—which require multiple simulation runs.
更多查看译文
关键词
Deep learning,Performance prediction,Encoder-decoder network,Surrogate model,Well schedule,Water-flooding
AI 理解论文
溯源树
样例
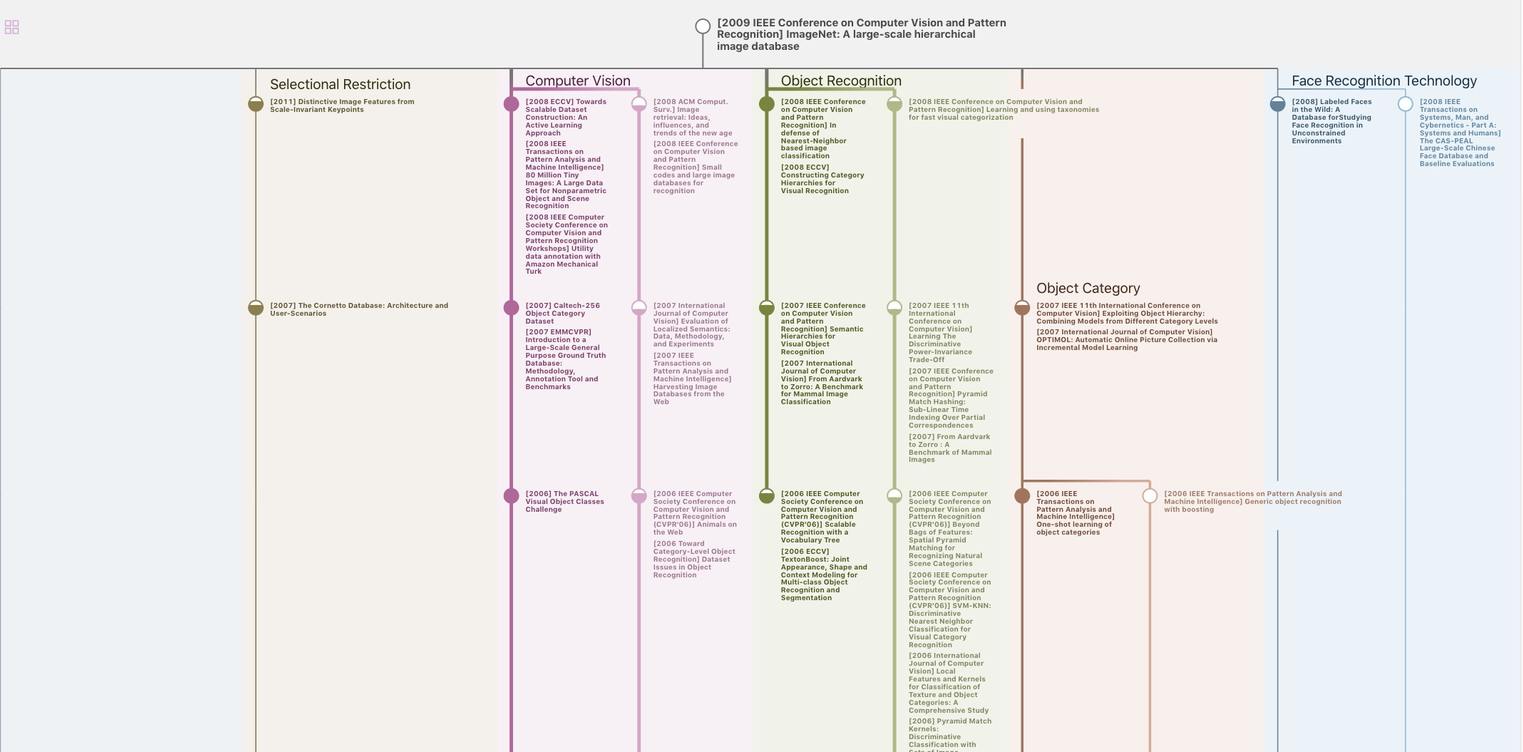
生成溯源树,研究论文发展脉络
Chat Paper
正在生成论文摘要